A feature filtering-based transfer network for few-shot soil organic carbon content estimation with spectral data
REMOTE SENSING LETTERS(2023)
摘要
At present, the deep learning method relies on its strong feature extraction ability and has been successfully applied to the estimation of soil organic carbon (SOC) content with large-scale hyperspectral data. However, in actual production, collecting and preparing soil samples is time-consuming and labour-intensive. This makes it difficult to train a well-behaved estimating model. To solve this problem, a novel few-shot learning (FSL) method is proposed in this letter. Specifically, the idea of domain adaptation and inductive transfer learning are integrated into a unified framework. Firstly, an unsupervised domain adaptive strategy is used to narrow the feature distribution of the source domain and target domain. Then, a network with two convolutional layers and a soft-threshold feature filtering (SFF) module is constructed, which aims to eliminate redundant features and reduce computational complexity. Wherein, the parameters of convolutional layers are migrated, and the SFF module uses the target domain to further fit. Furthermore, a modified mean square error (MMSE) loss function is used to deal with the problem of unbalanced sample labels in the target domain. Experiments on two small soil datasets show that this method outperforms the compared state-of-the-art methods and the coefficient of determination (R-2) reaches 0.448 and 0.809 when only using a few samples for AfSIS and SOC150, respectively.
更多查看译文
关键词
Estimation,few-shot learning,feature filtering,transfer learning,domain adaptation,hyperspectral data
AI 理解论文
溯源树
样例
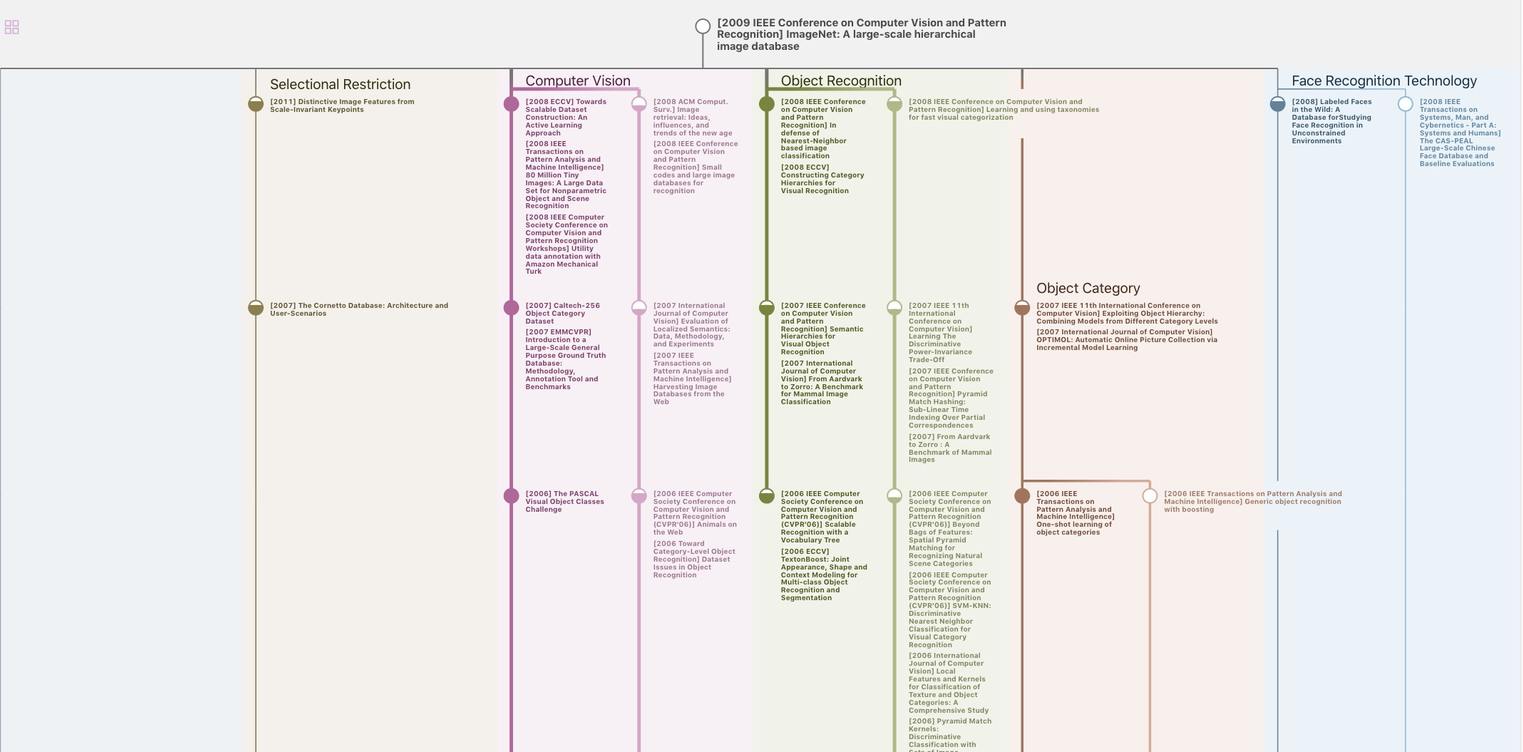
生成溯源树,研究论文发展脉络
Chat Paper
正在生成论文摘要