Orthogonality-based bias-corrected empirical likelihood inference for partial linear varying coefficient EV models with longitudinal data
JOURNAL OF COMPUTATIONAL AND APPLIED MATHEMATICS(2024)
摘要
In this paper, we study the model estimation for the partial linear varying coefficient errors -invariables (EV) models with longitudinal data. Based on the empirical likelihood and quadratic inference functions, we propose an orthogonality -based bias -corrected empirical likelihood estimation method using the QR decomposition method of matrix. The proposed method can handle the measurement errors of covariates and unknown within -subject correlation simultaneously, estimate the parametric and nonparametric components separately. Under some regularity conditions, the resulting estimator of the parametric component is asymptotic normal and the estimators of the nonparametric varying coefficients achieve the optimal convergence rate. Furthermore, we conduct simulation studies and a real data analysis to compare the performance of the proposed method with existing methods in finite samples. The results show that the feasibility of the proposed method is obvious.
更多查看译文
关键词
Longitudinal data,Partial linear varying coefficient EV models,Empirical likelihood,Quadratic inference functions
AI 理解论文
溯源树
样例
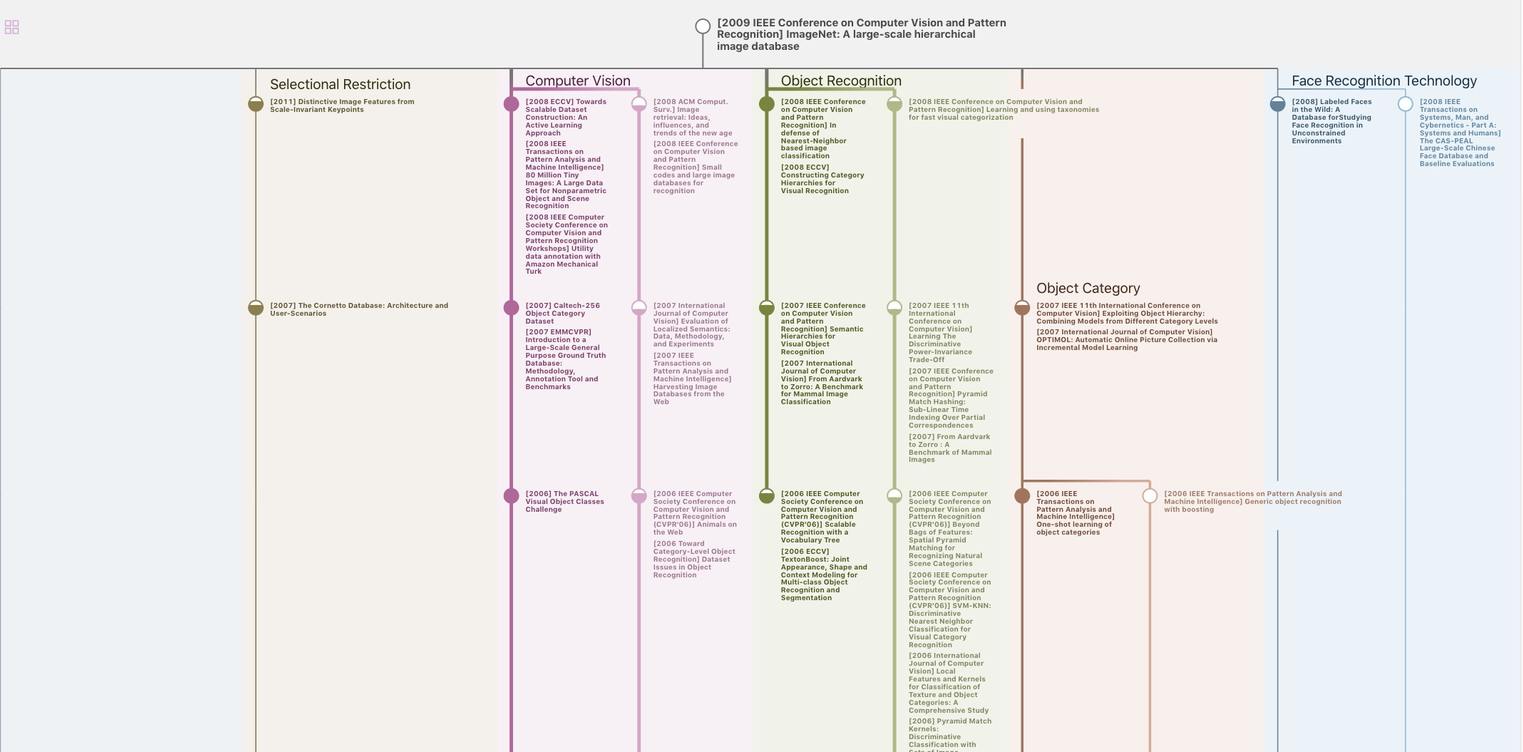
生成溯源树,研究论文发展脉络
Chat Paper
正在生成论文摘要