DTR-GAN: An Unsupervised Bidirectional Translation Generative Adversarial Network for MRI-CT Registration
APPLIED SCIENCES-BASEL(2024)
摘要
Medical image registration is a fundamental and indispensable element in medical image analysis, which can establish spatial consistency among corresponding anatomical structures across various medical images. Since images with different modalities exhibit different features, it remains a challenge to find their exact correspondence. Most of the current methods based on image-to-image translation cannot fully leverage the available information, which will affect the subsequent registration performance. To solve the problem, we develop an unsupervised multimodal image registration method named DTR-GAN. Firstly, we design a multimodal registration framework via a bidirectional translation network to transform the multimodal image registration into a unimodal registration, which can effectively use the complementary information of different modalities. Then, to enhance the quality of the transformed images in the translation network, we design a multiscale encoder-decoder network that effectively captures both local and global features in images. Finally, we propose a mixed similarity loss to encourage the warped image to be closer to the target image in deep features. We extensively evaluate methods for MRI-CT image registration tasks of the abdominal cavity with advanced unsupervised multimodal image registration approaches. The results indicate that DTR-GAN obtains a competitive performance compared to other methods in MRI-CT registration. Compared with DFR, DTR-GAN has not only obtained performance improvements of 2.35% and 2.08% in the dice similarity coefficient (DSC) of MRI-CT registration and CT-MRI registration on the Learn2Reg dataset but has also decreased the average symmetric surface distance (ASD) by 0.33 mm and 0.12 mm on the Learn2Reg dataset.
更多查看译文
关键词
multimodal image registration,image-to-image translation,unsupervised,deep learning
AI 理解论文
溯源树
样例
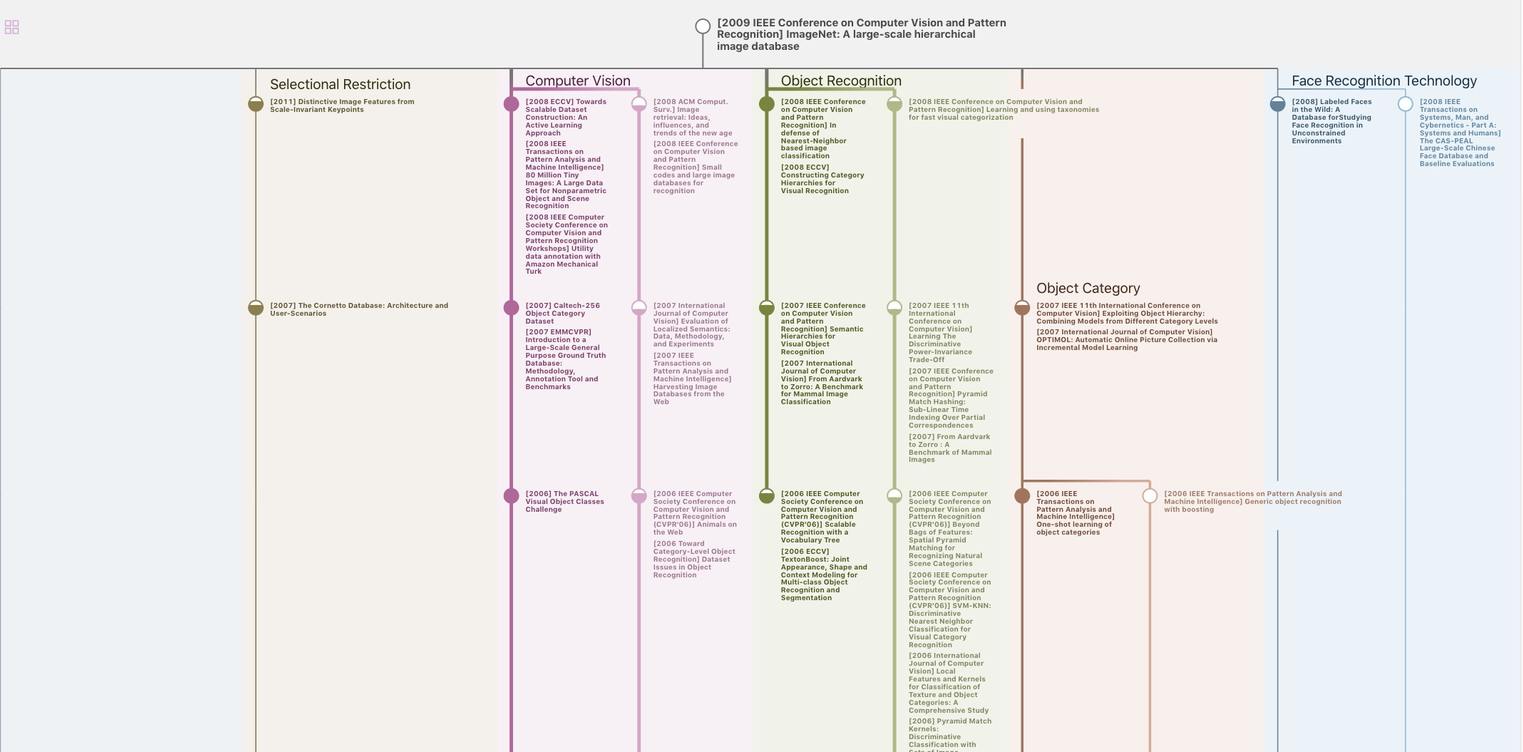
生成溯源树,研究论文发展脉络
Chat Paper
正在生成论文摘要