A Machine Learning Approach for Modelling Cold-Rolling Curves for Various Stainless Steels
MATERIALS(2024)
摘要
Stainless steel is a cold-work-hardened material. The degree and mechanism of hardening depend on the grade and family of the steel. This characteristic has a direct effect on the mechanical behaviour of stainless steel when it is cold-formed. Since cold rolling is one of the most widespread processes for manufacturing flat stainless steel products, the prediction of their strain-hardening mechanical properties is of great importance to materials engineering. This work uses artificial neural networks (ANNs) to forecast the mechanical properties of the stainless steel as a function of the chemical composition and the applied cold thickness reduction. Multiple linear regression (MLR) is also used as a benchmark model. To achieve this, both traditional and new-generation austenitic, ferritic, and duplex stainless steel sheets are cold-rolled at a laboratory scale with different thickness reductions after the industrial intermediate annealing stage. Subsequently, the mechanical properties of the cold-rolled sheets are determined by tensile tests, and the experimental cold-rolling curves are drawn based on those results. A database is created from these curves to generate a model applying machine learning techniques to predict the values of the tensile strength (Rm), yield strength (Rp), hardness (H), and elongation (A) based on the chemical composition and the applied cold thickness reduction. These models can be used as supporting tools for designing and developing new stainless steel grades and/or adjusting cold-forming processes.
更多查看译文
关键词
stainless steel,strain hardening,cold-rolling curves,machine learning,intelligent modelling,artificial neural networks
AI 理解论文
溯源树
样例
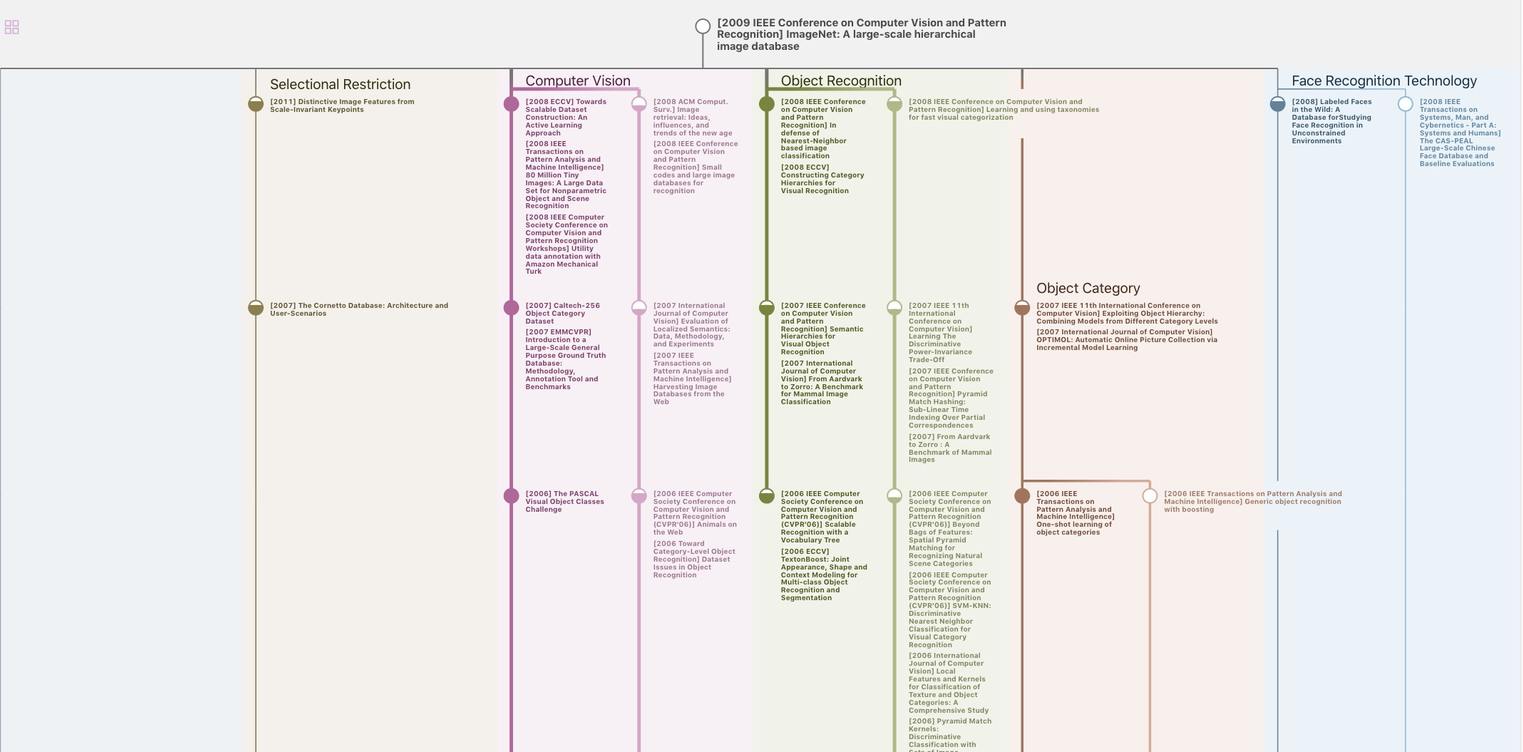
生成溯源树,研究论文发展脉络
Chat Paper
正在生成论文摘要