Prediction of purified water quality in industrial hydrocarbon wastewater treatment using an artificial neural network and response surface methodology
JOURNAL OF WATER PROCESS ENGINEERING(2024)
摘要
This study addresses the critical issue of determining the environmental impact of wastewater discharged from a petroleum complex's wastewater treatment plant (WWTP). The emphasis is on assessing the quality of purified water and its potential environmental consequences. Using the water quality index (WQI) as a comprehensive metric for water quality assessment, the study investigates the effects of key parameters such as pH, temperature, phosphates, and hydrocarbons derived from an oil refining complex WWTP. The aim is to contribute to water resource management and environmental monitoring. Machine learning models were trained on Principal Component Analysis (PCA) data, representing 70 % of the total data. The results show that water quality indices vary significantly across seasons, with maximum values for WQI in autumn (40.66), winter (32.96), spring (37.92), and summer (49.34). The ANN and RSM-BBD models effectively forecast experimental values with high predictive accuracy indicated by determination coefficients (R2). For WQI in autumn, winter, spring, and summer, R2 values for ANN were 0.9897, 0.9834, 0.9909, and 0.9956, respectively, and 0.9279, 0.9378, 0.9802, and 0.98866 for RSM-BBD. While the ANN model outperformed the RSM-BBD model, both demonstrated efficacy in predicting water quality indices. The study adds to our understanding of the ecological implications of discharged water from petroleum complexes by providing valuable insights into seasonal variations in water quality factors.
更多查看译文
关键词
Wastewater treatment plant,Water quality index,Prediction,Box Behnken design,Artificial neural network,Response surface methodology
AI 理解论文
溯源树
样例
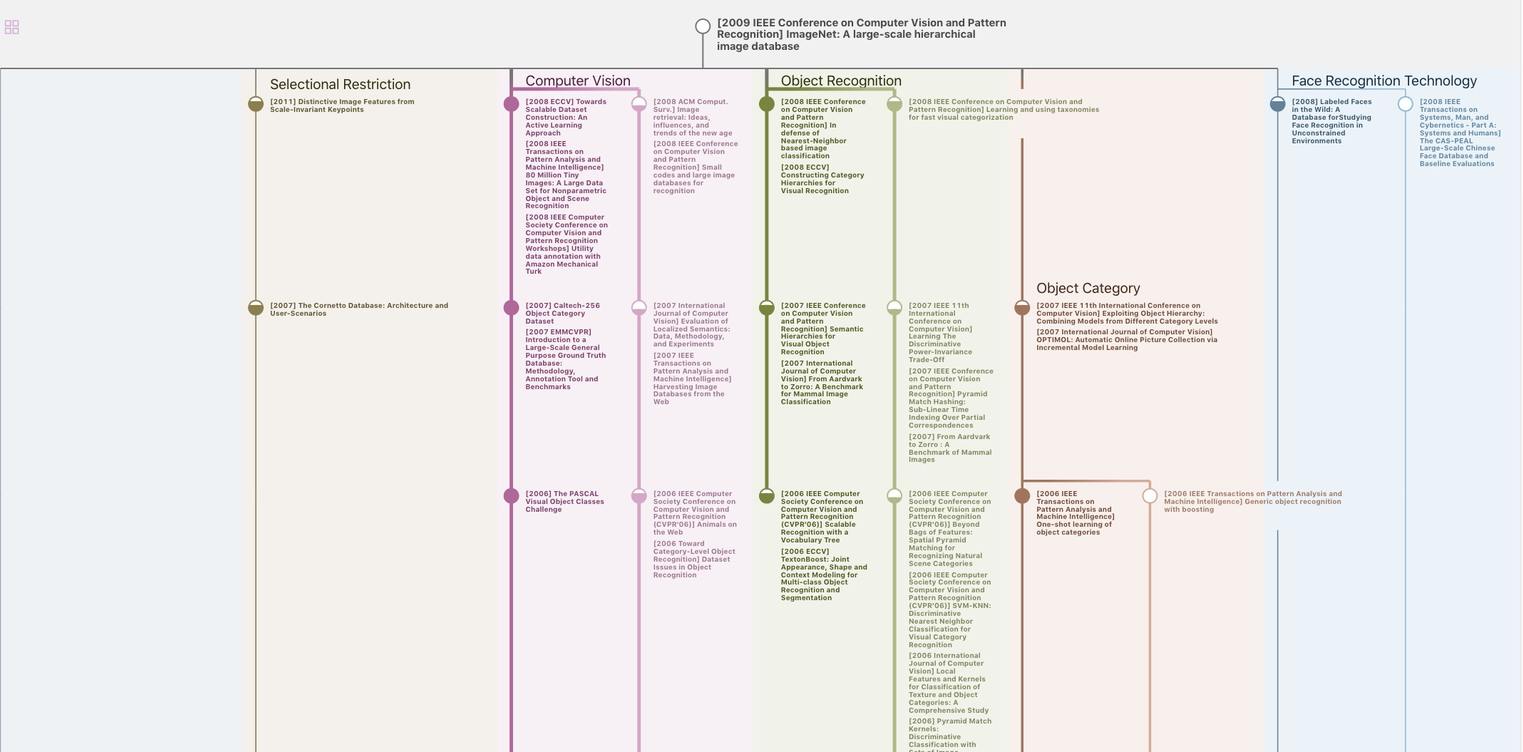
生成溯源树,研究论文发展脉络
Chat Paper
正在生成论文摘要