Generating CGAN-based Synthetic Dermoscopy Images and Classifying with Deep Lightweight CNN
2023 International Conference on Computing, Networking, Telecommunications & Engineering Sciences Applications (CoNTESA)(2023)
摘要
Effective dermatological diagnosis and treatments significantly depend on the accurate categorisation of the skin lesions. It is difficult to classify skin lesions using automated diagnostic techniques due to class-imbalance datasets and the absence of labelled data. The applications of various deep learning methods have demonstrated superior performance in medical diagnosis in the recent past. Unfortunately, training these models requires a substantial quantity of labelled instances. A novel approach, combining Deep Learning convolutional neural networks (CNNs) as well as conditional generative adversarial networks (CGANs), for classifying skin lesion images, has been presented in this study. CGAN is an enhanced version of the GAN, in which the generator takes input images with specific class labels. Applying conditions for minority classes can help to equalise the dataset by increasing the sample size for those minority classes. In this research, CGANs were used to produce synthetic images of minority classes for a skin lesion dataset named PAD UFES-20. The images generated from CGANS were added then to the original dataset to train a lightweight deep-learning CNN network named MobileNetv2. The analysis of the results shows an improvement of 6% average accuracy and 5% recall value, when the synthetic data augmentation was added to the original dataset. This synthetic data augmentation approach can also widely be used in various medical classification applications to reduce the imbalance problems, resulting in enhanced diagnosis.
更多查看译文
关键词
CNN,CGAN,Data Augmentation,Deep Learning,GAN,Image Classification
AI 理解论文
溯源树
样例
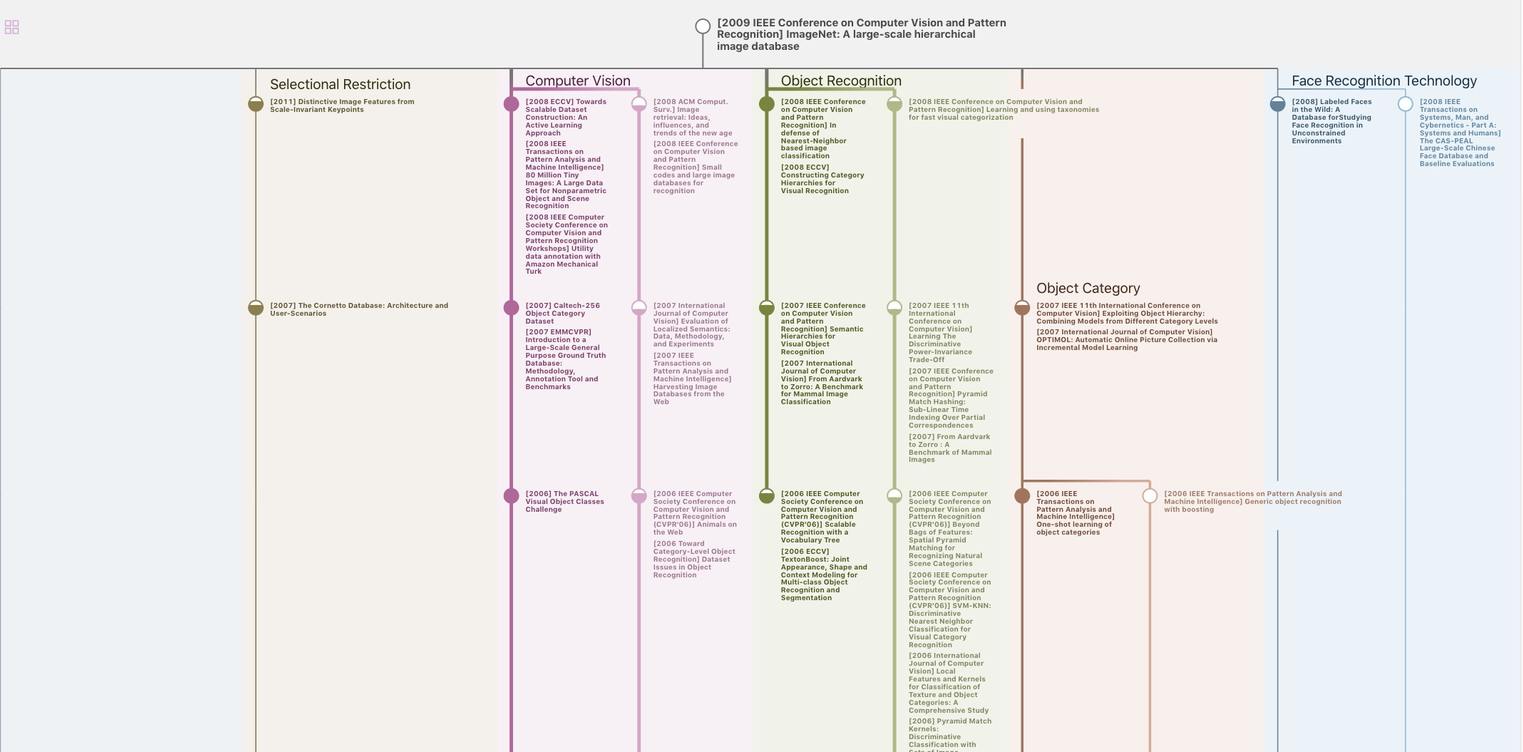
生成溯源树,研究论文发展脉络
Chat Paper
正在生成论文摘要