Neutral Class Handling for Customer Sentiment Analysis In Binary Classification: A Comparative Study of Supervised Machine Learning Classification Algorithm
2023 Eighth International Conference on Informatics and Computing (ICIC)(2023)
摘要
Customer sentiment analyses improve customer experience and ultimately drive business success. Polarity classification is considered the standard research task in sentiment analysis. This study’s primary focus is conducting a comparative study with 10-fold cross-validation to discover the most effective sentiment classifier to handle neutral sentiment in order to enhance sentiment polarity categorization. This study employs binary classification utilizing supervised machine learning methods such as Naïve Bayes and Support Vector Machine to classify neutral sentiment or a 3-star rating in online customer reviews. Results show that SVM outperforms Naïve Bayes in classifying sentiment with an AUC value of 0,923 for scenarios the neutral class considered removed from the data set. The comparison result based on three scenarios to handle the neutral class indicates that the neutral class may be considered a positive class with an AUC value of 0.904 based on the supervised machine-learning approach. A review with a 3-star rating is seen as positive feedback and slightly better than average or meets expectations. The neutral sentiment was considered an acceptable score of satisfied with some positive aspects. Further investigation is required to multiclass approaches to classify the customer sentiments as positive, negative, or neutral to achieve better accuracy and prevent the risk of misclassification.
更多查看译文
关键词
customer sentiment analysis,neutral class,binary classification,supervised machine learning
AI 理解论文
溯源树
样例
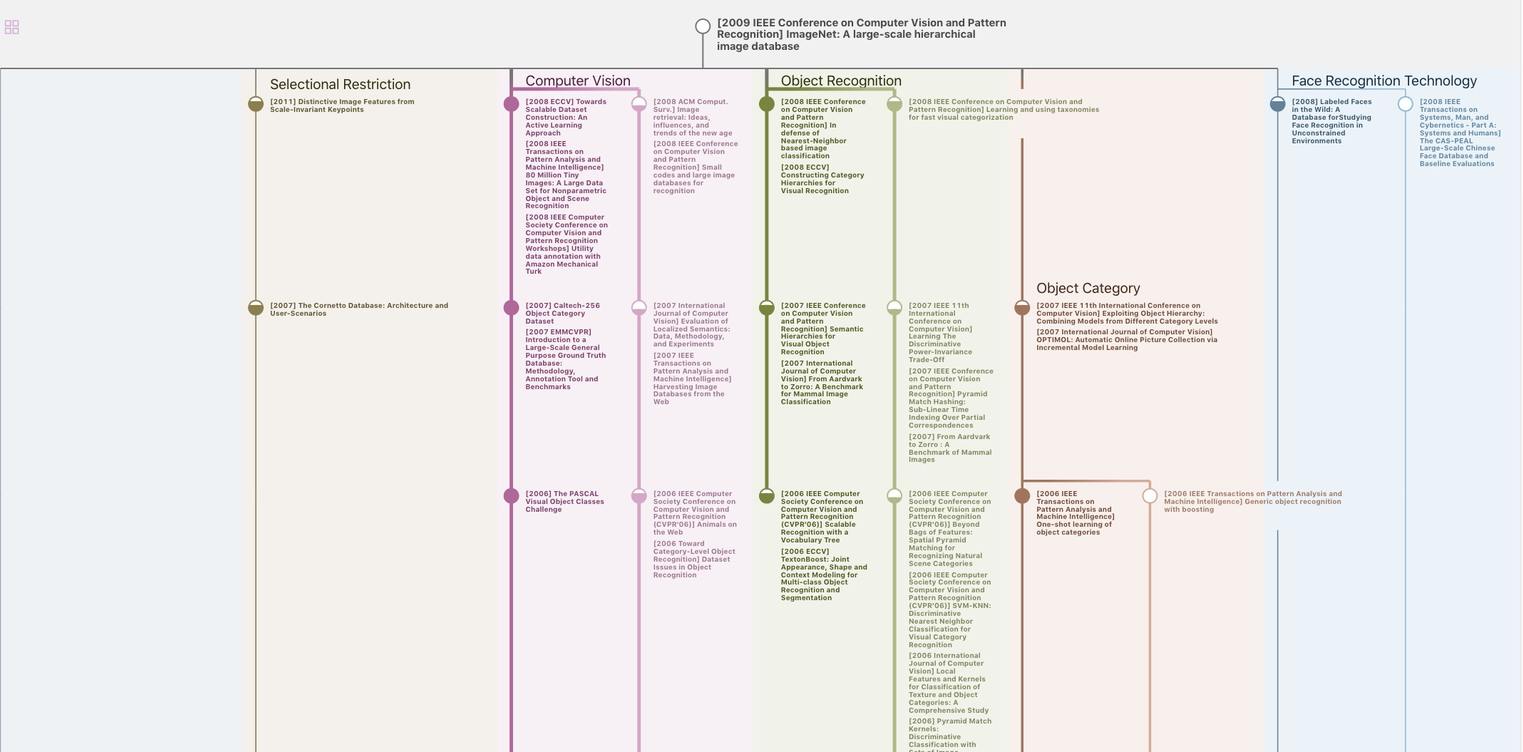
生成溯源树,研究论文发展脉络
Chat Paper
正在生成论文摘要