Nonlinear preconditioned primal-dual method for a class of structured minimax problems
CoRR(2024)
摘要
We propose and analyze a general framework called nonlinear preconditioned
primal-dual with projection for solving nonconvex-nonconcave and non-smooth
saddle-point problems. The framework consists of two steps. The first is a
nonlinear preconditioned map followed by a relaxed projection onto the
separating hyperspace we construct. One key to the method is the selection of
preconditioned operators, which tailors to the structure of the saddle-point
problem and is allowed to be nonlinear and asymmetric. The other is the
construction of separating hyperspace, which guarantees fast convergence. This
framework paves the way for constructing nonlinear preconditioned primal-dual
algorithms. We show that weak convergence, and so is sublinear convergence
under the assumption of the convexity of saddle-point problems and linear
convergence under a metric subregularity. We also show that many existing
primal-daul methods, such as the generalized primal-dual algorithm method, are
special cases of relaxed preconditioned primal-dual with projection.
更多查看译文
AI 理解论文
溯源树
样例
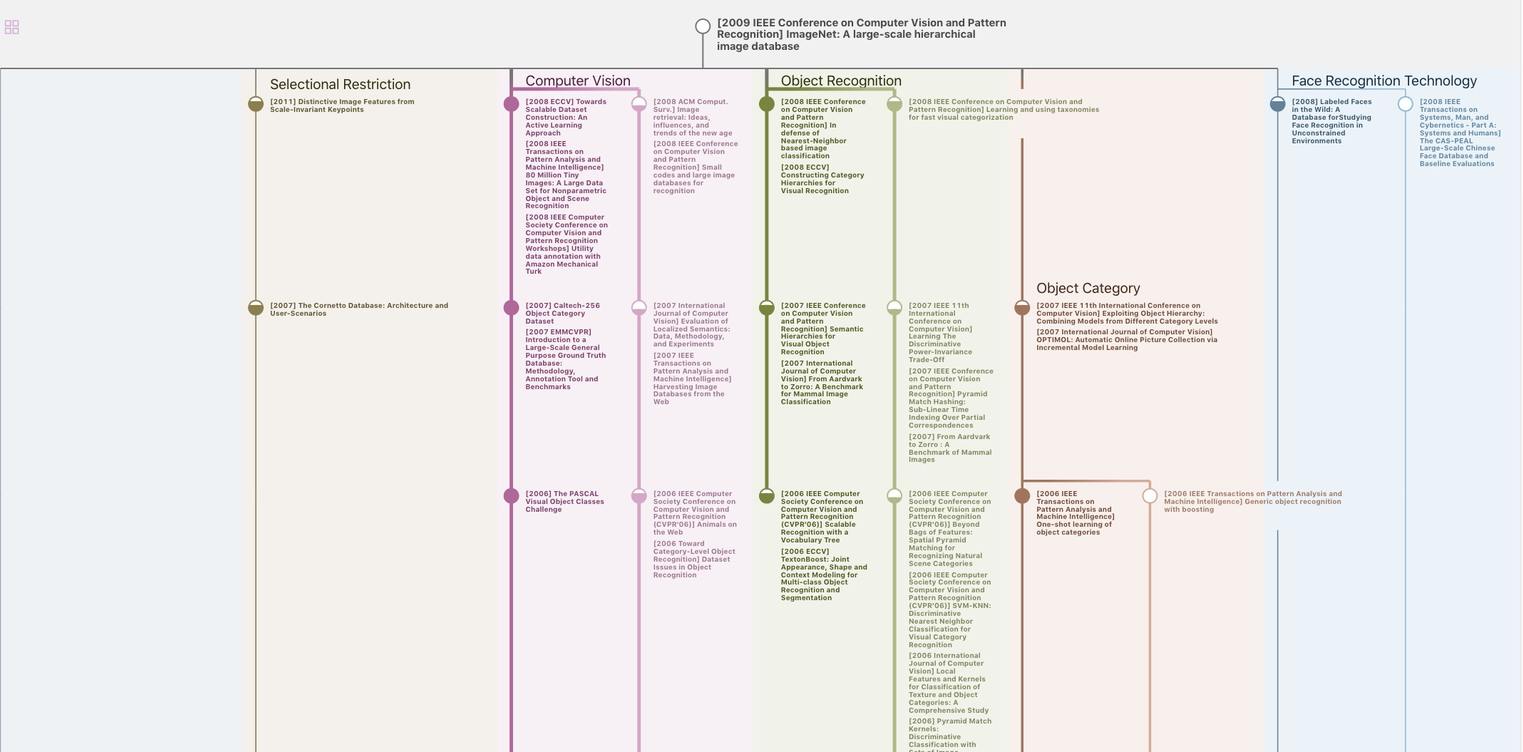
生成溯源树,研究论文发展脉络
Chat Paper
正在生成论文摘要