End-to-End Learning for SLP-Based ISAC Systems
2024 IEEE Wireless Communications and Networking Conference (WCNC)(2024)
摘要
Integrated sensing and communication (ISAC) is an encouraging wirelesstechnology which can simultaneously perform both radar and communicationfunctionalities by sharing the same transmit waveform, spectral resource, andhardware platform. Recently emerged symbol-level precoding (SLP) techniqueexhibits advancement in ISAC systems by leveraging the waveform design degreesof freedom (DoFs) in both temporal and spatial domains. However, traditionalSLP-based ISAC systems are designed in a modular paradigm, which potentiallylimits the overall performance of communication and radar sensing. The highcomplexity of existing SLP design algorithms is another issue that hurdles thepractical deployment. To break through the bottleneck of these approaches, inthis paper we propose an end-to-end approach to jointly design the SLP-baseddual-functional transmitter and receivers of communication and radar sensing.In particular, we aim to utilize deep learning-based methods to minimize thesymbol error rate (SER) of communication users, maximize the detectionprobability, and minimize the root mean square error (RMSE) of the target angleestimation. Multi-layer perceptron (MLP) networks and a long short term memory(LSTM) network are respectively applied to the transmitter, communication usersand radar receiver. Simulation results verify the feasibility of the proposeddeep-learning-based end-to-end optimization for ISAC systems and reveal theeffectiveness of the proposed neural networks for the end-to-end design.
更多查看译文
关键词
Integrated sensing and communication (ISAC),symbol-level precoding (SLP),end-to-end (E2E) learning
AI 理解论文
溯源树
样例
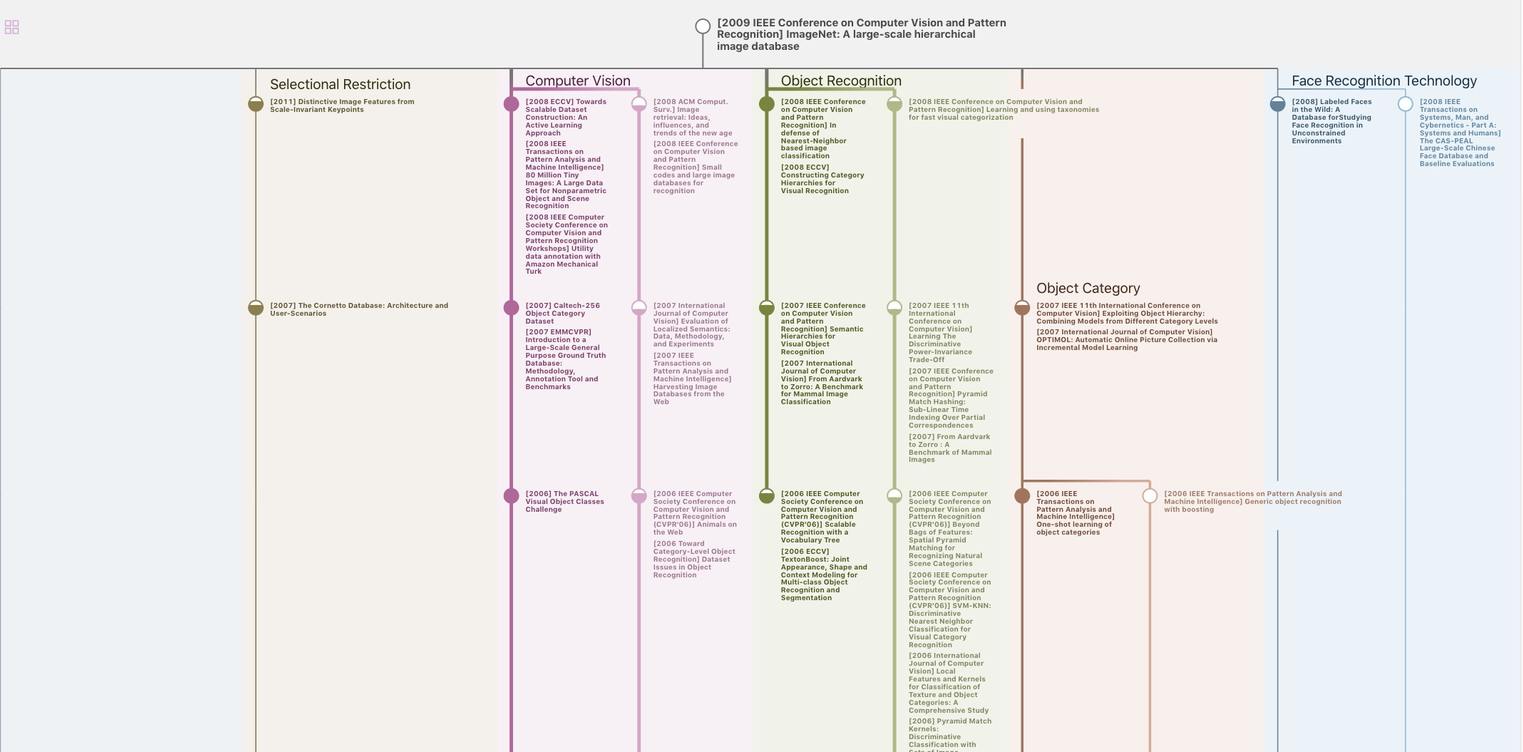
生成溯源树,研究论文发展脉络
Chat Paper
正在生成论文摘要