A 4D strong spatio-temporal feature learning network for behavior recognition of point cloud sequences
Multimedia Tools and Applications(2024)
摘要
Although the depth map sequence widely used in behavior recognition can provide depth information. However, depth pixels are not strongly correlated with each other, and the spatio-temporal structure information of behavior data is largely lost. Point cloud data can provide rich spatial information and geometric features, which make up for the lack of depth images. In order to further utilize the geometric information of behavior action and improve the utilization rate of spatio-temporal structure information, this paper proposed a 4D strong spatio-temporal feature learning network for behavior recognition of point cloud sequences. Coordinate transformation was performed on a depth dataset to generate a point cloud dataset, then our network processed each frame of point cloud data and learned 4D strong spatio-temporal features (three spatial and one temporal dimension). The network consists of two modules, a spatial-level feature learning module and a temporal-level position encoding module. In the spatial-level feature learning module, the spatial dimension of the point cloud is processed and learned. Each frame of point cloud data outputs a feature sequence through two progressive structure enhanced set abstract layers, which represents the strong spatial structure. Then, it becomes a complete spatial-level feature sequence through a maxing pooling operation. In the temporal-level position coding module, the processing and learning of the time dimension of the point cloud are performed. The time-series information is injected into the feature sequence through position coding and so on. Finally, the multi-level features of human actions are aggregated and classified. It was carried out on three public datasets. Extensive experiments showed that the network structure proposed in this paper outperformed the current state-of-the-art methods.
更多查看译文
关键词
Point cloud sequence,Coordinate transformation,Spatio-temporal information,Feature enhancement
AI 理解论文
溯源树
样例
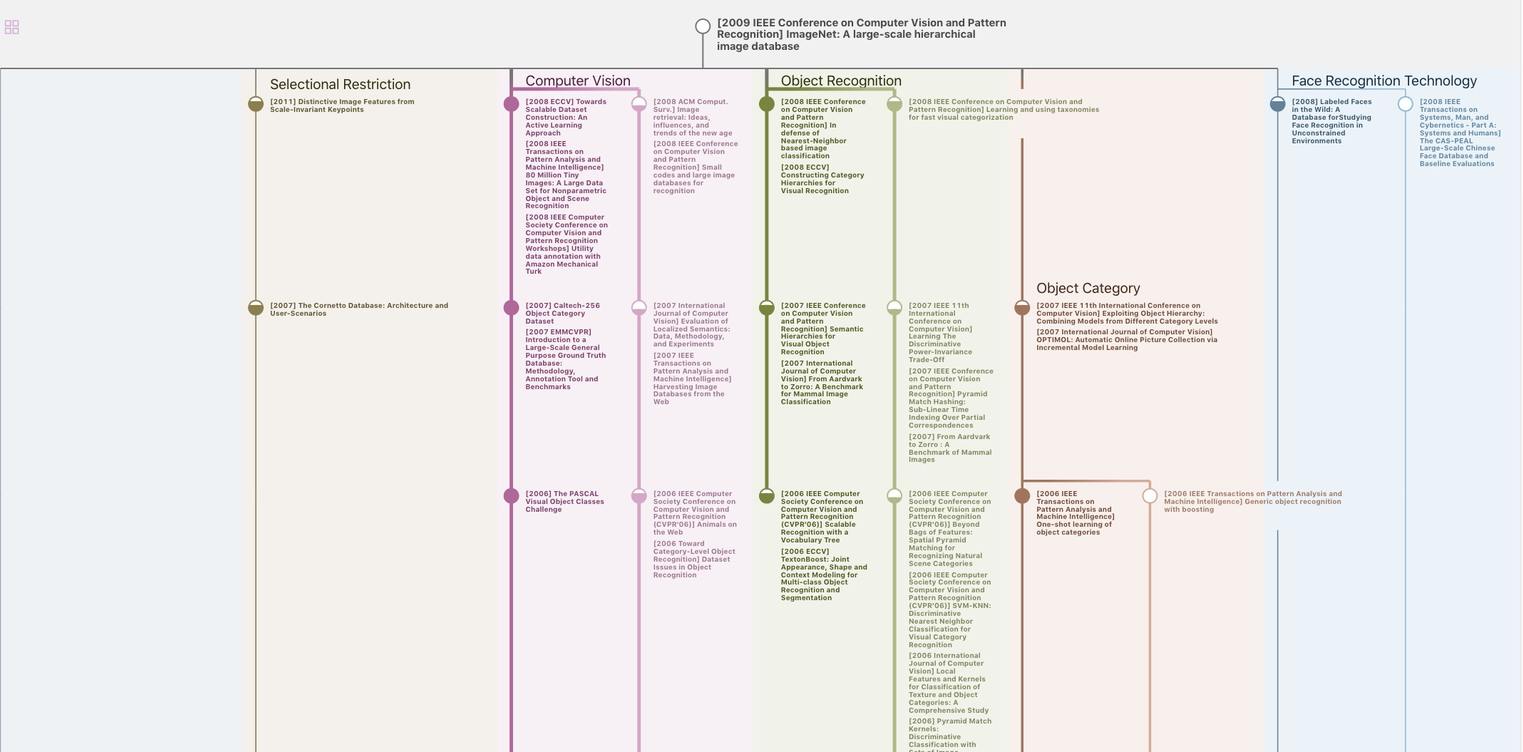
生成溯源树,研究论文发展脉络
Chat Paper
正在生成论文摘要