Video Summarization Using Knowledge Distillation-Based Attentive Network
Cognitive computation(2024)
摘要
The vast volumes of videos produced daily require highly efficient measures to ensure that key information is reported for effective review and storage, which leads to the popularity of video summarization techniques. Deep learning has shown its advantages in video summarization, especially convolutional neural network, which are effective in extracting features for video summarization. However, the deep network layers and the limited range of temporal dependence make it challenging to deploy the network and thus affect the accuracy of identifying important video frames. To tackle these issues, we present a knowledge distillation-based attentive network (KDAN) for supervised video summarization in this paper. The proposed method separates the full convolutional network from the attention mechanism based on the idea of education and learning processes in biology and uses a full convolutional network as a teacher network to guide the learning of the student network consisting of an attention mechanism. The obtained lightweight network considers the knowledge learned from both networks, thus solving the problems of explosion in the number of participants and slow training. We have conducted experiments on two widely used benchmarks SumMe and TVSum. DANtea achieves F-scores 53.09 and 60.30, and DAN achieves F-scores 51.26 and 61.55 in Canonical settings on the SumMe and TVSum datasets, respectively. Experiments on two public benchmarks SumMe and TVSum demonstrate the effectiveness and superiority of the proposed network over existing state-of-the-art methods.
更多查看译文
关键词
Video summarization,Knowledge distillation,Dilated convolution,Dual attention,Attentive network
AI 理解论文
溯源树
样例
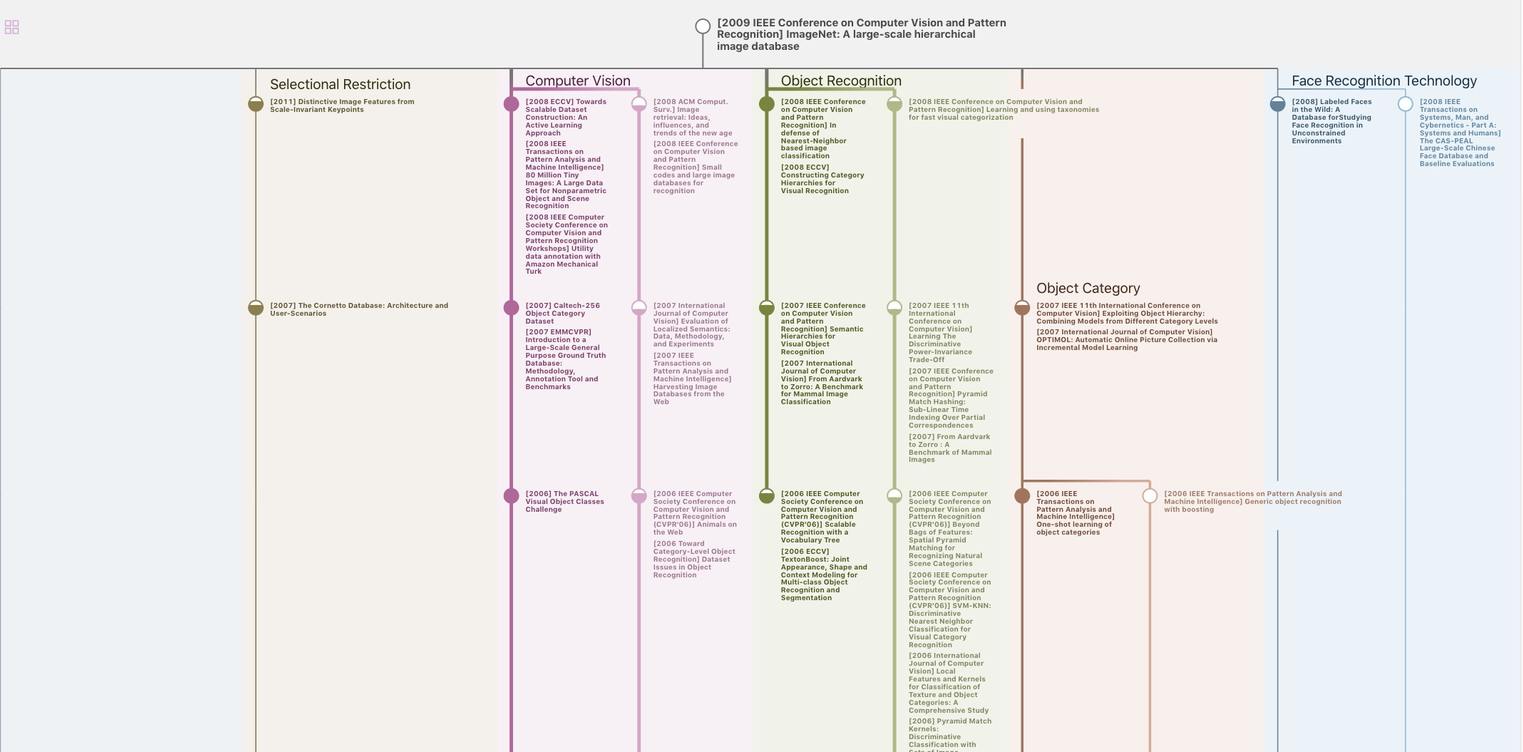
生成溯源树,研究论文发展脉络
Chat Paper
正在生成论文摘要