Current Effect-Eliminated Optimal Target Assignment and Motion Planning for a Multi-UUV System
IEEE TRANSACTIONS ON INTELLIGENT TRANSPORTATION SYSTEMS(2024)
摘要
The paper presents an innovative approach (CBNNTAP) that addresses the complexities and challenges introduced by ocean currents when optimizing target assignment and motion planning for a multi-unmanned underwater vehicle (UUV) system. The core of the proposed algorithm involves the integration of several key components. Firstly, it incorporates a bio-inspired neural network-based (BINN) approach which predicts the most efficient paths for individual UUVs while simultaneously ensuring collision avoidance among the vehicles. Secondly, an efficient target assignment component is integrated by considering the path distances determined by the BINN algorithm. In addition, a critical innovation within the CBNNTAP algorithm is its capacity to address the disruptive effects of ocean currents, where an adjustment component is seamlessly integrated to counteract the deviations caused by these currents, which enhances the accuracy of both motion planning and target assignment for the UUVs. The effectiveness of the CBNNTAP algorithm is demonstrated through comprehensive simulation results and the outcomes underscore the superiority of the developed algorithm in nullifying the effects of static and dynamic ocean currents in 2D and 3D scenarios.
更多查看译文
关键词
Planning,Neurons,Oceans,Heuristic algorithms,Biological neural networks,Vehicle dynamics,Path planning,Bio-inspired neural network,current effect,motion planning,target assignment,unmanned underwater vehicle
AI 理解论文
溯源树
样例
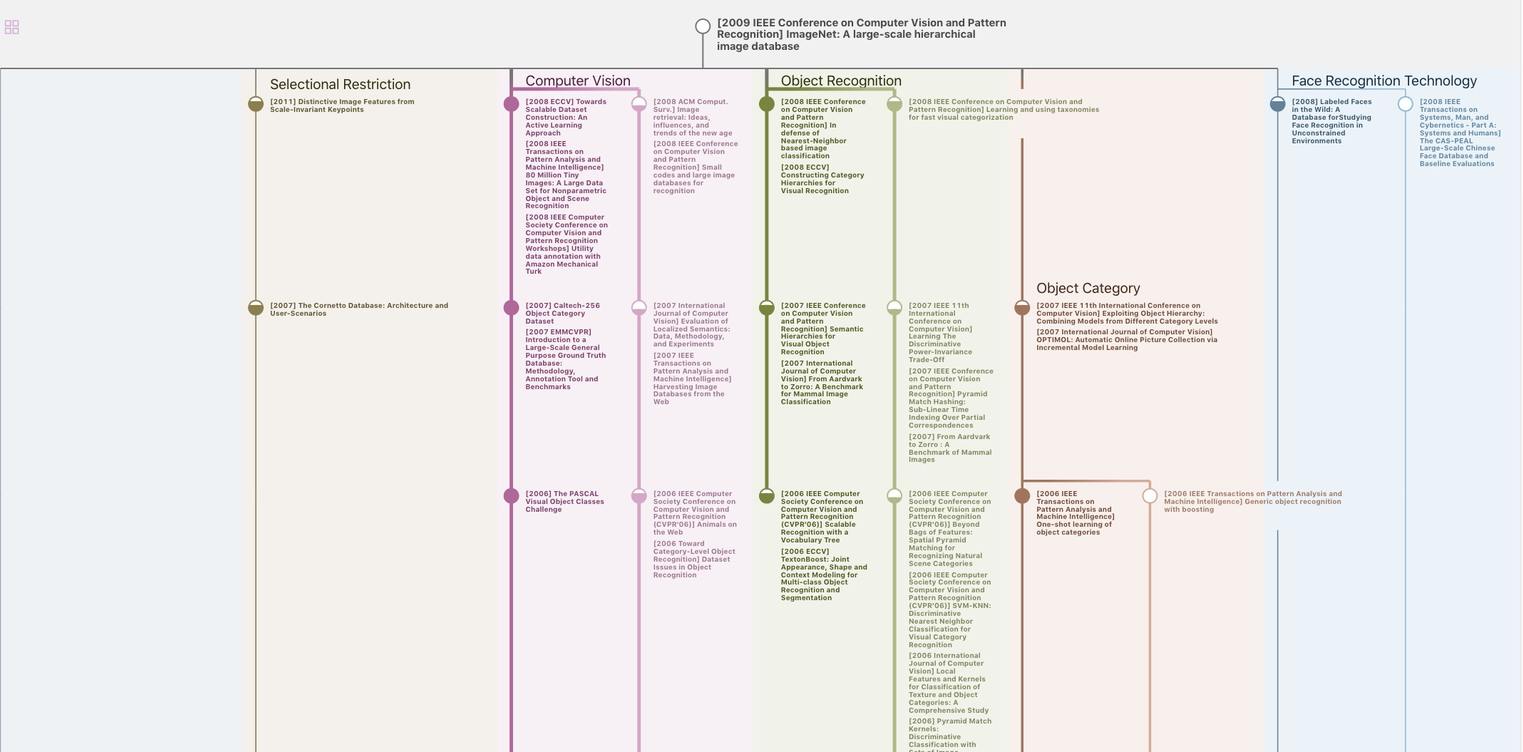
生成溯源树,研究论文发展脉络
Chat Paper
正在生成论文摘要