Deep Learning-Based Seasonal Forecast of Sea Ice Considering Atmospheric Conditions
JOURNAL OF GEOPHYSICAL RESEARCH-ATMOSPHERES(2023)
摘要
The ongoing decline of sea ice in the Arctic has heightened the need for accurate sea-ice forecasts to support environmental protection and resource development in the region and beyond. While deep learning has shown promise in seasonal sea-ice forecasting, most of the existing models overlook the crucial influence of atmospheric factors, thereby limiting their ability to capture the intricate characteristics of the sea-ice system and improve forecast accuracy. To address this deficiency, we propose an attention convolutional long short-term memory ensemble network named Atsicn, which integrates atmospheric factors to enhance the precision of multi-step seasonal sea-ice concentration forecasts. Our findings reveal that Atsicn outperforms state-of-the-art dynamic and statistical models, and demonstrates remarkable reliability in extreme years. Furthermore, the impact of atmospheric factors on sea-ice forecasts exhibits significant seasonality, with a relatively minimal impact on forecasts from March to June, a growing impact from July to October, and a persistent yet diminishing impact from November to February. This study provides a practical approach for seasonal sea-ice forecasts and contributes a new perspective to the understanding of the intricate interplay between sea ice and atmospheric factors. Arctic sea ice is a crucial and sensitive component of the complex Earth system. The ongoing global warming has intensified the instability and unpredictability of the Arctic sea-ice system, emphasizing the need for accurate sea-ice forecasts to support environmental protection and resource development in the Arctic and beyond. In this study, we propose a deep learning approach that considers the influence of atmospheric factors on sea ice to improve the accuracy of the seasonal sea ice concentration forecast. Our findings reveal that the proposed model performs well and maintains remarkable reliability, even in extreme years. The impact of atmospheric factors on sea-ice forecasts exhibits significant seasonality, with a relatively minimal impact on forecasts from March to June, a growing impact from July to October, and a persistent yet diminishing impact from November to February. A deep learning ensemble network integrating atmospheric factors named Atsicn is proposed for the seasonal sea-ice concentration forecastsThe proposed model outperforms state-of-the-art dynamic and statistical models, and demonstrates remarkable reliability in extreme yearsThe results highlight the pronounced seasonality in the influence of atmospheric factors on the sea-ice concentration forecasts
更多查看译文
关键词
sea-ice forecasts,sea ice concentration,deep learning,atmospheric factors
AI 理解论文
溯源树
样例
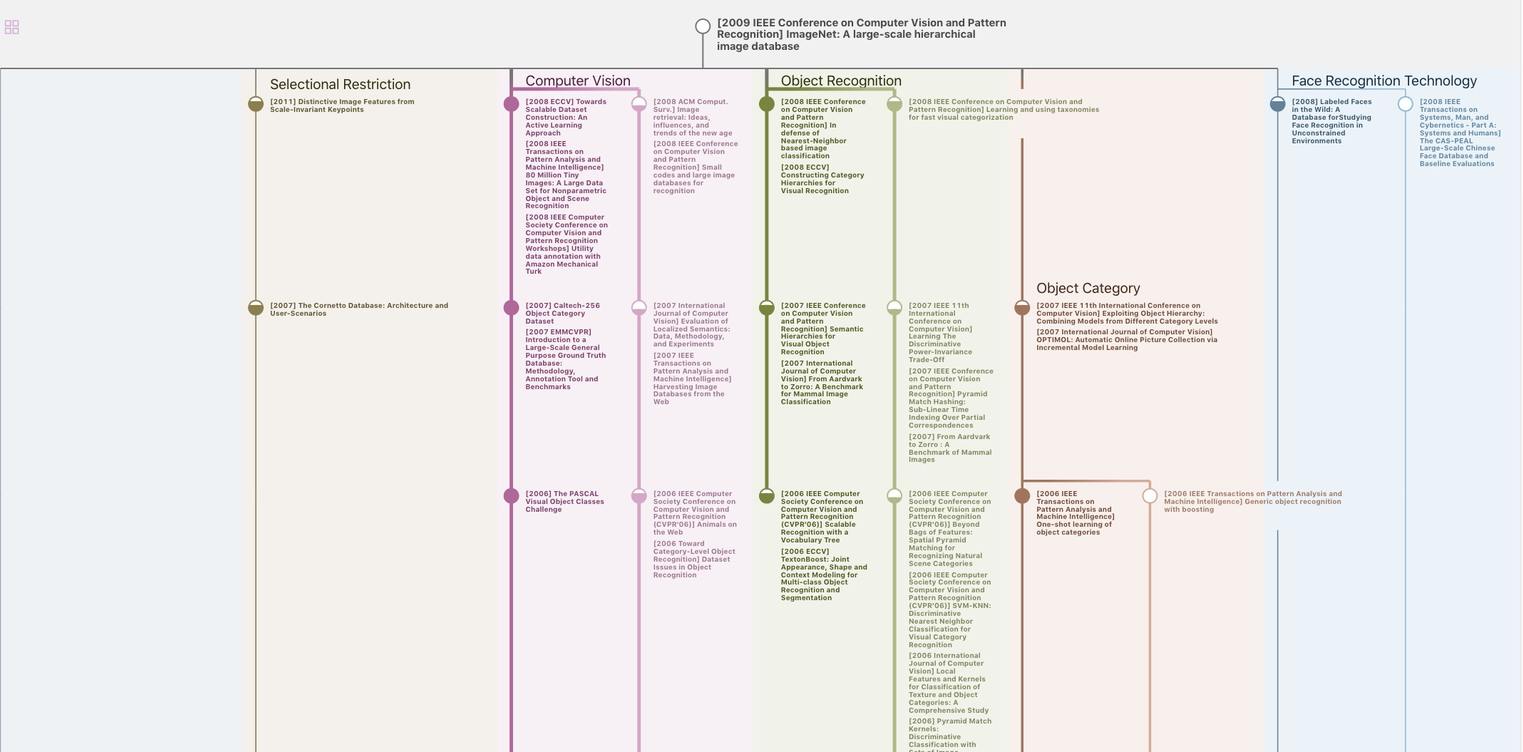
生成溯源树,研究论文发展脉络
Chat Paper
正在生成论文摘要