Histogram-Based Gradient Boosting Tree: A Federated Learning Approach for Collaborative Fault Diagnosis
IEEE-ASME TRANSACTIONS ON MECHATRONICS(2023)
摘要
Data-driven approaches have been widely applied in fault diagnosis. However, having sufficient labels to effectively develop fault diagnosis models in real-world manufacturing scenarios has remained a challenge. The key issue is to break down data silos and expand the source of data availability while safeguarding data privacy. To address abovementioned gap, a federated learning model using histogram-based gradient boosting tree, is proposed for collaborative fault diagnosis under privacy-preserving. During model training process, only an update of relevant local model parameters is communicated, thus protecting data privacy while contributing to the increased accuracy of the global model. Meanwhile, a data compensation mechanism is introduced to alleviate the disparity problem in data volume across participants. Two case studies are carried out and further compared with traditional situations to reveal the effectiveness of the proposed method. It is envisioned that this developed method can be useful for industrial practitioners to integrate their data for model training while maintaining data privacy, and promoting collaboration in digital manufacturing.
更多查看译文
关键词
Data privacy,fault diagnosis,federated learning (FL),predictive maintenance,rotating machines
AI 理解论文
溯源树
样例
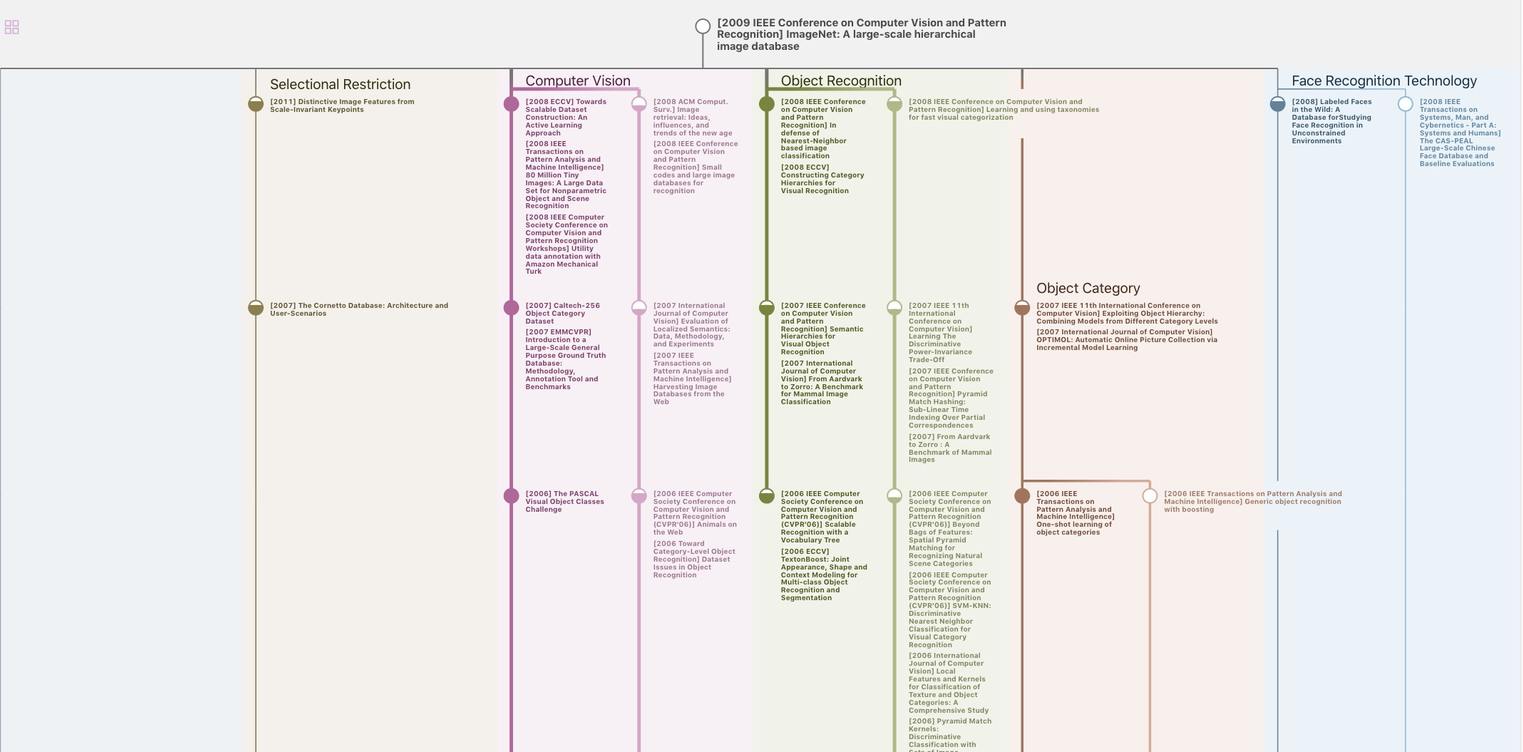
生成溯源树,研究论文发展脉络
Chat Paper
正在生成论文摘要