Seismic Damage Identification by Graph Convolutional Autoencoder Using Adjacency Matrix Based on Structural Modes
EARTHQUAKE ENGINEERING & STRUCTURAL DYNAMICS(2024)
摘要
Immediate post-earthquake identification of structural damage is essential to prevent the loss of structural functionality and system failure. Vibration-based damage identification methods have been widely implemented, but most tend to ignore a critical damage-sensitive feature, the spatial correlation between sensor measurements. To this end, this paper proposes near-real-time damage identification by a graph convolutional autoencoder (GCAE) based on seismic responses of the structural system. The GCAE model accurately considers the spatial correlation and structural characteristics using a weighted adjacency matrix based on the structural modes. The proposed model consists of three main parts: (1) an "encoder" that learns latent features of the input data by considering the spatial information; (2) a "graph structure decoder" that detects anomalies in spatial correlation; and (3) a "node feature decoder" that captures changes in vibration signals. The GCAE model is trained to reconstruct structural responses of the target structure and the adjacency matrix in a healthy state. The seismic damage is then identified by the structural damage index calculated based on the difference between the input and reconstructed data. As numerical investigations, the proposed method is applied to two- and three-dimensional steel frame structures. The train, validation, and test datasets are obtained by structural analyses using ground motions from the PEER-NGA strong motion database. The proposed method is verified by the near-real-time simulation using the test dataset. The results show that the proposed GCAE model can accurately identify seismic damage in near-real-time.
更多查看译文
关键词
autoencoder,damage identification,graph convolutional network,near-real-time,seismic response,structural modes
AI 理解论文
溯源树
样例
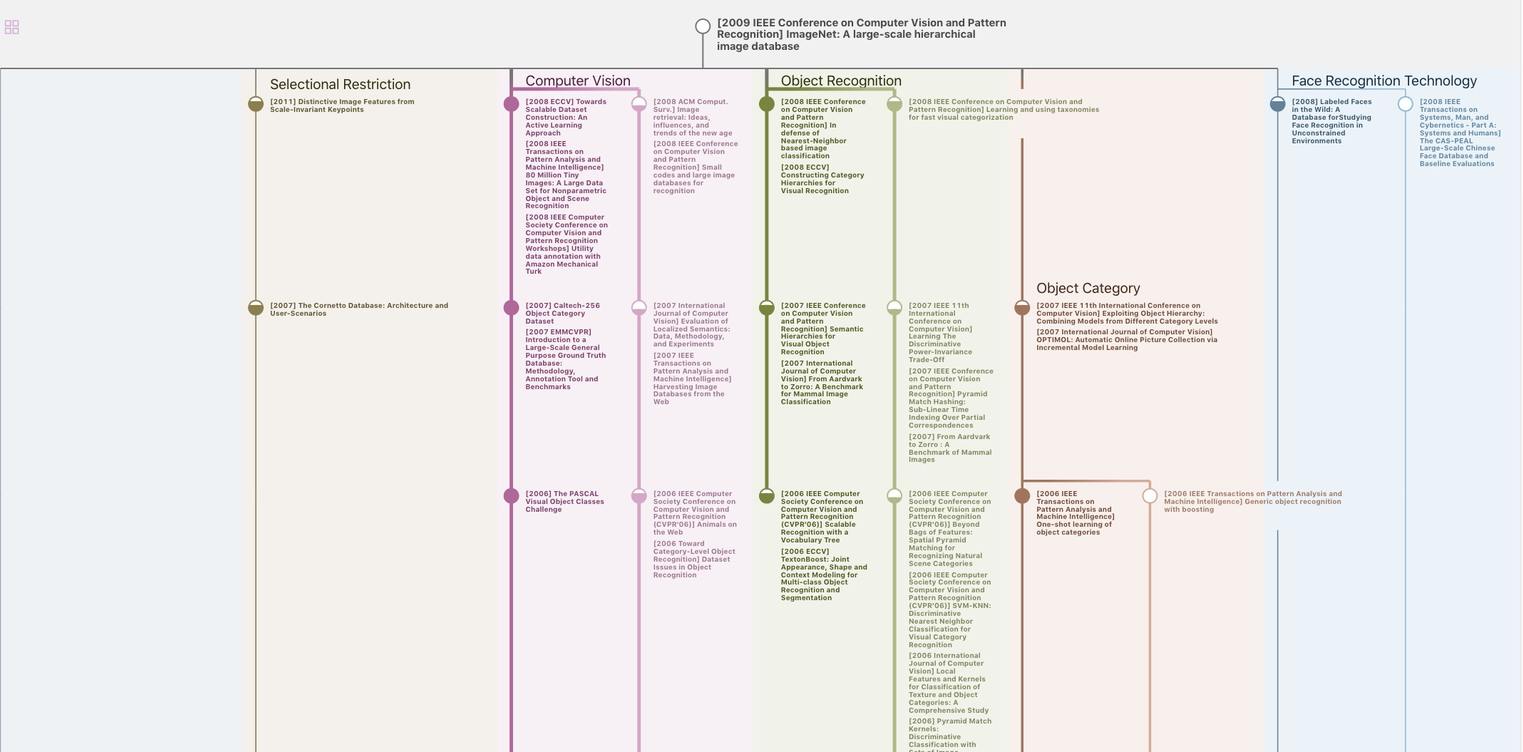
生成溯源树,研究论文发展脉络
Chat Paper
正在生成论文摘要