Integration of Computational Docking into Anti-Cancer Drug Response Prediction Models
CANCERS(2024)
摘要
Simple Summary Anti-cancer drug response prediction models aim to reduce the time necessary for developing a treatment for patients affected by this complex disease. Their goal is to decrease the number of required biological experiments by computationally weeding out unpromising compounds. In this work, we explore the potential gains of incorporating large-scale applications of classical virtual screening techniques like molecular docking into cutting-edge deep learning models. We demonstrate improvement in performance as well as limitations of our approach.Abstract Cancer is a heterogeneous disease in that tumors of the same histology type can respond differently to a treatment. Anti-cancer drug response prediction is of paramount importance for both drug development and patient treatment design. Although various computational methods and data have been used to develop drug response prediction models, it remains a challenging problem due to the complexities of cancer mechanisms and cancer-drug interactions. To better characterize the interaction between cancer and drugs, we investigate the feasibility of integrating computationally derived features of molecular mechanisms of action into prediction models. Specifically, we add docking scores of drug molecules and target proteins in combination with cancer gene expressions and molecular drug descriptors for building response models. The results demonstrate a marginal improvement in drug response prediction performance when adding docking scores as additional features, through tests on large drug screening data. We discuss the limitations of the current approach and provide the research community with a baseline dataset of the large-scale computational docking for anti-cancer drugs.
更多查看译文
关键词
anti-cancer drug response prediction,machine learning,deep learning,binding affinity,computational docking,molecular mechanisms of action
AI 理解论文
溯源树
样例
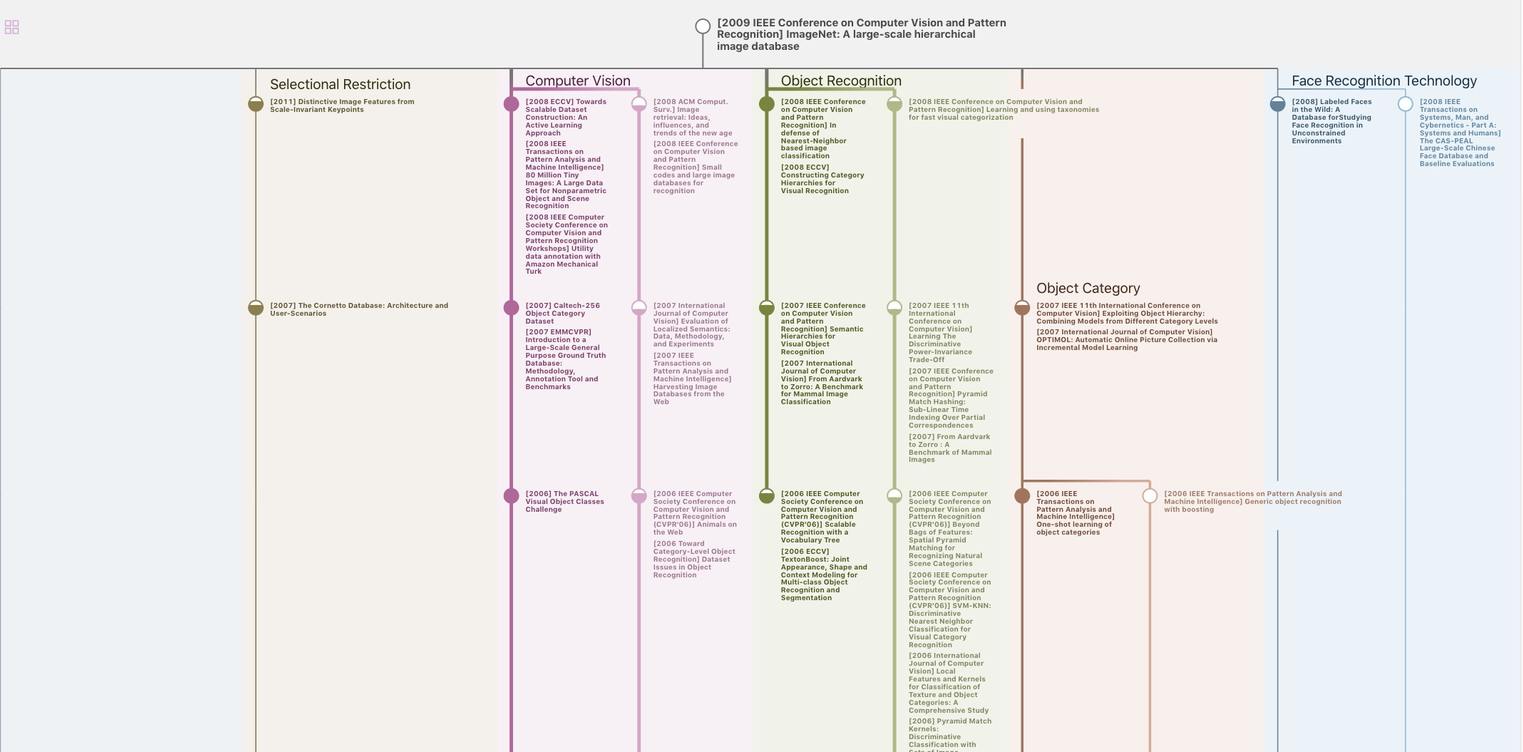
生成溯源树,研究论文发展脉络
Chat Paper
正在生成论文摘要