Generalized memory polynomial input neural networks for nonlinear modeling in digital predistortion applications
INTERNATIONAL JOURNAL OF NUMERICAL MODELLING-ELECTRONIC NETWORKS DEVICES AND FIELDS(2024)
摘要
The power amplifiers (PAs) will embody the nonlinear distortion if they work at saturation so as to increase efficiency. The neural network (NN)-based digital predistortion (DPD) is widely applied for the linearization of the PA in wireless communication. However, the current NN-based DPD schemes only focus on designing the NN hidden layer and ignore improving the NN input layer, which loses the degree of freedom to fit the DPD. Therefore, this paper first proposes the generalized memory polynomial (GMP) input NN structure. The training data of GMP-NN outputs are obtained by iterative learning control. The experimental results show that the GMP-NN presents a great advantage in performance compared with the widely used GMP model and recurrent NN (RNN) model. From the testing data, it is observed that the minimum mean square error (MMSE) of the GMP-NN is 4 dB lower than the conventional GMP model and is nearly 8 dB lower than the RNN model. The proposed GMP-NN model is also verified in the DPD application, which shows 2.61 dB improvement in comparison with the GMP DPD model.
更多查看译文
关键词
digital predistortion (DPD),generalized memory polynomial (GMP),nonlinear distortion
AI 理解论文
溯源树
样例
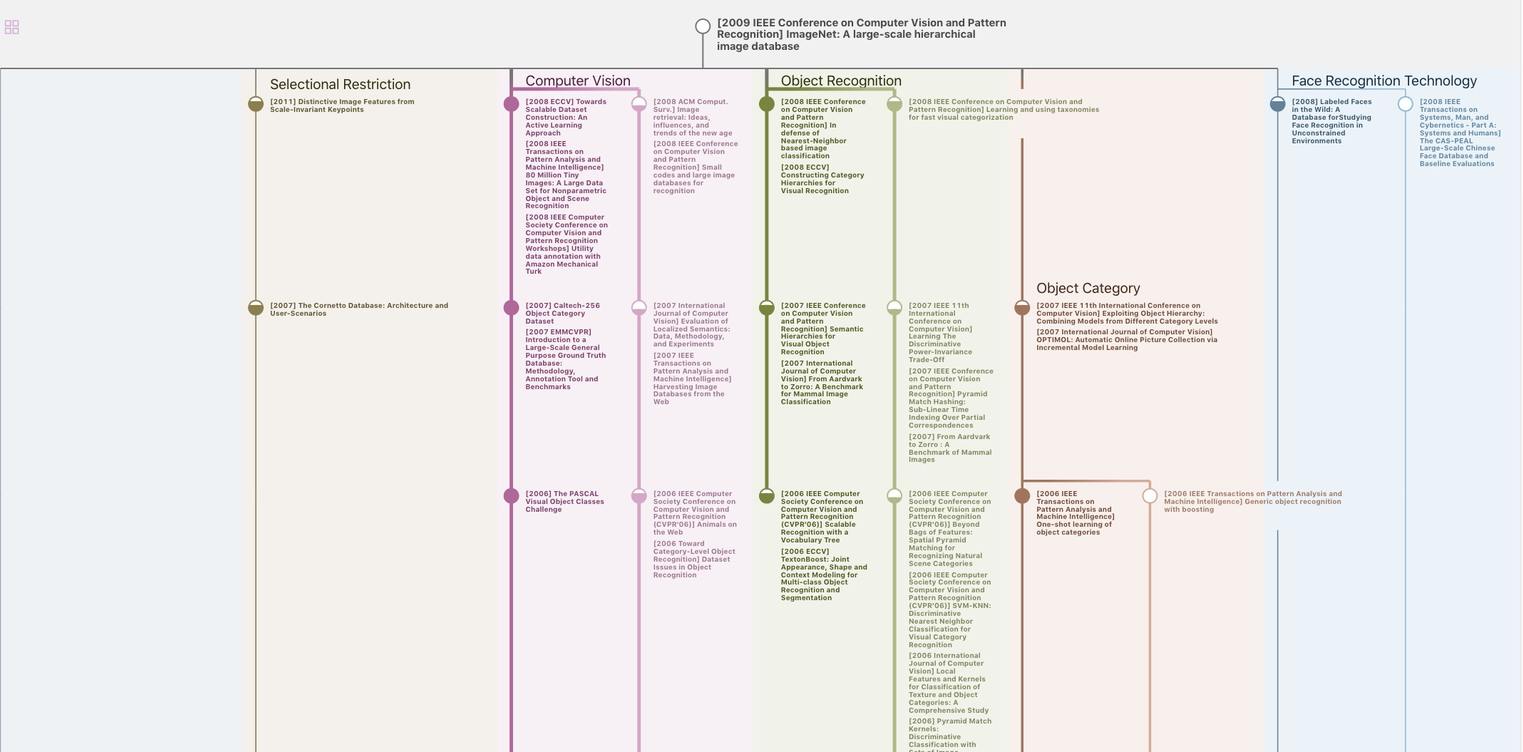
生成溯源树,研究论文发展脉络
Chat Paper
正在生成论文摘要