Deep Learning of Joint Scalar PDFs in Turbulent Flames from Sparse Multiscalar Data
COMBUSTION SCIENCE AND TECHNOLOGY(2023)
摘要
We investigate the reconstruction of multi-dimensional joint scalar probability density functions starting with sparse multiscalar data in turbulent flames using deep operator networks (DeepONets). The formulation is based on 1) a dimensionality reduction to reduce the complexity of modeling the PDF using principal component analysis (PCA), and 2) the implementation of the deep operator neural network (or DeepONet) to construct a generalizable joint PDF of the principal components (PCs). We demonstrate the accuracy and generalizability of the DeepONet on the Sandia turbulent non-premixed flames D, E and F where the DeepONet is trained on data for flame E and is evaluated for the 3 flames. The DeepONet-based approach is able to construct multiple PDF shapes based on the PCs for all conditions. Combined with a recently proposed method for evaluating thermochemical scalar means conditioned on the PCs, the convolution of these means with the joint PDFs yields good predictions of thermochemical scalars' unconditional means profiles in the flames.
更多查看译文
关键词
DeepONet,kernel density estimation,principal component analysis,joint scalar PDFs,turbulent combustion
AI 理解论文
溯源树
样例
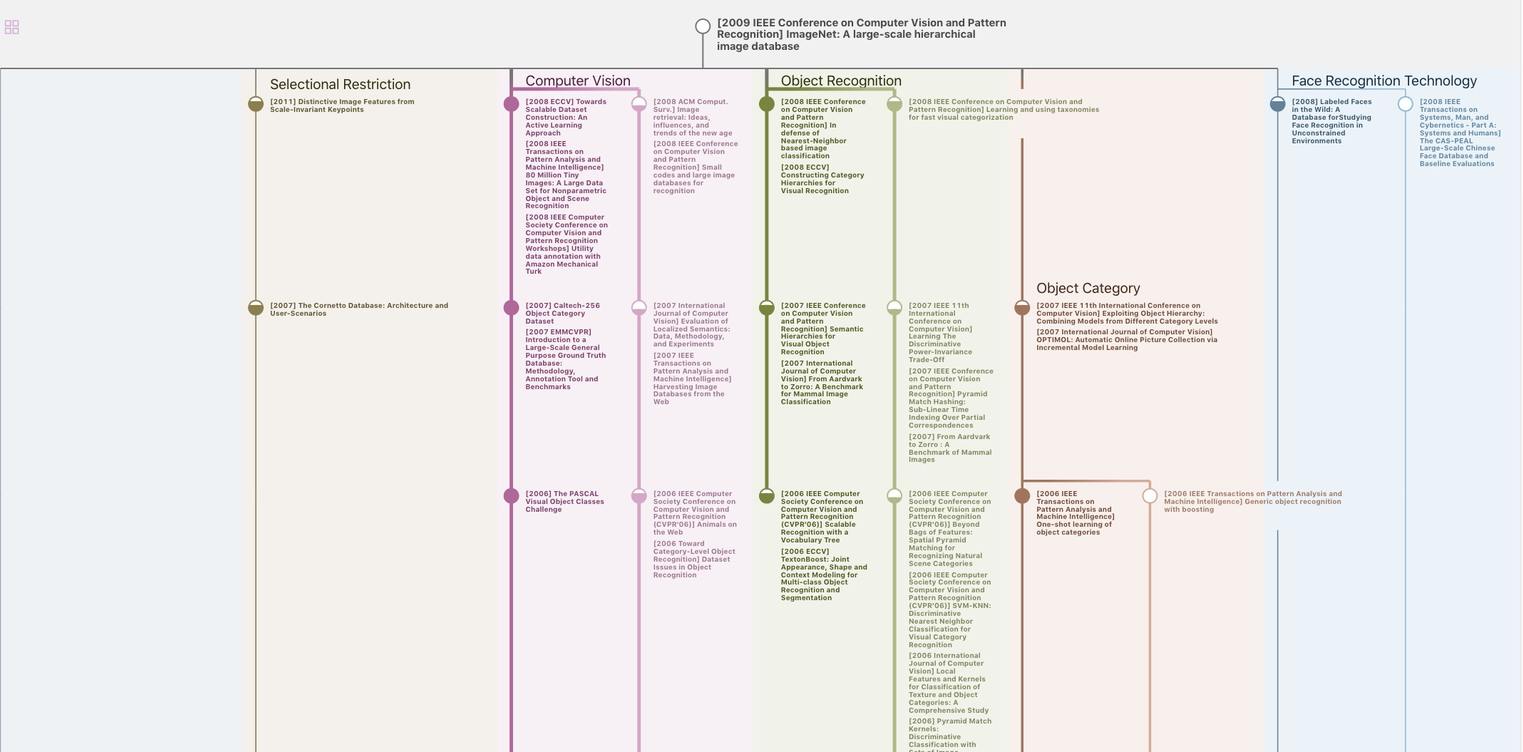
生成溯源树,研究论文发展脉络
Chat Paper
正在生成论文摘要