Machine learning unveils RNA polymerase II binding as a predictor for SMAD2-dependent transcription dynamics in response to Actvin signalling
IET SYSTEMS BIOLOGY(2024)
摘要
The transforming growth factor-beta (TGF-beta) superfamily, including Nodal and Activin, plays a critical role in various cellular processes. Understanding the intricate regulation and gene expression dynamics of TGF-beta signalling is of interest due to its diverse biological roles. A machine learning approach is used to predict gene expression patterns induced by Activin using features, such as histone modifications, RNA polymerase II binding, SMAD2-binding, and mRNA half-life. RNA sequencing and ChIP sequencing datasets were analysed and differentially expressed SMAD2-binding genes were identified. These genes were classified into activated and repressed categories based on their expression patterns. The predictive power of different features and combinations was evaluated using logistic regression models and their performances were assessed. Results showed that RNA polymerase II binding was the most informative feature for predicting the expression patterns of SMAD2-binding genes. The authors provide insights into the interplay between transcriptional regulation and Activin signalling and offers a computational framework for predicting gene expression patterns in response to cell signalling. The authors used machine learning to predict gene expression patterns induced by Activin, a member of the TGF-beta superfamily. RNA polymerase II binding emerged as the most informative feature for predicting expression patterns of SMAD2-binding genes. These findings shed light on Activin signalling regulation and offer a computational framework for predicting gene expression in response to cell signalling.image
更多查看译文
关键词
bioinformatics,learning (artificial intelligence),signal transduction
AI 理解论文
溯源树
样例
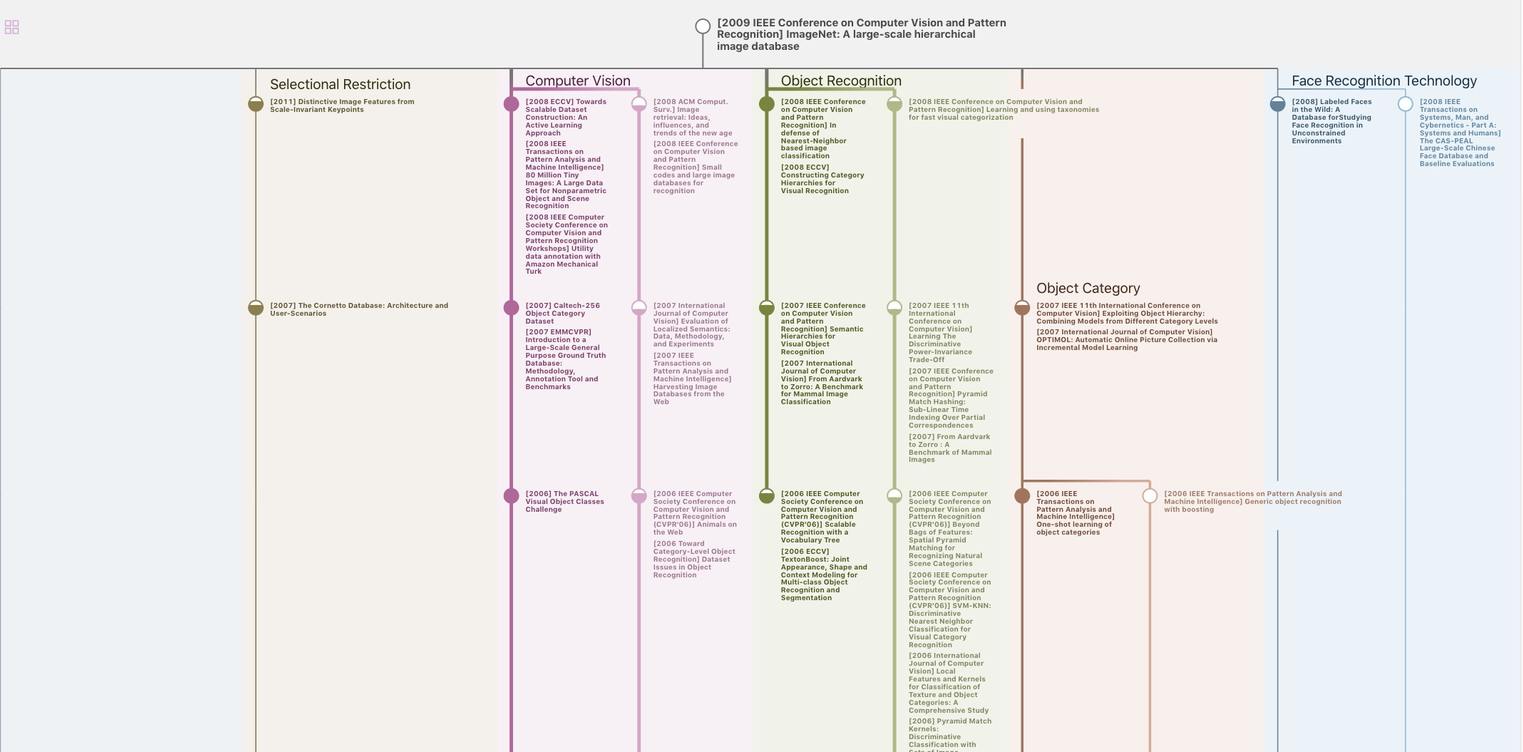
生成溯源树,研究论文发展脉络
Chat Paper
正在生成论文摘要