Error-Sensitivity-Aware Write-Energy Optimization for an MTJ-Based Binarized Neural Network.
2023 30th IEEE International Conference on Electronics, Circuits and Systems (ICECS)(2023)
摘要
This paper proposes a write-energy optimization method in the processing of binarized neural networks (BNNs) utilizing the stochastic properties of magnetic tunnel junction (MTJ) devices for energy-efficient artificial intelligence (AI) applications. By exploiting the high bit-error tolerance of BNNs, the performance requirements for writing to MTJ-based on-chip buffer memory can be relaxed, resulting in the realization of an energy-efficient BNN. Furthermore, by utilizing the fact that each layer of the BNN has a different sensitivity to bit errors, it is possible to optimize the write energy on a layer-by-layer basis. Through the evaluation results for BNNs with various error conditions, it is demonstrated that the proposed method reduces write energy for MTJ-based on-chip buffer memory by 14% compared with the conventional method.
更多查看译文
关键词
MTJ device,switching probability,binarized neural network,deep learning
AI 理解论文
溯源树
样例
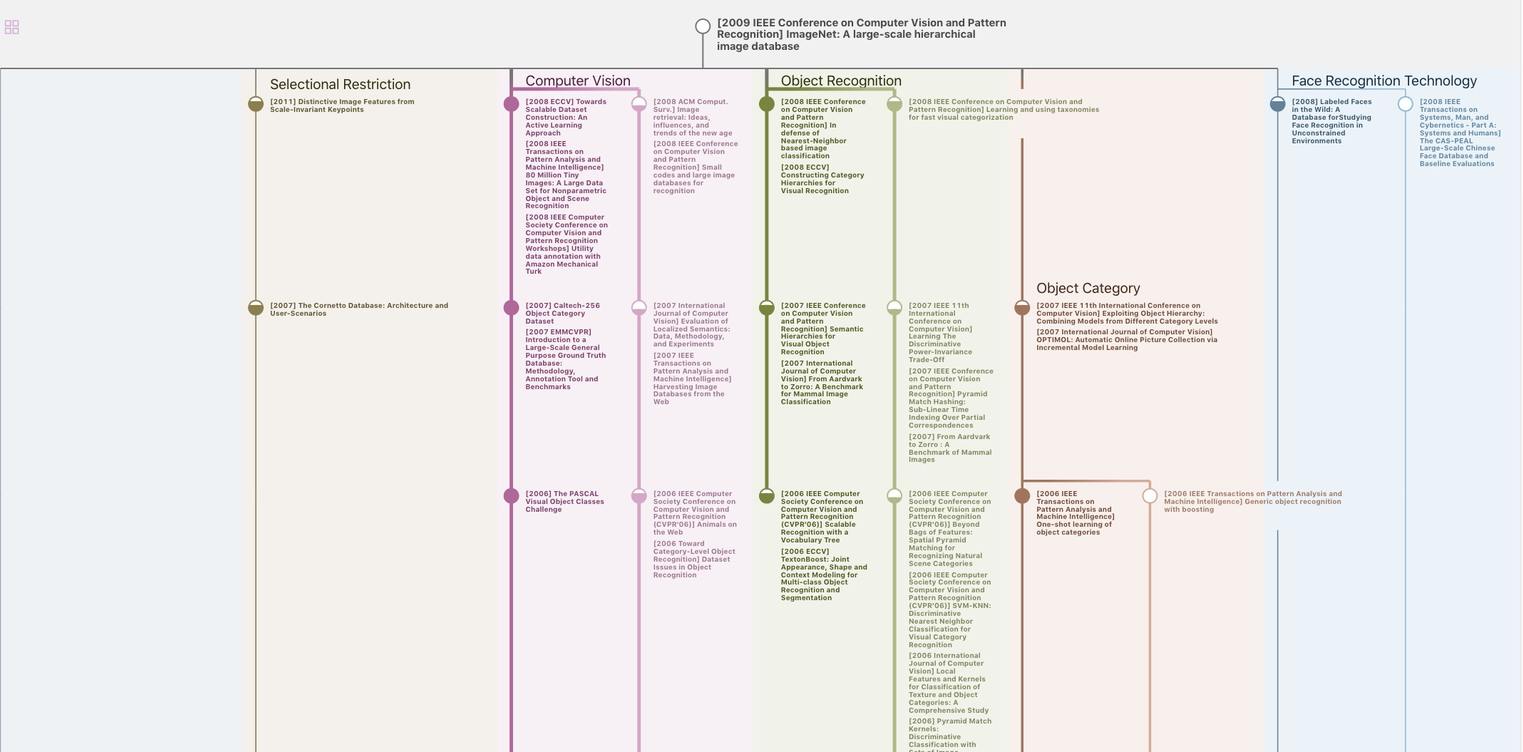
生成溯源树,研究论文发展脉络
Chat Paper
正在生成论文摘要