Spatial-temporal offshore wind speed characteristics prediction based on an improved purely 2D CNN approach in a large-scale perspective using reanalysis dataset
ENERGY CONVERSION AND MANAGEMENT(2024)
摘要
Accurate prediction of spatial-temporal wind speed with a large-scale perspective is vital for the wind farm dynamic regulation, the floating wind turbine deployment and its early warning. Machine-learning approaches have been applied for wind prediction to alleviate the computational challenges of traditional numerical models. Yet, most previous studies emphasized the forecasting accuracy employing location-specific dataset, such methodologies are site-specific and ignore the importance of spatial-temporal fidelity required by the 2D regional wind farm blueprint. Furtherly, the Recurrent Neural Networks based approach they employed exhibit low efficiency in terms of model convergence on the aspect of practical engineering purposes. This study firstly proposed an improved Unet-based machine learning method using residual learning to predict the spatial-temporal wind field in the North Atlantic Ocean with great potential wind-energy in a large spatial-scale perspective. Several state-of-the-art machine learning methods were established for a better performance comparison. A public reanalysis dataset with very high resolution (0.25 degrees x 0.25 degrees) was employed to verify the proposed method with multi-steps ahead predictions, the model performance was furtherly compared with the rest approaches under different criteria, consisting of Pearson correlation coefficient (PCC), Mean absolutely error (MAE), Root mean square error (RMSE) and symmetric mean absolute percentage error (SMAPE), all exhibiting considerable improvements. The overall forecasting RMSE for the proposed method dropped to 0.088 m/s and 0.087 m/s for wind component U and V correspondingly. Furtherly, comprehensive discussions were provided with both temporal and spatial perspective, revealing that the proposed model can offer an accurate 2D wind speed prediction along timeline (the PCC equals to 0.99). The proposed data-driven method presents promising potential to accurately preserve the spatial-resolved patterns which are variant in both spatial and temporal dimensions, and show superiority in terms of computational efficiency in terms of achieving large-scale wind pattern predictions.
更多查看译文
关键词
Spatial-temporal wind speed forecasting,purely CNN,Autocorrelation analysis,Deep learning,Spatial -temporal distribution
AI 理解论文
溯源树
样例
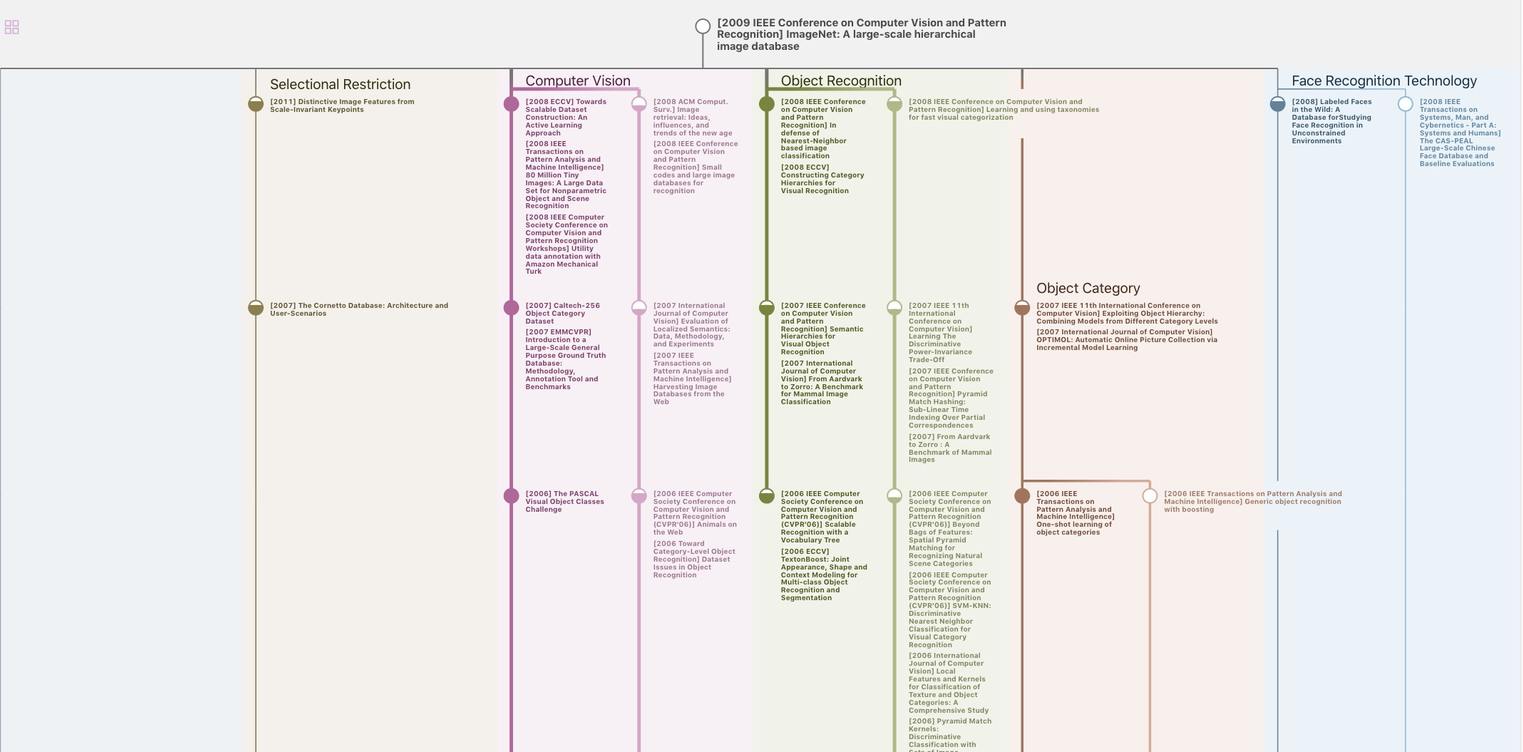
生成溯源树,研究论文发展脉络
Chat Paper
正在生成论文摘要