Local descriptors-based machine learning model refined by cluster analysis for accurately predicting adsorption energies on bimetallic alloys
JOURNAL OF MATERIALS CHEMISTRY A(2024)
摘要
Exploring the vast chemical compound space to provide activity-site relationships on bimetallic catalysts presents significant challenges. It also raises the necessity of developing methodologies capable of overcoming the cost of the computational screening of high-performing heterogeneous catalysts. In the present contribution, we introduce machine learning models enhanced by local descriptors related to the adsorption site for predicting adsorption energies. Additionally, we combined them with cluster analysis to bring valuable tools to detect anomalies in the database, thus enhancing the accuracy and robustness of the predictive models. This approach accurately predicts the adsorption energies of several species containing C, N, S, O, and atomic H adsorbed on AB-type bimetallic alloys with stoichiometric variation of A : B ratios. Among all the evaluated ML-based architectures, the CatBoost model exhibits the best performance with a MAE of 0.019 eV and 0.174 eV for the training and test sets, respectively. The cluster analysis highlights the importance of constructing descriptors containing physicochemical-intuitive insight for describing the bonding interactions. This methodology facilitates the recognition of electronic-structural trends of the surrounding local active site, thereby becoming a potential tool to screen adsorption energies and, ultimately, the catalytic activity. The CatBoost method, combined with cluster filtering, accurately predicts adsorption energies on metal alloys. The approach uses local chemical descriptors to understand chemisorption on metal alloys, which is essential for catalytic applications.
更多查看译文
AI 理解论文
溯源树
样例
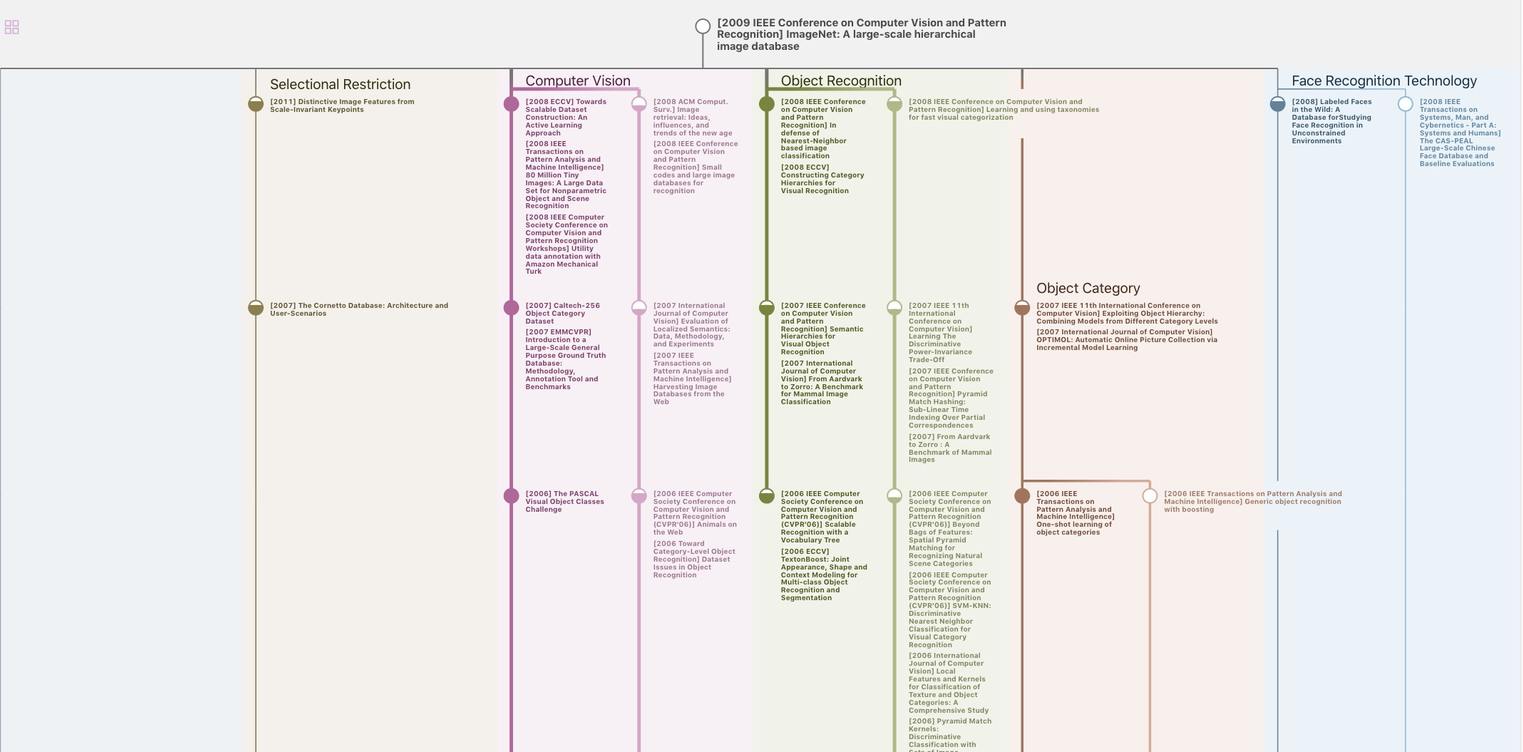
生成溯源树,研究论文发展脉络
Chat Paper
正在生成论文摘要