Building energy efficiency: using machine learning algorithms to accurately predict heating load
Asian Journal of Civil Engineering(2024)
摘要
The use of machine learning techniques to forecast heating load, a crucial component of building energy efficiency is examined in this work. Numerous building characteristics are found to correlate with heating load. Building height, wall area, surface area, roof area, and so forth are some examples of these. With an R-squared score of 0.921, a root mean square error (RMSE) of 2.87, and a mean absolute error (MAE) of 2.14, the models created for this study show high accuracy. With an astounding accuracy rate of 99.2%, the decision tree model excels among the tested algorithms. The exceptional performance of this model highlights its potential for useful applications in heating load prediction. Promising outcomes are also shown by other algorithms, such as logistic regression at 94.14%, K-nearest neighbors at 95.6%, and neural networks at 96.24%. The results of this study demonstrate how machine learning can be used to predict heating load, offering important information for the creation of energy-saving measures. We can contribute to sustainability efforts by designing more energy-efficient buildings by knowing the relationships between building features and heating load. This study is a major advancement in the use of machine learning for energy management in buildings.
更多查看译文
关键词
Heating load prediction,Energy optimization in buildings,Decision tree,KNN,Neural networks,Logistic regression
AI 理解论文
溯源树
样例
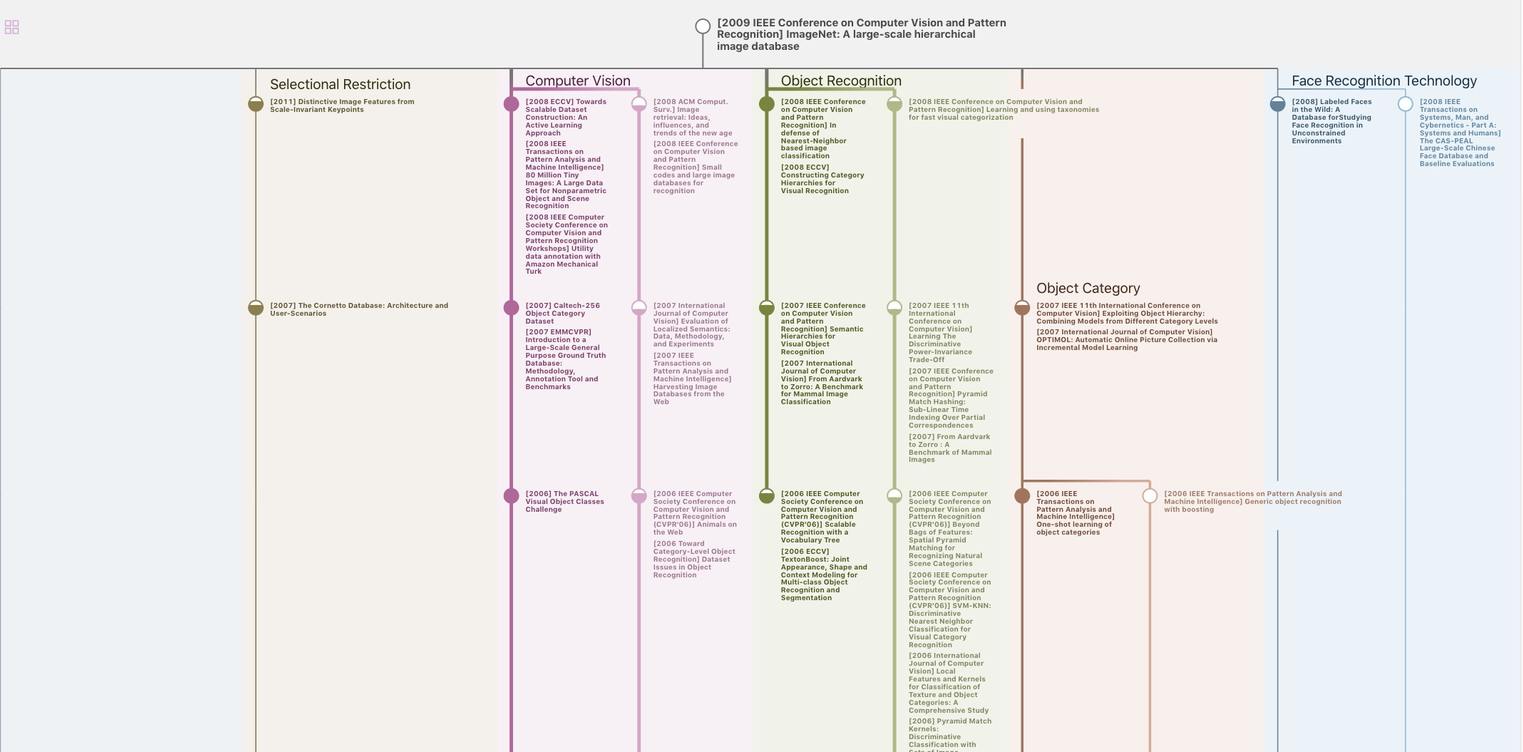
生成溯源树,研究论文发展脉络
Chat Paper
正在生成论文摘要