Multiple Time-Varying Formation Tracking of Multiple Heterogeneous Euler–Lagrange Systems Via Prescribed-Time Hierarchical Control Algorithms in Task Space
NONLINEAR DYNAMICS(2024)
Hubei Normal University | East China University of Science and Technology
Abstract
This paper aims at addressing the multiple time-varying formation tracking (MTVFT) problem for multiple heterogeneous Euler–Lagrange systems (MHELSs) in the scenario of uncertain dynamic terms and external disturbances within a prescribed time in the task space. A prescribed-time hierarchical control protocol is proposed to tackle above-mentioned problem. To be specific, a distributed prescribed-time estimator algorithm ensures that each agent can derive the accurate information of their corresponding leaders. In the local control layer, the prescribed-time controller is employed to verify MTVFT problem of the MHELSs, where the settling time is irrelevant to the initial conditions and control parameters of the system. With the help of the Lyapunov stability, the corresponding sufficient conditions for fulfilling the MTVFT in task space are derived. Eventually, the simulations results are performed to illustrate the feasibility and effectiveness of the main results.
MoreTranslated text
Key words
Multiple heterogeneous Euler–Lagrange systems (MHELSs),Multiple time-varying formation tracking (MTVFT),Prescribed-time hierarchical control (PTHC)
求助PDF
上传PDF
View via Publisher
AI Read Science
AI Summary
AI Summary is the key point extracted automatically understanding the full text of the paper, including the background, methods, results, conclusions, icons and other key content, so that you can get the outline of the paper at a glance.
Example
Background
Key content
Introduction
Methods
Results
Related work
Fund
Key content
- Pretraining has recently greatly promoted the development of natural language processing (NLP)
- We show that M6 outperforms the baselines in multimodal downstream tasks, and the large M6 with 10 parameters can reach a better performance
- We propose a method called M6 that is able to process information of multiple modalities and perform both single-modal and cross-modal understanding and generation
- The model is scaled to large model with 10 billion parameters with sophisticated deployment, and the 10 -parameter M6-large is the largest pretrained model in Chinese
- Experimental results show that our proposed M6 outperforms the baseline in a number of downstream tasks concerning both single modality and multiple modalities We will continue the pretraining of extremely large models by increasing data to explore the limit of its performance
Upload PDF to Generate Summary
Must-Reading Tree
Example
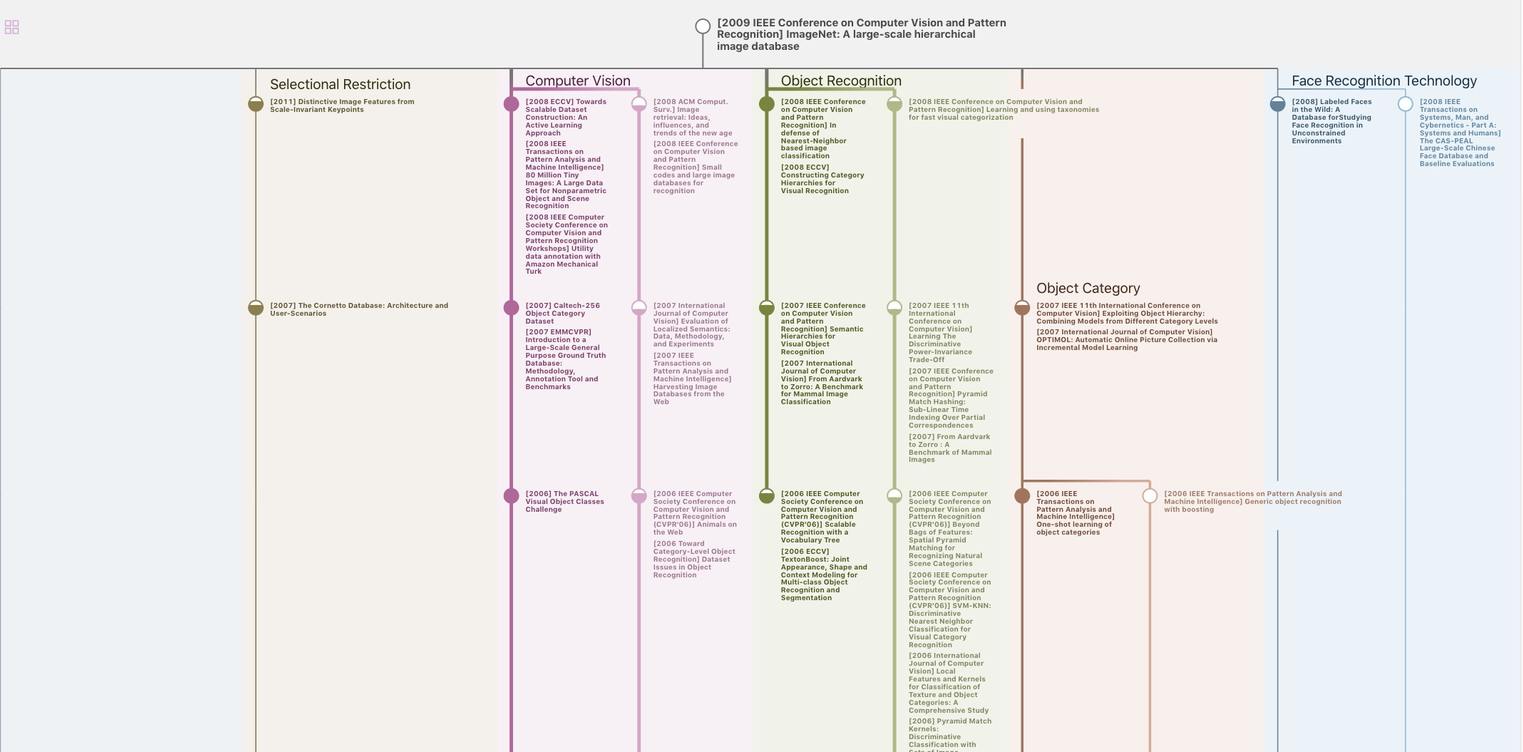
Generate MRT to find the research sequence of this paper
Related Papers
International Journal of Robust and Nonlinear Control 2024
被引用0
Data Disclaimer
The page data are from open Internet sources, cooperative publishers and automatic analysis results through AI technology. We do not make any commitments and guarantees for the validity, accuracy, correctness, reliability, completeness and timeliness of the page data. If you have any questions, please contact us by email: report@aminer.cn
Chat Paper
GPU is busy, summary generation fails
Rerequest