GSA-KELM-KF: A Hybrid Model for Short-Term Traffic Flow Forecasting
MATHEMATICS(2024)
摘要
Short-term traffic flow forecasting, an essential enabler for intelligent transportation systems, is a fundamental and challenging task for dramatically changing traffic flow over time. In this paper, we present a gravitational search optimized kernel extreme learning machine, named GSA-KELM, to avoid manually traversing all possible parameters to improve the potential performance. Furthermore, with the interference of heavy-tailed impulse noise, the performance of KELM may be seriously deteriorated. Based on the Kalman filter that cleverly combines observed data and estimated data to perform the closed-loop management of errors and limit the errors within a certain range, we propose a combined model, termed GSA-KELM-KF. The experimental results of two real-world datasets demonstrate that GSA-KELM-KF outperforms the state-of-the-art parametric and non-parametric models.
更多查看译文
关键词
traffic flow theory,extreme learning machine,Kalman filter
AI 理解论文
溯源树
样例
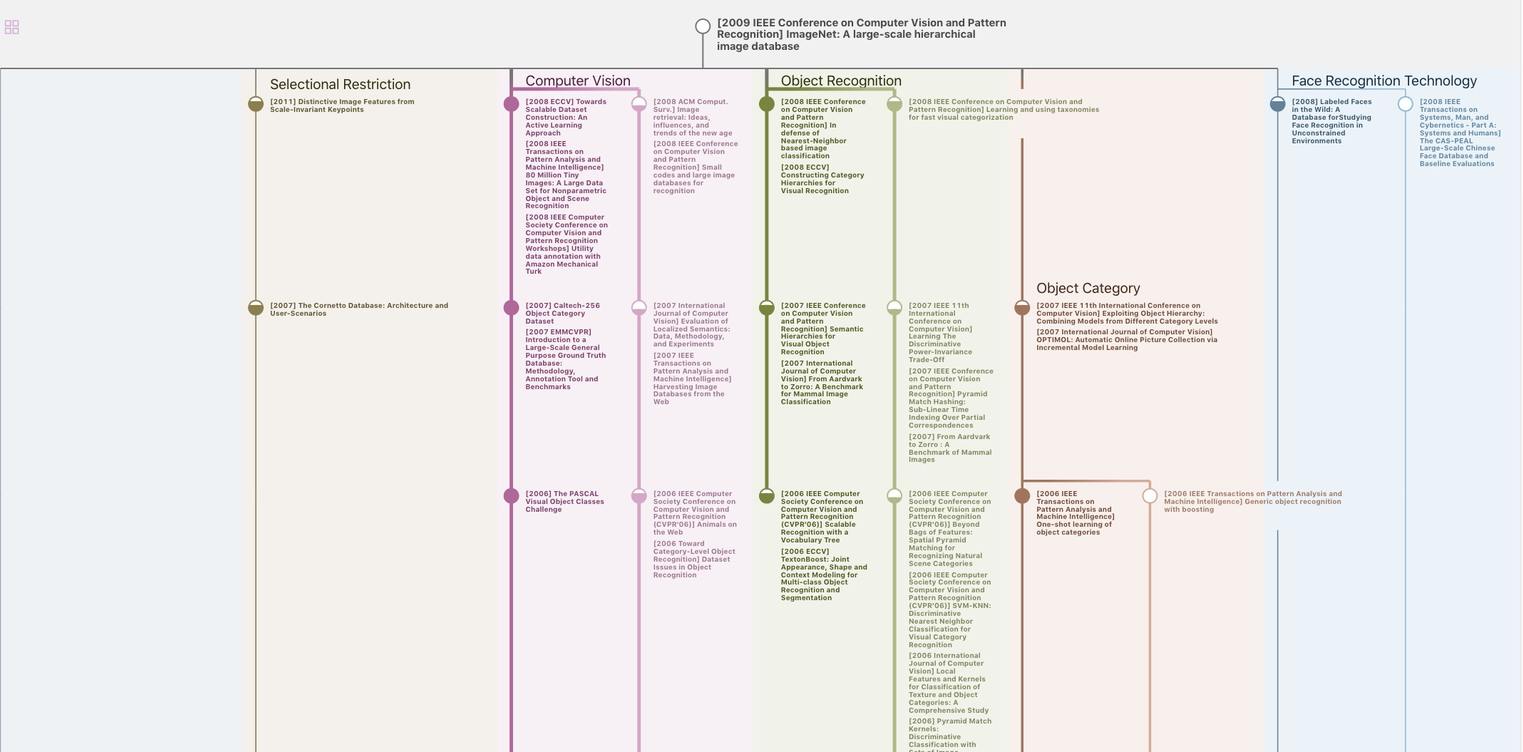
生成溯源树,研究论文发展脉络
Chat Paper
正在生成论文摘要