Learning and Controlling Multiscale Dynamics in Spiking Neural Networks Using Recursive Least Square Modifications.
IEEE transactions on cybernetics(2024)
摘要
Invasive brain-computer interfaces (BCIs) have the capability to simultaneously record discrete signals across multiple scales, but how to effectively process and analyze these potentially related signals remains an open challenge. This article introduces an innovative approach that merges modern control theory with spiking neural networks (SNNs) to bridge the gap among multiscale discrete information. Specifically, the macroscopic point-to-point trajectory is formulated as an optimal control problem with fixed terminal time and state, and it is iteratively solved using the direct dynamic programming (DDP) algorithm. Additionally, SNN is utilized to simulate microscale neural activities in the premotor cortex, employing the product of the weighted adjacency matrix and the mesoscale firing rate to approximate the macroscopic trajectory. The error between actual macroscale behavior and the preceding approximation is then used to update the weighted adjacency matrix through the recursive least square (RLS) method. Analysis and simulation of various tasks, including low-dimensional point-to-point tasks, high-dimensional complex Lorenz systems, and center-out-and-back tasks, verify the feasibility and interpretability of our method in processing multiscale signals ranging from spiking neurons to motion trajectory through the integration of SNN and control theory.
更多查看译文
关键词
Task analysis,Neurons,Trajectory,Behavioral sciences,Dynamic programming,Control theory,Biology,Direct dynamic programming (DDP),Lorenz system,multiscale dynamics,point-to-point control,recursive least square (RLS),spiking neural network (SNN)
AI 理解论文
溯源树
样例
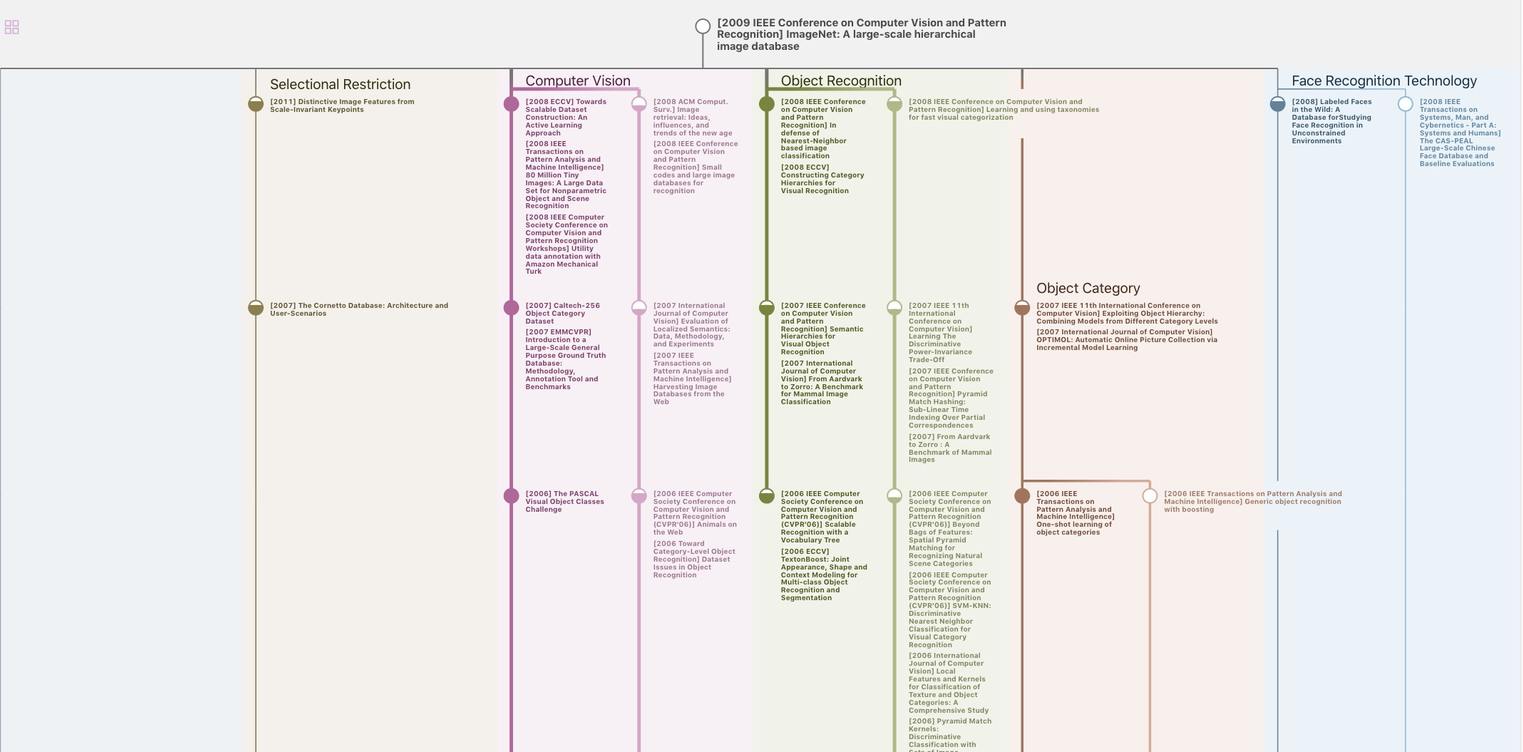
生成溯源树,研究论文发展脉络
Chat Paper
正在生成论文摘要