Wavelet-Inspired Multiscale Graph Convolutional Recurrent Network for Traffic Forecasting
IEEE International Conference on Acoustics, Speech, and Signal Processing(2024)
摘要
Traffic forecasting is the foundation for intelligent transportation systems.Spatiotemporal graph neural networks have demonstrated state-of-the-artperformance in traffic forecasting. However, these methods do not explicitlymodel some of the natural characteristics in traffic data, such as themultiscale structure that encompasses spatial and temporal variations atdifferent levels of granularity or scale. To that end, we propose aWavelet-Inspired Graph Convolutional Recurrent Network (WavGCRN) which combinesmultiscale analysis (MSA)-based method with Deep Learning (DL)-based method. InWavGCRN, the traffic data is decomposed into time-frequency components withDiscrete Wavelet Transformation (DWT), constructing a multi-stream inputstructure; then Graph Convolutional Recurrent networks (GCRNs) are employed asencoders for each stream, extracting spatiotemporal features in differentscales; and finally the learnable Inversed DWT and GCRN are combined as thedecoder, fusing the information from all streams for traffic metricsreconstruction and prediction. Furthermore, road-network-informed graphs anddata-driven graph learning are combined to accurately capture spatialcorrelation. The proposed method can offer well-defined interpretability,powerful learning capability, and competitive forecasting performance onreal-world traffic data sets.
更多查看译文
关键词
Spatiotemporal correlation,wavelet-inspired network,graph learning,traffic forecasting
AI 理解论文
溯源树
样例
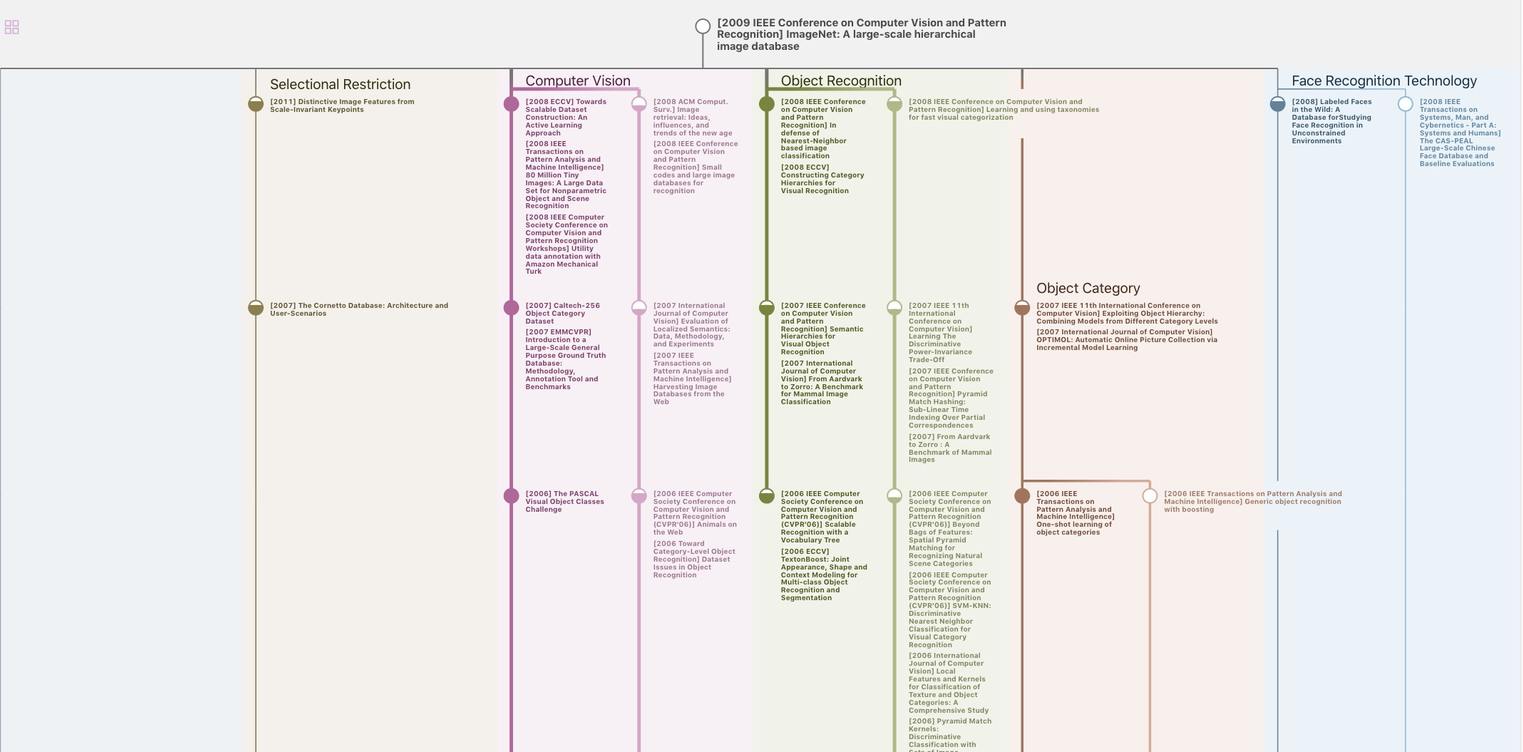
生成溯源树,研究论文发展脉络
Chat Paper
正在生成论文摘要