Credit Card Fraud Detection: Addressing Imbalanced Datasets with a Multi-phase Approach
SN Computer Science(2024)
摘要
Credit card fraud detection plays a crucial role in safeguarding the financial security of individuals and organizations. However, imbalanced datasets pose significant challenges to accurately identifying fraudulent transactions. In this research paper, we propose a novel approach that combines autoencoder (AE) and fully connected deep networks (FCDN) models to address this issue. The process involves three phases: training an AE on fraudulent transactions, utilizing another AE for dimensionality reduction, and using the encoded representations as input for FCDN classification. To further enhance the model’s performance, we introduce an additional FCDN trained on the preprocessed data using the synthetic minority oversampling technique (SMOTE). The predictions from both AE, AE–FCDN, and the FCDN are combined using a majority voting approach. We evaluate the proposed method using standard performance metrics, including accuracy, precision, recall, and F1-score. Our experimental results demonstrate the effectiveness and robustness of the integrated model architecture in accurately detecting credit card fraud. These findings provide valuable insights for improving financial security measures and mitigating potential losses associated with credit card fraud.
更多查看译文
关键词
AE,Reconstruction error,Dimensionality reduction,FCDN,SMOTE,Majority voting,Credit card fraud detection
AI 理解论文
溯源树
样例
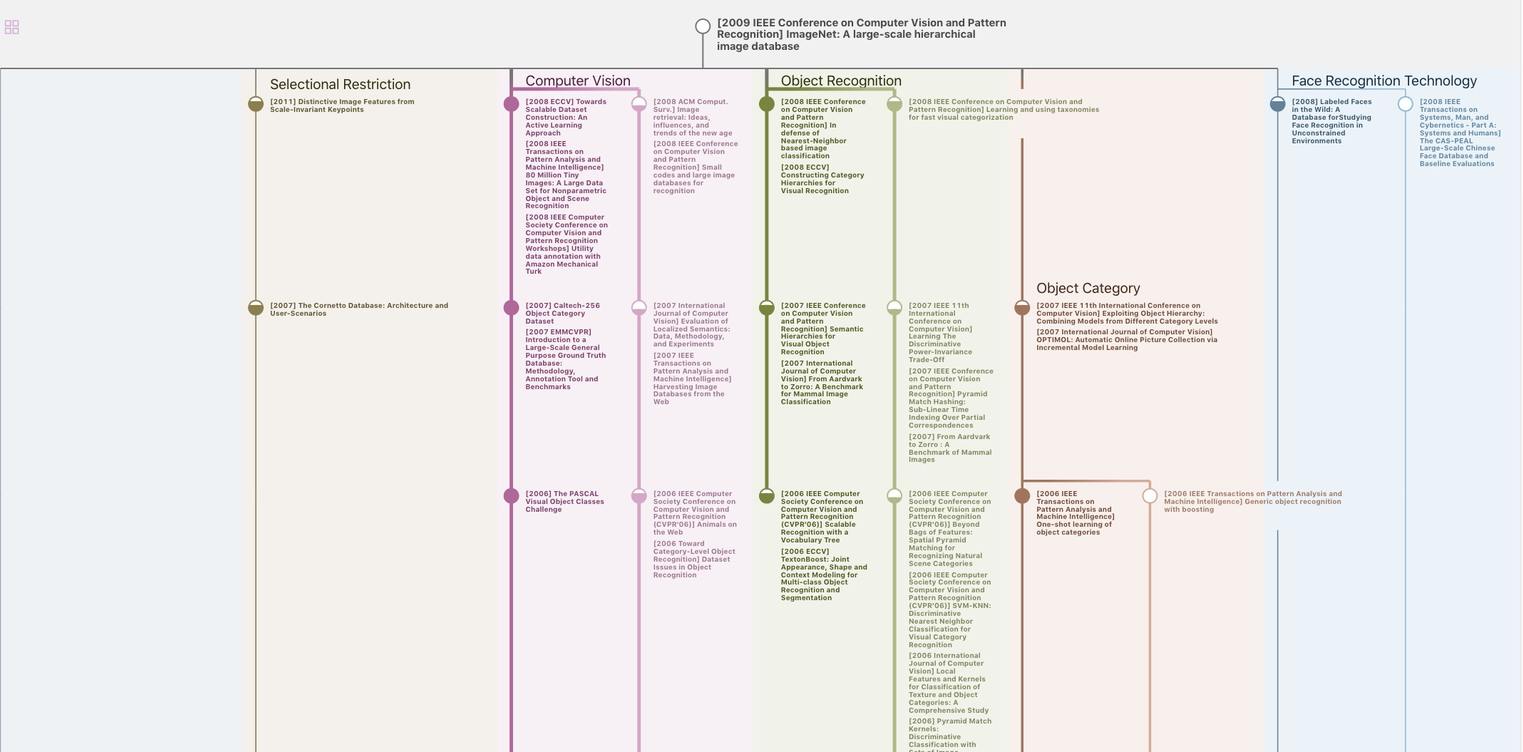
生成溯源树,研究论文发展脉络
Chat Paper
正在生成论文摘要