Spatially-correlated time series clustering using location-dependent Dirichlet process mixture model
STATISTICAL ANALYSIS AND DATA MINING(2024)
摘要
The Dirichlet process mixture (DPM) model has been widely used as a Bayesian nonparametric model for clustering. However, the exchangeability assumption of the Dirichlet process is not valid for clustering spatially correlated time series as these data are indexed spatially and temporally. While analyzing spatially correlated time series, correlations between observations at proximal times and locations must be appropriately considered. In this study, we propose a location-dependent DPM model by extending the traditional DPM model for clustering spatially correlated time series. We model the temporal pattern as an infinite mixture of Gaussian processes while considering spatial dependency using a location-dependent Dirichlet process prior over mixture components. This encourages the assignment of observations from proximal locations to the same cluster. By contrast, because mixture atoms for modeling temporal patterns are shared across space, observations with similar temporal patterns can be still grouped together even if they are located far apart. The proposed model also allows the number of clusters to be automatically determined in the clustering procedure. We validate the proposed model using simulated examples. Moreover, in a real case study, we cluster adjacent roads based on their traffic speed patterns that have changed as a result of a traffic accident occurred in Seoul, South Korea.
更多查看译文
关键词
Gaussian process,location-dependent Dirichlet process,mixture model,spatiotemporal clustering,spatiotemporal data,traffic speed patterns
AI 理解论文
溯源树
样例
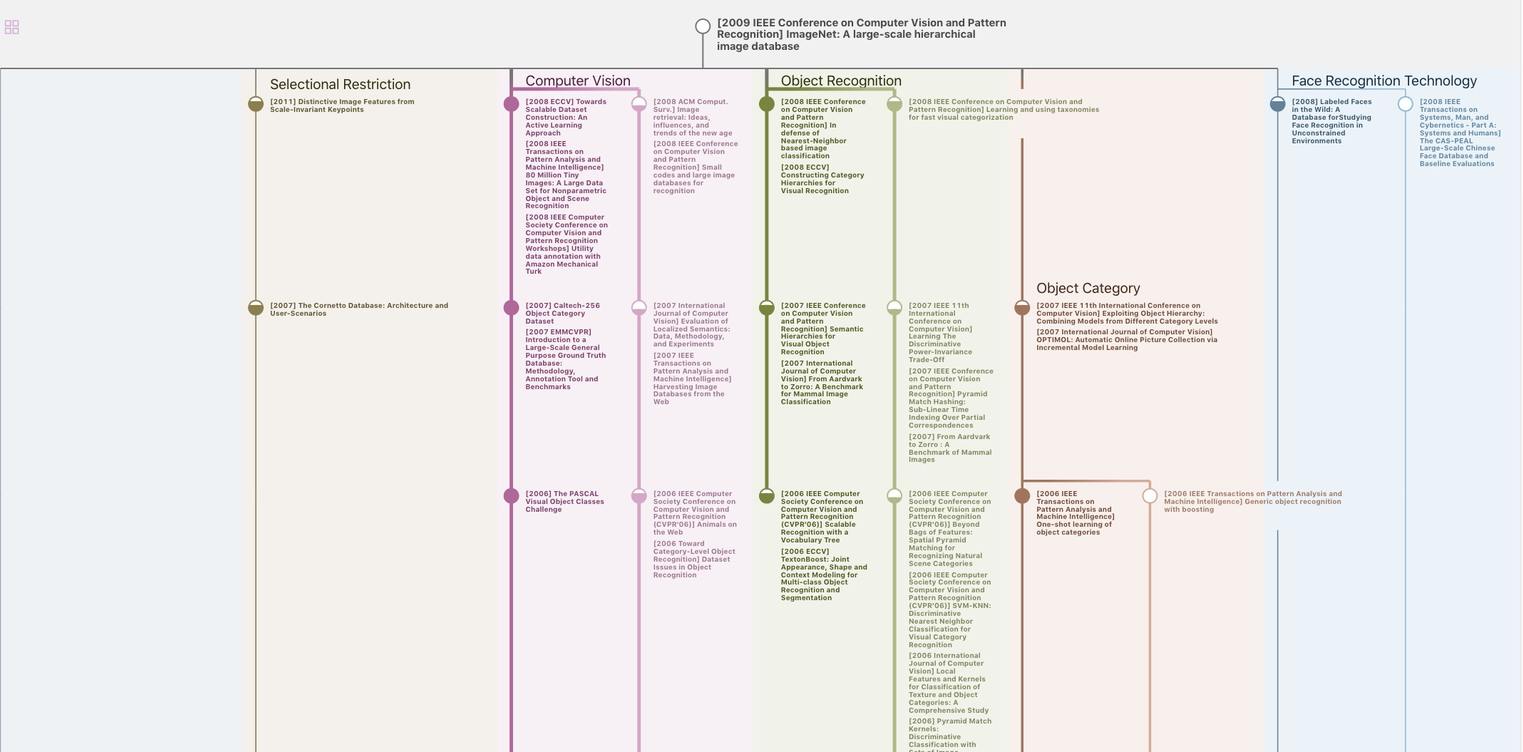
生成溯源树,研究论文发展脉络
Chat Paper
正在生成论文摘要