Utilization of deep learning-based metamodel for probabilistic seismic damage analysis of railway bridges considering the geometric variation
EARTHQUAKES AND STRUCTURES(2023)
摘要
A probabilistic seismic damage analysis is an essential procedure to identify seismically vulnerable structures, prioritize the seismic retrofit, and ultimately minimize the overall seismic risk. To assess the seismic risk of multiple structures within a region, a large number of nonlinear time-history structural analyses must be conducted and studied. As a result, each assessment requires high computing resources. To overcome this limitation, we explore a deep learning-based metamodel to enable the prediction of the mean and the standard deviation of the seismic damage distribution of track-on steel-plate girder railway bridges in Korea considering the geometric variation. For machine learning training, nonlinear dynamic time-history analyses are performed to generate 800 high-fidelity datasets on the seismic response. Through intensive trial and error, the study is concentrated on developing an optimal machine learning architecture with the pre-identified variables of the physical configuration of the bridge. Additionally, the prediction performance of the proposed method is compared with a previous, well-defined, response surface model. Finally, the statistical testing results indicate that the overall performance of the deep-learning model is improved compared to the response surface model, as its errors are reduced by as much as 61%. In conclusion, the model proposed in this study can be effectively deployed for the seismic fragility and risk assessment of a region with a large number of structures.
更多查看译文
关键词
deep learning,geometric variation,metamodel,railway bridge,response surface,seismic risk
AI 理解论文
溯源树
样例
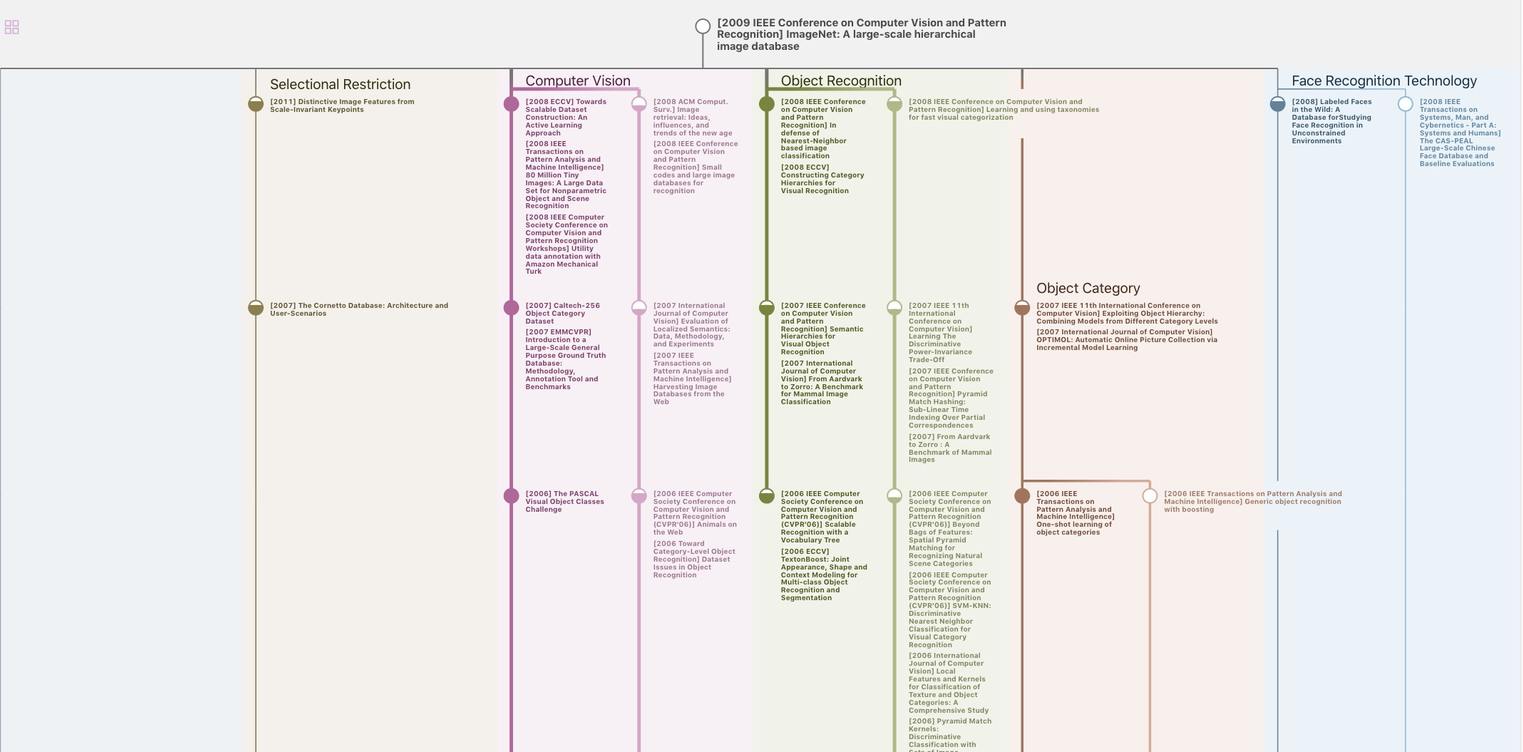
生成溯源树,研究论文发展脉络
Chat Paper
正在生成论文摘要