Resource-Aware Federated Hybrid Profiling for Edge Node Selection in Federated Patient Similarity Network
APPLIED SCIENCES-BASEL(2023)
摘要
The widespread adoption of edge computing for resource-constrained devices presents challenges in computational straggler issues, primarily due to the heterogeneity of edge node resources. This research addresses these issues by introducing a novel resource-aware federated hybrid profiling approach. This approach involves classifying edge node resources with relevant performance metrics and leveraging their capabilities to optimize performance and improve Quality of Service (QoS), particularly in real-time eHealth applications. Such paradigms include Federated Patient Similarity Network (FPSN) models that distribute processing at each edge node and fuse the built PSN matrices in the cloud, presenting a unique challenge in terms of optimizing training and inference times, while ensuring efficient and timely updates at the edge nodes. To address this concern, we propose a resource-aware federated hybrid profiling approach that measures the available static and dynamic resources of the edge nodes. By selecting nodes with the appropriate resources, we aim to optimize the FPSN to ensure the highest possible Quality of Service (QoS) for its users. We conducted experiments using edge performance metrics, i.e., accuracy, training convergence, memory and disk usage, execution time, and network statistics. These experiments uniquely demonstrate our work's contribution to optimizing resource allocation and enhancing the performance of eHealth applications in real-time contexts using edge computing.
更多查看译文
关键词
federated learning,federated resource profiling,deep learning,edge computing,workload profile,PSN,FPSN,eHealth
AI 理解论文
溯源树
样例
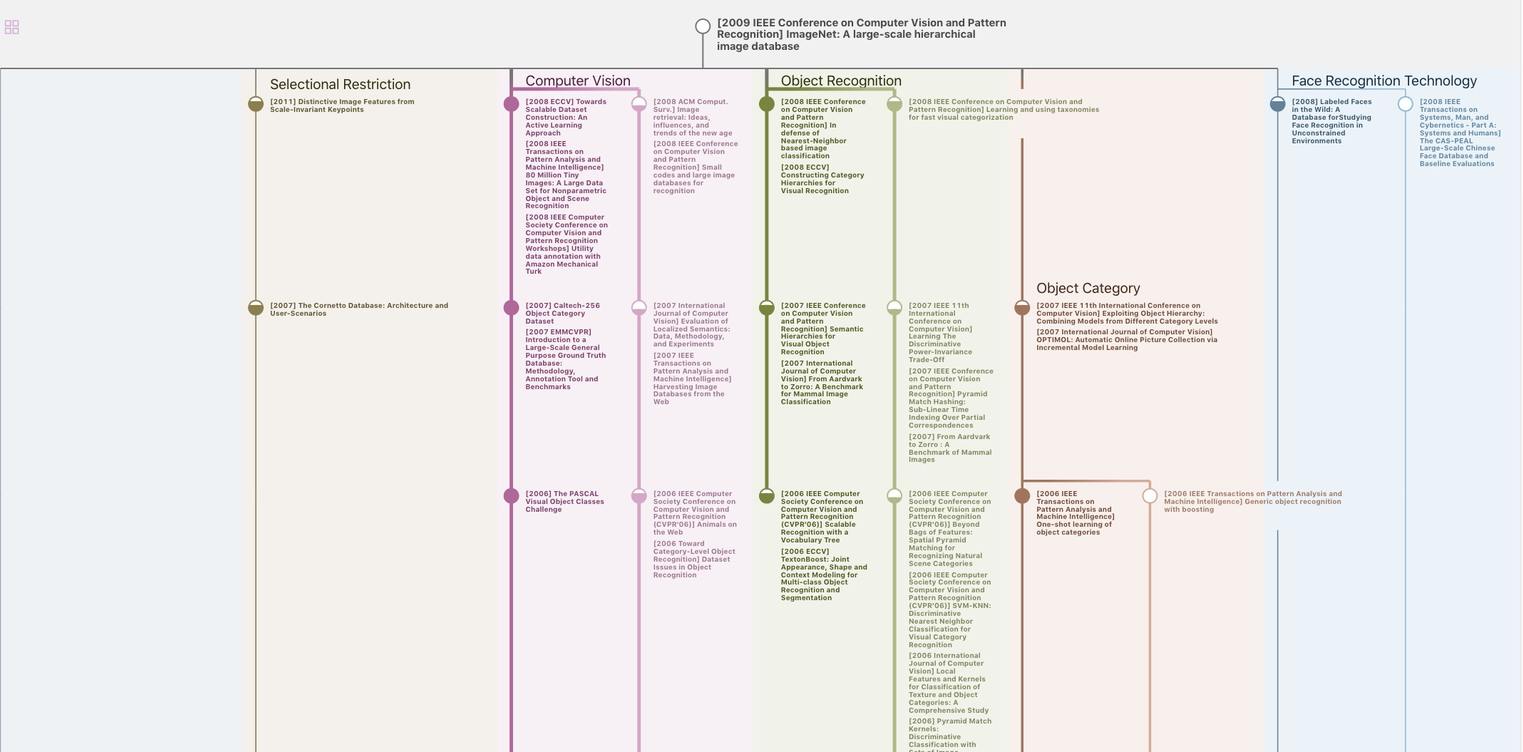
生成溯源树,研究论文发展脉络
Chat Paper
正在生成论文摘要