DYMatch: Semi-Supervised Learning with Dynamic Pseudo Labeling and Feature Consistency
APPLIED SCIENCES-BASEL(2024)
摘要
A considerable number of approaches based on consistency regularization and pseudo-labeling have been proposed in Semi-supervised Learning (SSL) so far. These approaches significantly enhance the SSL methods by effectively utilizing large amount of unlabeled data to improve the model's performance. However, existing methods may fail to utilize the unlabeled data more efficiently, mainly due to the challenges faced in pseudo-label estimation. In this paper, we begin by analyzing the impact of the pseudo-label estimation method on training the SSL model. Furthermore, we emphasize that an effective pseudo-label estimation method should reflect the difference of recognition performance among samples from different categories, and also ensure the maintenance of both high-quantity and high-quality pseudo-labels during each training iteration. Based on above analysis, we propose DYMatch, an innovative SSL method employing a dynamic estimation process. Firstly, a dynamic pseudo-label estimation method based on Gaussian mixture model is proposed to dynamically estimate the confidence threshold on different categories. Secondly, a feature-correlation consistency regularization method is introduced to further enhance the learning on unlabeled data. The experimental results show that DYMatch is a simple and effective SSL method, especially when the labeled data is limited.
更多查看译文
关键词
semi-supervised learning,dynamic pseudo-label estimation,Gaussian mixture model,feature-correlation consistency regularization
AI 理解论文
溯源树
样例
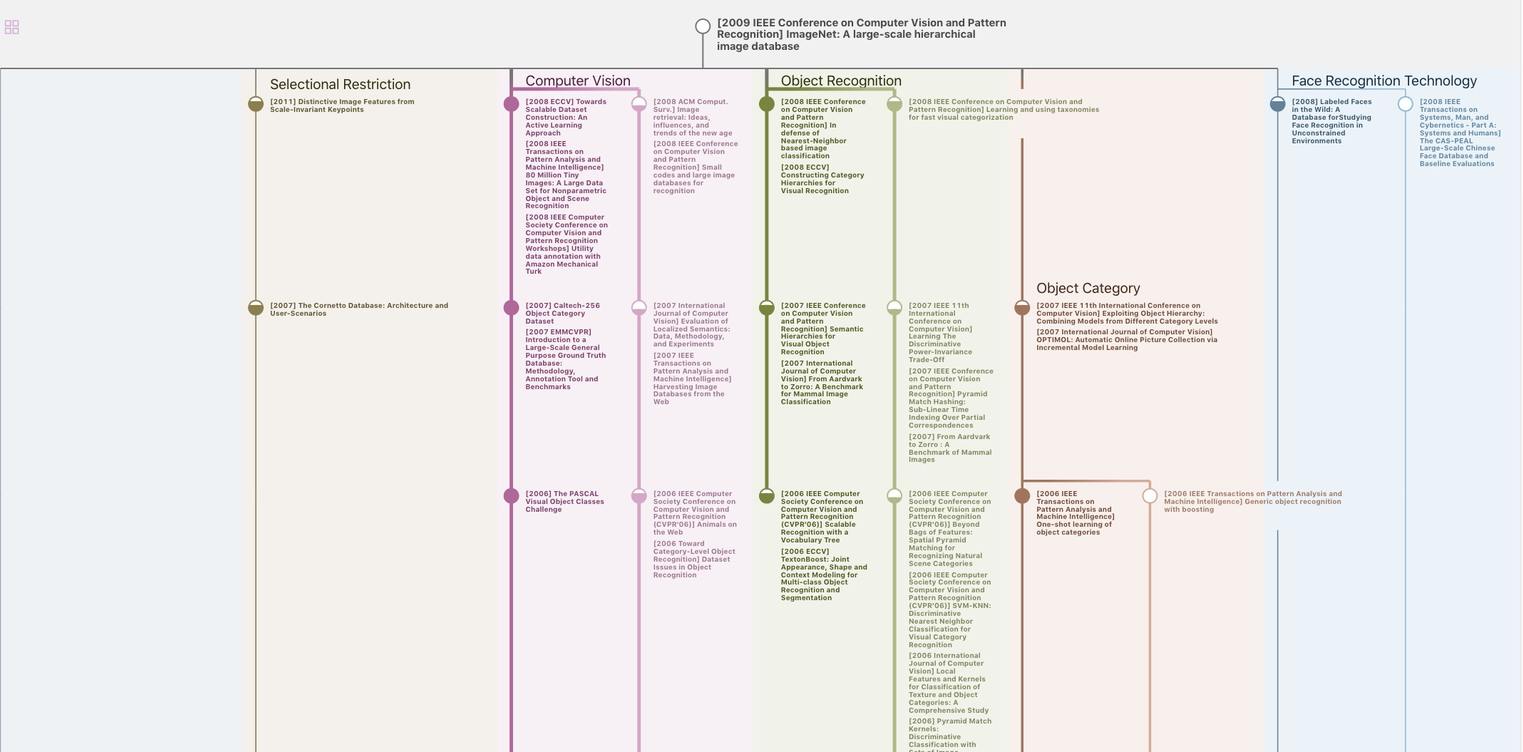
生成溯源树,研究论文发展脉络
Chat Paper
正在生成论文摘要