New deep recurrent hybrid artificial neural network for forecasting seasonal time series
Granular Computing(2024)
摘要
The simple recurrent artificial neural network is a deep artificial neural network frequently used in the literature for solving forecasting problems. It is preferred due to its low number of parameters. At the same time, the simple exponential smoothing method, which is one of the classical forecasting methods, is also used in the literature for solving forecasting problems. Both methods draw attention to their performance in solving forecasting problems. In this study, a hybrid approach is proposed in which these two methods, which perform very well in the forecasting problems of time series with seasonal components, are used together. The architecture of the network uses the forecasts during the seasonality period as recurrent. In the training of the proposed hybrid neural network, training algorithms based on particle swarm optimization algorithm and sine cosine optimization algorithms are proposed. The proposed training algorithms use a restart strategy to reduce the variability caused by random initial weights and to make the results more stable. In the recommended training algorithm, an early stopping condition is applied to solve the overfitting problem based on proportional error. The performance of this proposed method is analyzed using many seasonal time series and compared with both classical forecasting methods and deep and shallow artificial neural network methods.
更多查看译文
关键词
Simple recurrent artificial neural network,Exponential smoothing,Sine cosine optimization algorithm,Particle swarm optimization algorithm,Seasonal time series,Forecasting
AI 理解论文
溯源树
样例
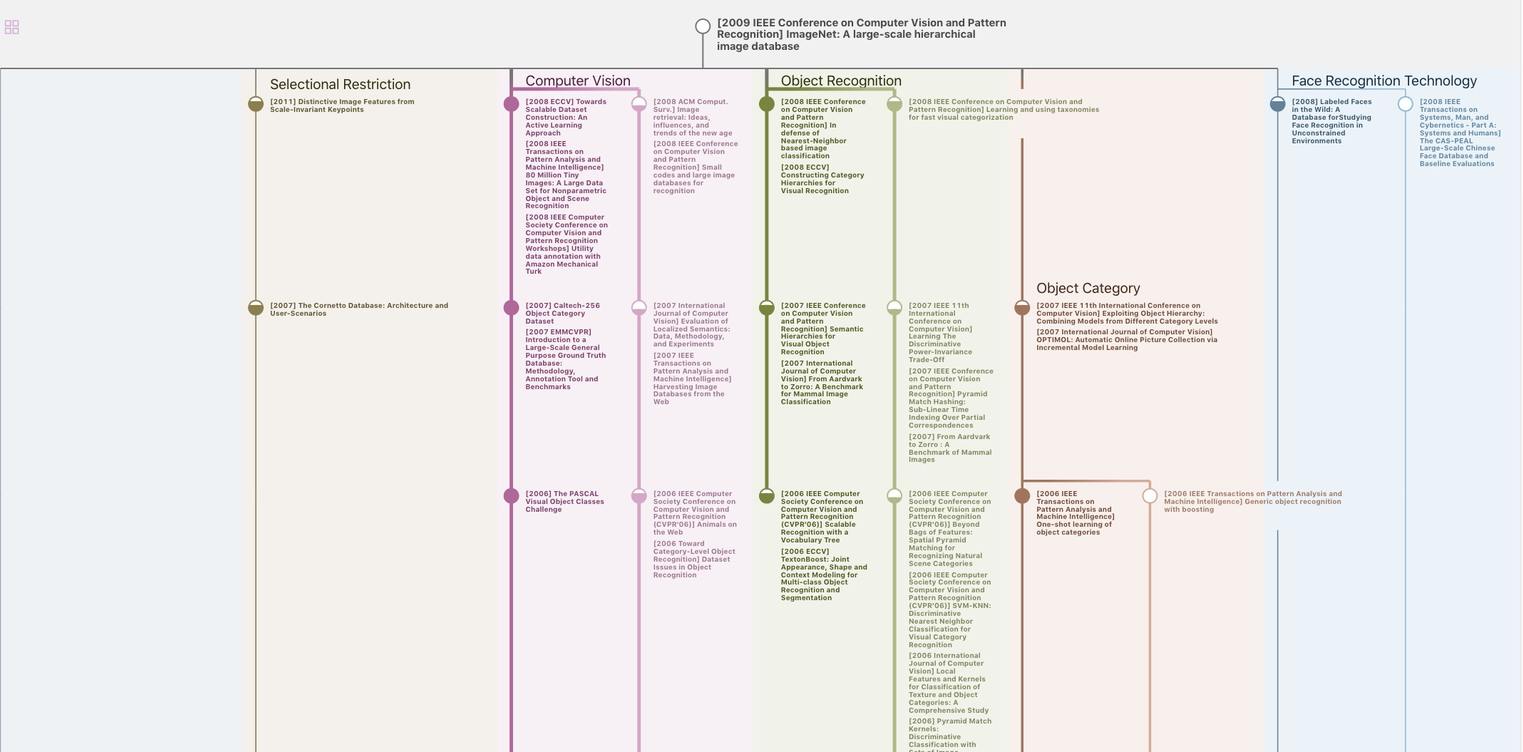
生成溯源树,研究论文发展脉络
Chat Paper
正在生成论文摘要