Assessing Face Image Quality: A Large-Scale Database and a Transformer Method
IEEE TRANSACTIONS ON PATTERN ANALYSIS AND MACHINE INTELLIGENCE(2024)
摘要
The amount of face images has been witnessing an explosive increase in the last decade, where various distortions inevitably exist on transmitted or stored face images. The distortions lead to visible and undesirable degradation on face images, affecting their quality of experience (QoE). To address this issue, this paper proposes a novel Transformer-based method for quality assessment on face images (named as TransFQA). Specifically, we first establish a large-scale face image quality assessment (FIQA) database, which includes 42,125 face images with diversifying content at different distortion types. Through an extensive crowdsource study, we obtain 712,808 subjective scores, which to the best of our knowledge contribute to the largest database for assessing the quality of face images. Furthermore, by investigating the established database, we comprehensively analyze the impacts of distortion types and facial components (FCs) on the overall image quality. Accordingly, we propose the TransFQA method, in which the FC-guided Transformer network (FT-Net) is developed to integrate the global context, face region and FC detailed features via a new progressive attention mechanism. Then, a distortion-specific prediction network (DP-Net) is designed to weight different distortions and accurately predict final quality scores. Finally, the experiments comprehensively verify that our TransFQA method significantly outperforms other state-of-the-art methods for quality assessment on face images.
更多查看译文
关键词
Face image database,image quality assessment,transformer
AI 理解论文
溯源树
样例
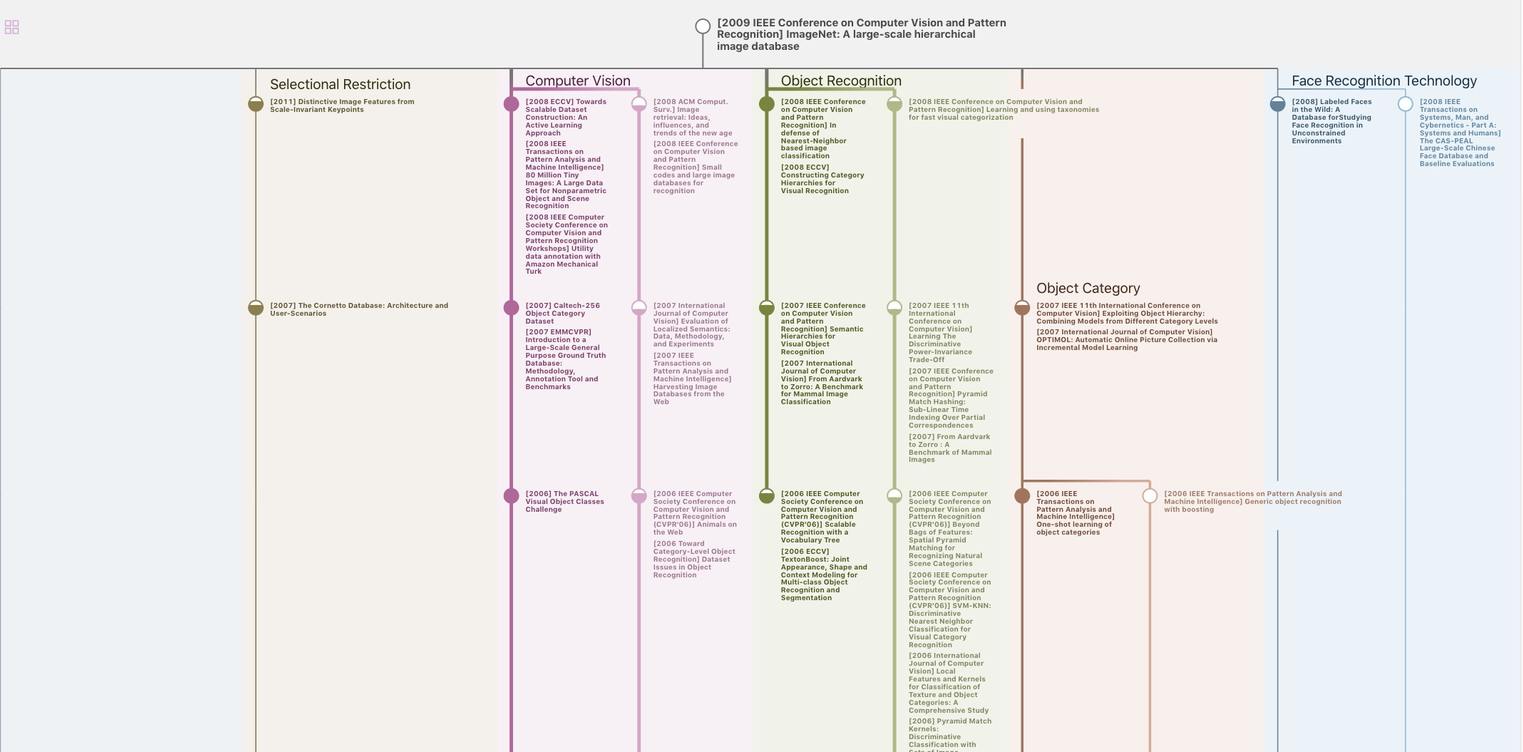
生成溯源树,研究论文发展脉络
Chat Paper
正在生成论文摘要