A Generalized Framework for Agricultural Field Delineation from High-Resolution Satellite Imageries
INTERNATIONAL JOURNAL OF DIGITAL EARTH(2024)
摘要
Accurate digital data on agricultural fields are crucial for various agriculture applications. While deep learning methods have shown promise in delineating fields from high-resolution imagery, there is a lack of research evaluating key techniques for field boundary detection. Challenges also exist in converting detection results into high-quality fields. This study addresses these issues and proposes a generalized framework for agricultural field delineation (GF-AFD). First, we identify three key techniques for field boundary detection and apply them to MPSPNet model. Ablation and comparison experiments demonstrate significant performance enhancements due to techniques. They also prove effective for DeeplabV3+ model, which shares a similar architecture. The modified models outperform U-Net-based models and approach the state-of-the-art models. Second, we show that performing region segmentation on boundary results yields improved field shapes. To address weak boundary loss and unstable parameters issues in existing segmentation-based methods, we introduce the OWT method to enhance weak boundaries directionally before segmentation. We also develop a hierarchical merging method, leveraging the observational hierarchy of fields, resulting in stable parameters across regions and models. The proposed GF-AFD framework was validated cross three diverse Chinese counties. The results demonstrate the framework's robust performance, providing a valuable solution for delineating agricultural fields.
更多查看译文
关键词
Agriculture,agricultural field delineation,convolution neural network,high spatial resolution image,generalization
AI 理解论文
溯源树
样例
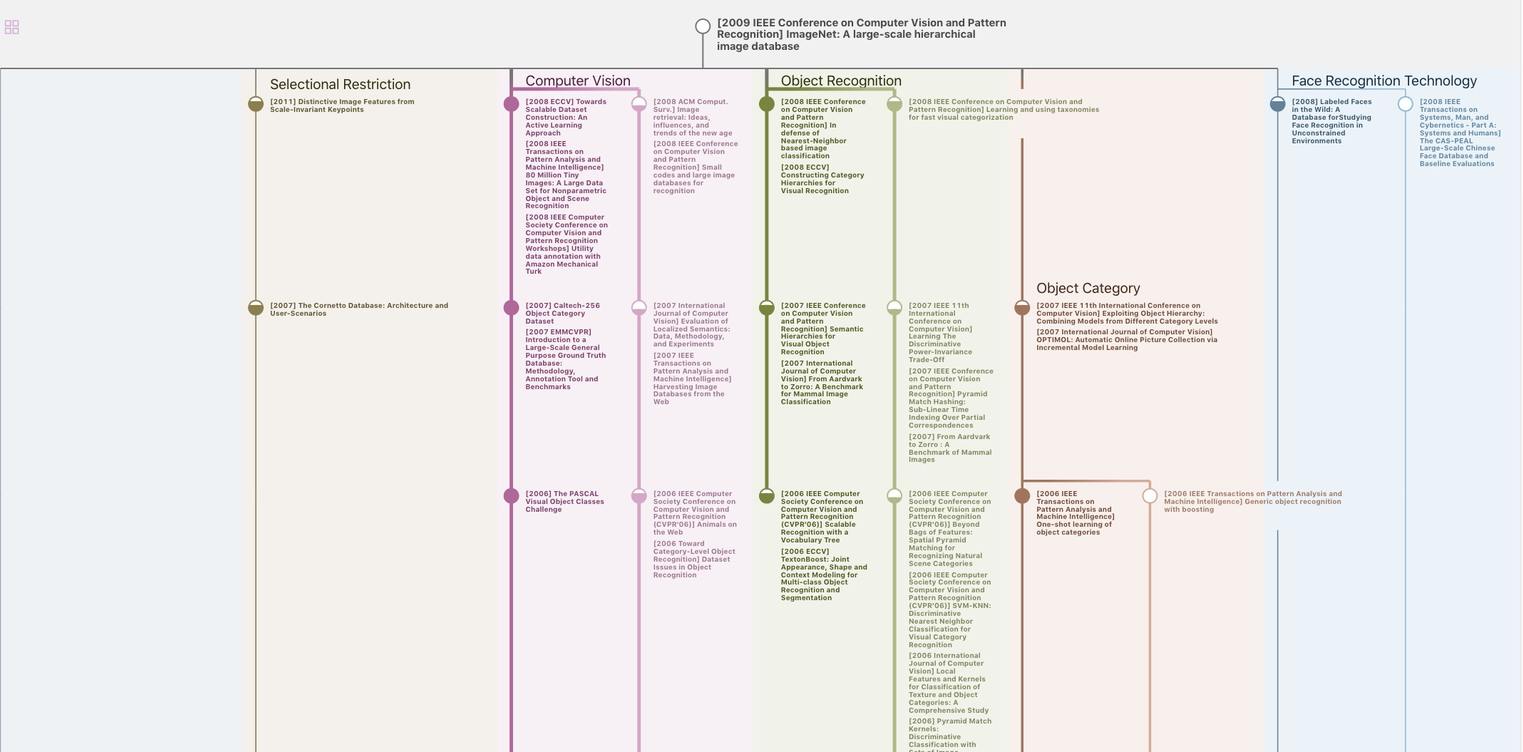
生成溯源树,研究论文发展脉络
Chat Paper
正在生成论文摘要