A Closed-Loop Falls Monitoring and Prevention App for Multiple Sclerosis Clinical Practice: Human-Centered Design of the Multiple Sclerosis Falls InsightTrack
JMIR HUMAN FACTORS(2024)
摘要
Background: Falls are common in people with multiple sclerosis (MS), causing injuries, fear of falling, and loss of independence. Although targeted interventions (physical therapy) can help, patients underreport and clinicians undertreat this issue. Patient-generated data, combined with clinical data, can support the prediction of falls and lead to timely intervention (including referral to specialized physical therapy). To be actionable, such data must be efficiently delivered to clinicians, with care customized to the patient's specific context. Objective: This study aims to describe the iterative process of the design and development of Multiple Sclerosis Falls InsightTrack (MS-FIT), identifying the clinical and technological features of this closed-loop app designed to support streamlined falls reporting, timely falls evaluation, and comprehensive and sustained falls prevention efforts. Methods: Stakeholders were engaged in a double diamond process of human-centered design to ensure that technological features aligned with users' needs. Patient and clinician interviews were designed to elicit insight around ability blockers and boosters using the capability, opportunity, motivation, and behavior (COM-B) framework to facilitate subsequent mapping to the Behavior Change Wheel. To support generalizability, patients and experts from other clinical conditions associated with falls (geriatrics, orthopedics, and Parkinson disease) were also engaged. Designs were iterated based on each round of feedback, and final mock-ups were tested during routine clinical visits. Results: A sample of 30 patients and 14 clinicians provided at least 1 round of feedback. To support falls reporting, patients favored a simple biweekly survey built using REDCap (Research Electronic Data Capture; Vanderbilt University) to support bring-your-own-device accessibility-with optional additional context (the severity and location of falls). To support the evaluation and prevention of falls, clinicians favored a clinical dashboard featuring several key visualization widgets: a longitudinal falls display coded by the time of data capture, severity, and context; a comprehensive, multidisciplinary, and evidence-based checklist of actions intended to evaluate and prevent falls; and MS resources local to a patient's community. In-basket messaging alerts clinicians of severe falls. The tool scored highly for usability, likability, usefulness, and perceived effectiveness (based on the Health IT Usability Evaluation Model scoring). Conclusions: To our knowledge, this is the first falls app designed using human-centered design to prioritize behavior change and, while being accessible at home for patients, to deliver actionable data to clinicians at the point of care. MS-FIT streamlines data delivery to clinicians via an electronic health record-embedded window, aligning with the 5 rights approach. Leveraging MS-FIT for data processing and algorithms minimizes clinician load while boosting care quality. Our innovation seamlessly integrates real-world patient-generated data as well as clinical and community-level factors, empowering self-care and addressing the impact of falls in people with MS. Preliminary findings indicate wider relevance, extending to other neurological conditions associated with falls and their consequences.
更多查看译文
关键词
digital health,mobile tools,falls,prevention,behavioral medicine,implementation science,closed-loop monitoring,multiple sclerosis,mobile phone
AI 理解论文
溯源树
样例
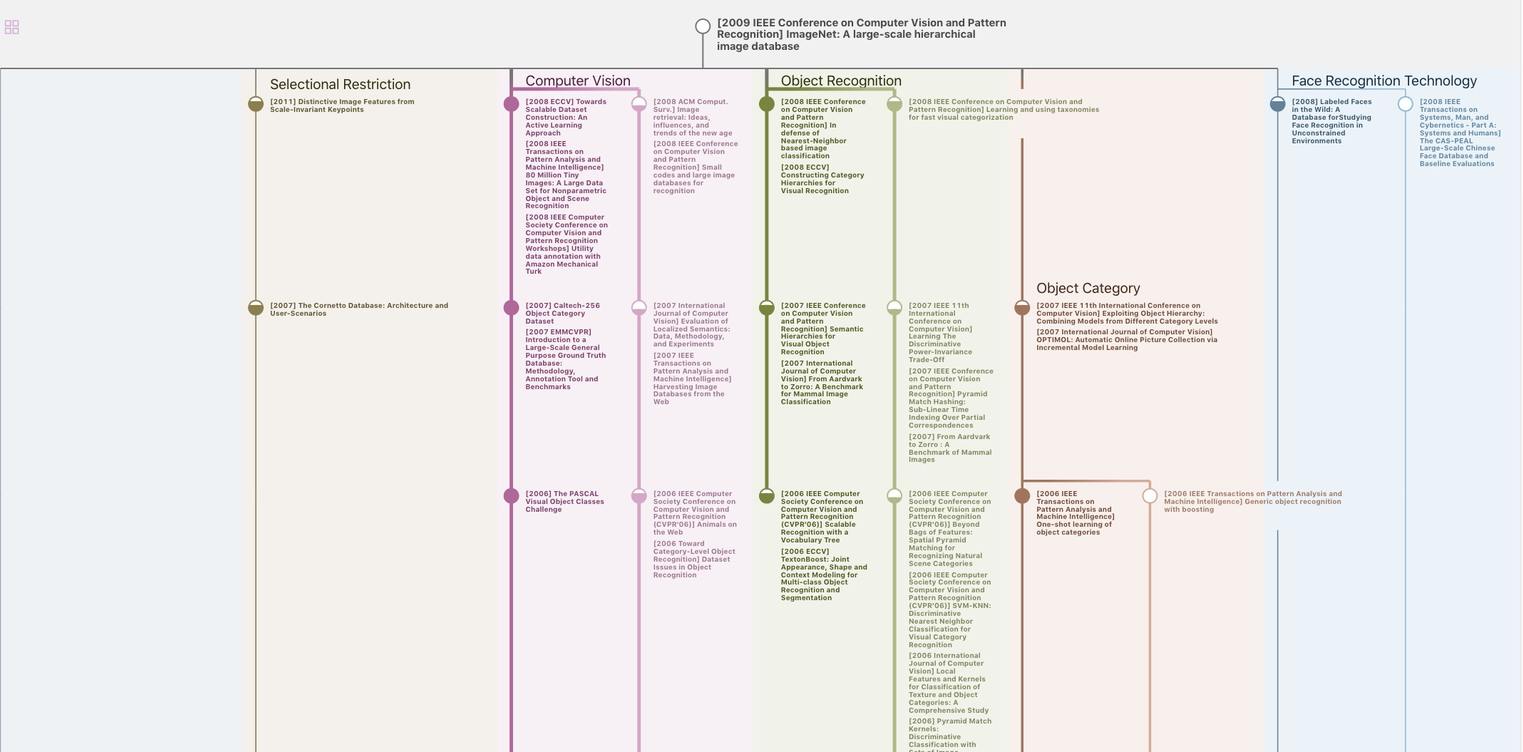
生成溯源树,研究论文发展脉络
Chat Paper
正在生成论文摘要