Probabilistic Load Flow Analysis Using Nonparametric Distribution
Sustainability(2023)
摘要
In the pursuit of sustainable energy solutions, this research addresses the critical need for accurate probabilistic load flow (PLF) analysis in power systems. PLF analysis is an essential tool for estimating the statistical behavior of power systems under uncertainty. It plays a vital part in power system planning, operation, and dependability studies. To perform accurate PLF analysis, this article proposes a Kernel density estimation with adaptive bandwidth for probability density function (PDF) estimation of power injections from sustainable energy sources like solar and wind, reducing errors in PDF estimation. To reduce the computational burden, a Latin hypercube sampling approach was incorporated. Input random variables are modeled using kernel density estimation (KDE) in conjunction with Latin hypercube sampling (LHS) for probabilistic load flow (PLF) analysis. To test the proposed techniques, IEEE 14 and IEEE 118 bus systems are used. Two benchmark techniques, the Monte Carlo Simulation (MCS) method and Hamiltonian Monte Carlo (HMC), were set side by side for validation of results. The results illustrate that an adaptive bandwidth kernel density estimation with the Latin hypercube sampling (AKDE-LHS) method provides better performance in terms of precision and computational efficiency. The results also show that the suggested technique is more feasible in reducing errors, uncertainties, and computational time while depicting arbitrary distributions of photovoltaic and wind farms for probabilistic load flow analysis. It can be a potential solution to tackle challenges posed by sustainable energy sources in power systems.
更多查看译文
关键词
adaptive kernel density,probabilistic load flow,Latin hypercube sampling,HMC,Monte Carlo
AI 理解论文
溯源树
样例
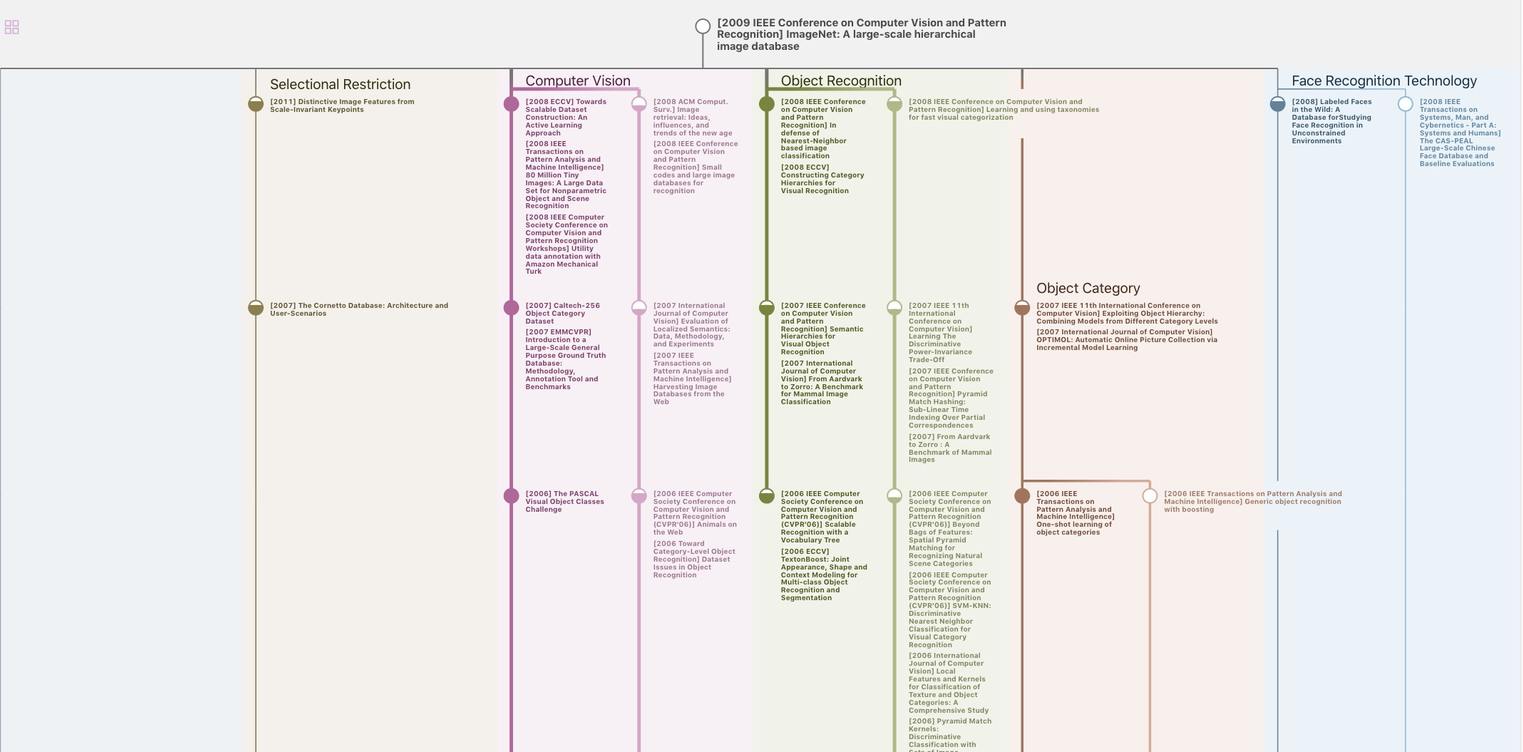
生成溯源树,研究论文发展脉络
Chat Paper
正在生成论文摘要