Identifying Molecular Structure-Energy Level Quantitative Relationship of Thermally Activated Delayed Fluorescence Materials Using Machine Learning
JOURNAL OF PHYSICAL CHEMISTRY C(2023)
摘要
The energy levels of thermally activated delayed fluorescence (TADF) materials are critical parameters in material screen and device structure design to obtain high-performance organic light-emitting diodes (OLEDs). In this article, we use machine learning (ML) algorithms to map quantitative molecular structure-energy level relationships and quickly predict the experimental energy levels of TADF materials. The molecular descriptors are used as the variables, and the experimentally measured energy levels are used as the output. The ML models present considerable low error and high accuracy in prediction, especially in predicting the highest occupied molecular orbital (HOMO), and they also point out the key factors determining the energy levels from a molecular viewpoint. Based on the optimized model, a series of TADF materials are enumerated and the corresponding HOMO energy levels are predicted and correlated with their specific molecular structures. As the dataset for ML used here is obtained from the experimental reports, the models and the results obtained in this work are referable for material design with desired energy levels.
更多查看译文
AI 理解论文
溯源树
样例
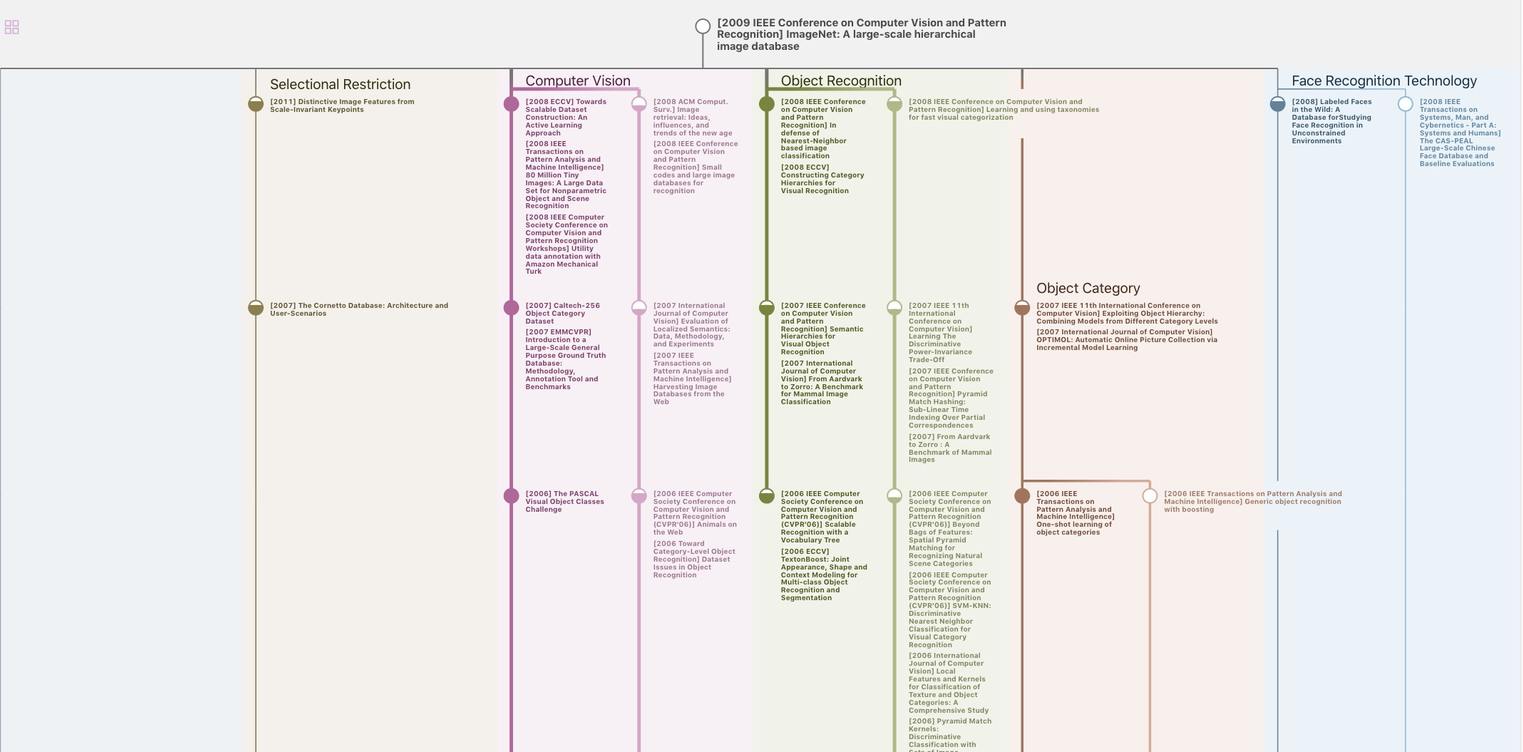
生成溯源树,研究论文发展脉络
Chat Paper
正在生成论文摘要