Multi-Sensor Fusion Simultaneous Localization Mapping Based on Deep Reinforcement Learning and Multi-Model Adaptive Estimation
SENSORS(2024)
摘要
In this study, we designed a multi-sensor fusion technique based on deep reinforcement learning (DRL) mechanisms and multi-model adaptive estimation (MMAE) for simultaneous localization and mapping (SLAM). The LiDAR-based point-to-line iterative closest point (PLICP) and RGB-D camera-based ORBSLAM2 methods were utilized to estimate the localization of mobile robots. The residual value anomaly detection was combined with the Proximal Policy Optimization (PPO)-based DRL model to accomplish the optimal adjustment of weights among different localization algorithms. Two kinds of indoor simulation environments were established by using the Gazebo simulator to validate the multi-model adaptive estimation localization performance, which is used in this paper. The experimental results of the proposed method in this study confirmed that it can effectively fuse the localization information from multiple sensors and enable mobile robots to obtain higher localization accuracy than the traditional PLICP and ORBSLAM2. It was also found that the proposed method increases the localization stability of mobile robots in complex environments.
更多查看译文
关键词
simultaneous localization and mapping (SLAM),deep reinforcement learning (DRL),multi-model adaptive estimation (MMAE),sensor fusion
AI 理解论文
溯源树
样例
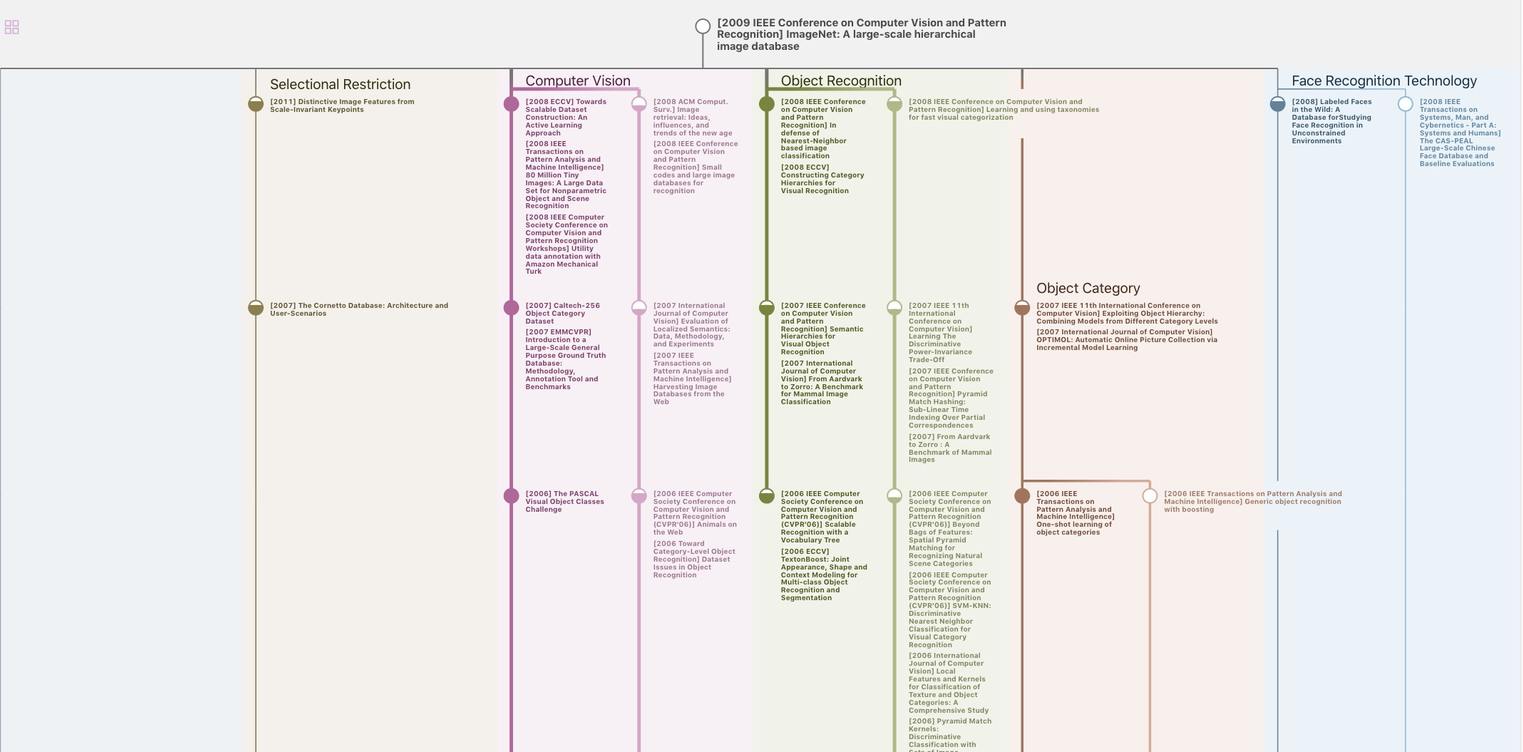
生成溯源树,研究论文发展脉络
Chat Paper
正在生成论文摘要