Detection of Hydrogen Bubbles Produced by Corrosion Inhibition of Metal Weldment Using Machine Learning
APPLIED SCIENCES-BASEL(2024)
摘要
This study analyzes the corrosion inhibition efficiency of sodium molybdate (SM) solution on weldment specimens in 1 M HCl, based on H2 bubbles detection. The detection of the bubbles produced by the corrosion process is achieved by a YOLOv4 deep learning algorithm. The results indicate that the corrosion rate is higher on the weld metal zone than on the base metal zone in the same solution, which might be attributed to the coarser grain of the weld metal zone and the stability of the produced oxide layer. The addition of sodium molybdate was discovered to boost the stability of the oxide layer, hence enhancing the specimens' corrosion resistance. The overall inhibitory efficiency of the sodium molybdate solution was 59% for the weld metal (WM) (0.4 g/L), 52% for the heat-affected zone (HAZ) (0.2 g/L), and 37% for the base metal (BM) (0.2 g/L). The object detection algorithm models showed 97% for the mAP and 0.98 for recall. The minimum average bubble detected for the WM was 0.353 /mm2 at an SM concentration of 0.4 g/L, while the HAZ was 0.612 /mm2 at 0.2 g/L, and the BM was 1.055 /mm2 at 0.2 g/L. The results of the bubbles detection appeared to be consistent with the corrosion experiment outcomes obtained by the potentiodynamic polarization and hydrogen volume measurement tests. This experiment validates the distinctiveness of the different weld zones in terms of the inhibitor concentration required for successful corrosion prevention, as well as the potential of analyzing corrosion using machine learning algorithms for object detection.
更多查看译文
关键词
deep learning,hydrogen evolution,carbon steel welding,inhibitor,corrosion
AI 理解论文
溯源树
样例
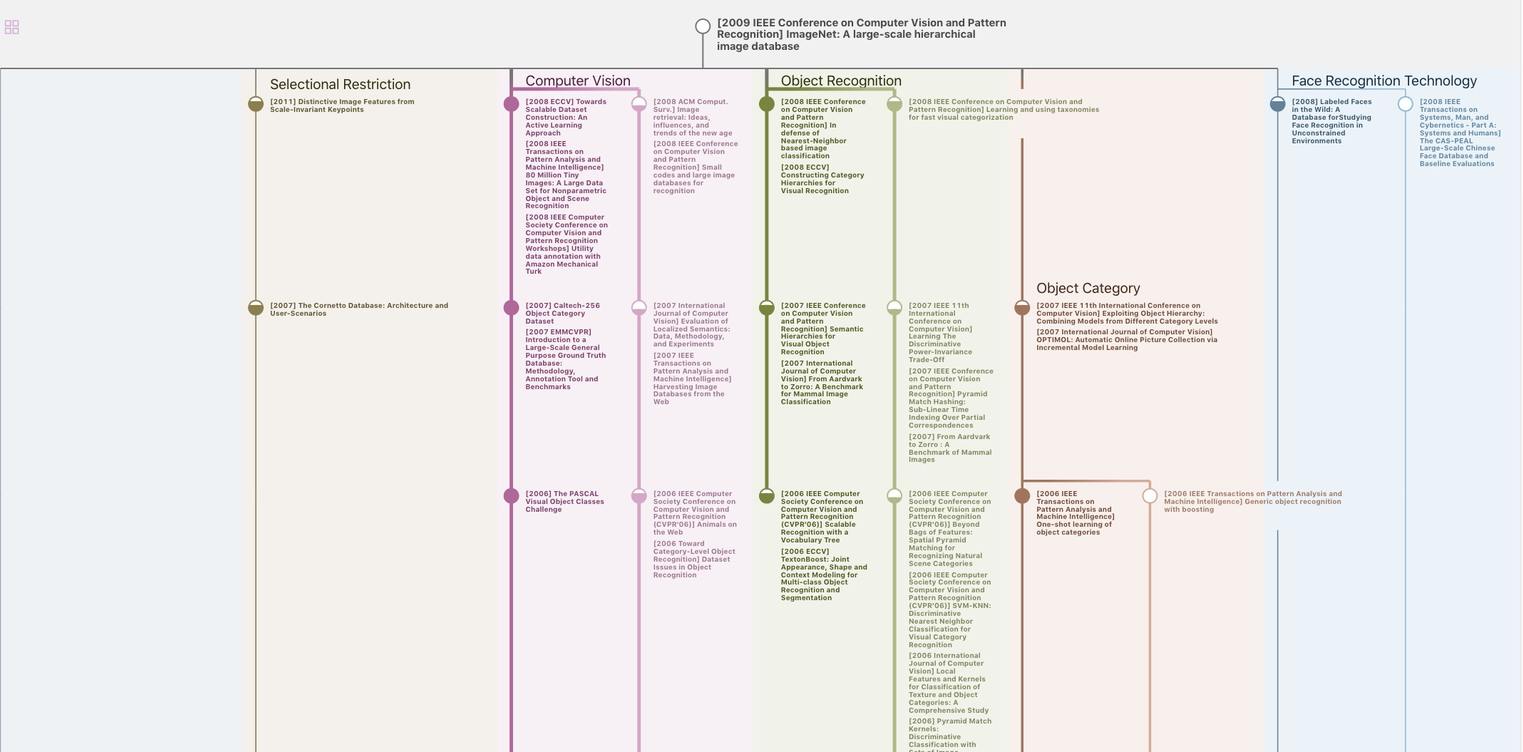
生成溯源树,研究论文发展脉络
Chat Paper
正在生成论文摘要