Emotional State Prediction Based on EEG Signals using Ensemble Methods
INTERNATIONAL JOURNAL OF ADVANCED COMPUTER SCIENCE AND APPLICATIONS(2023)
摘要
emotional state is an essential factor that affects mental health. Electroencephalography (EEG) signal analysis is a promising method for detecting emotional states. Although multiple studies exist on EEG emotional signals classification, they have rarely considered processing time as a metric for classification model evaluation. Instead, they used either model accuracy and/or the number of features for evaluation. Processing time is an important factor to be considered in the context of mental health. Many people commonly use smart devices, such as smartwatches to monitor their emotional state and such devices require a short processing time. This research proposes an EEG-based model that detects emotional signals based on three factors: accuracy, number of features, and processing time. Two feature extraction algorithms were applied to EEG emotional signals: principal components analysis (PCA) and fast independent components analysis (FastICA). In the classification process, ensemble method classifiers were adopted due to their powerful performance. Three ensemble classifiers were used: random forest (RF), extreme gradient boosting (XGBoost), and adaptive boost (AdaBoost). The experimental results showed that the RF and XGBoost achieved the best accuracy, i.e., 95%, for both methods. However, XGBoost outperformed RF in terms of the number of features; it used 33 components extracted by PCA within 14 seconds, while RF used 36 within 4 seconds. AdaBoost was the worst in terms of both accuracy and processing time in the two experiments.
更多查看译文
关键词
-Electroencephalograph,mental health,feature extraction,random forest,extreme gradient boosting,adaptive boost
AI 理解论文
溯源树
样例
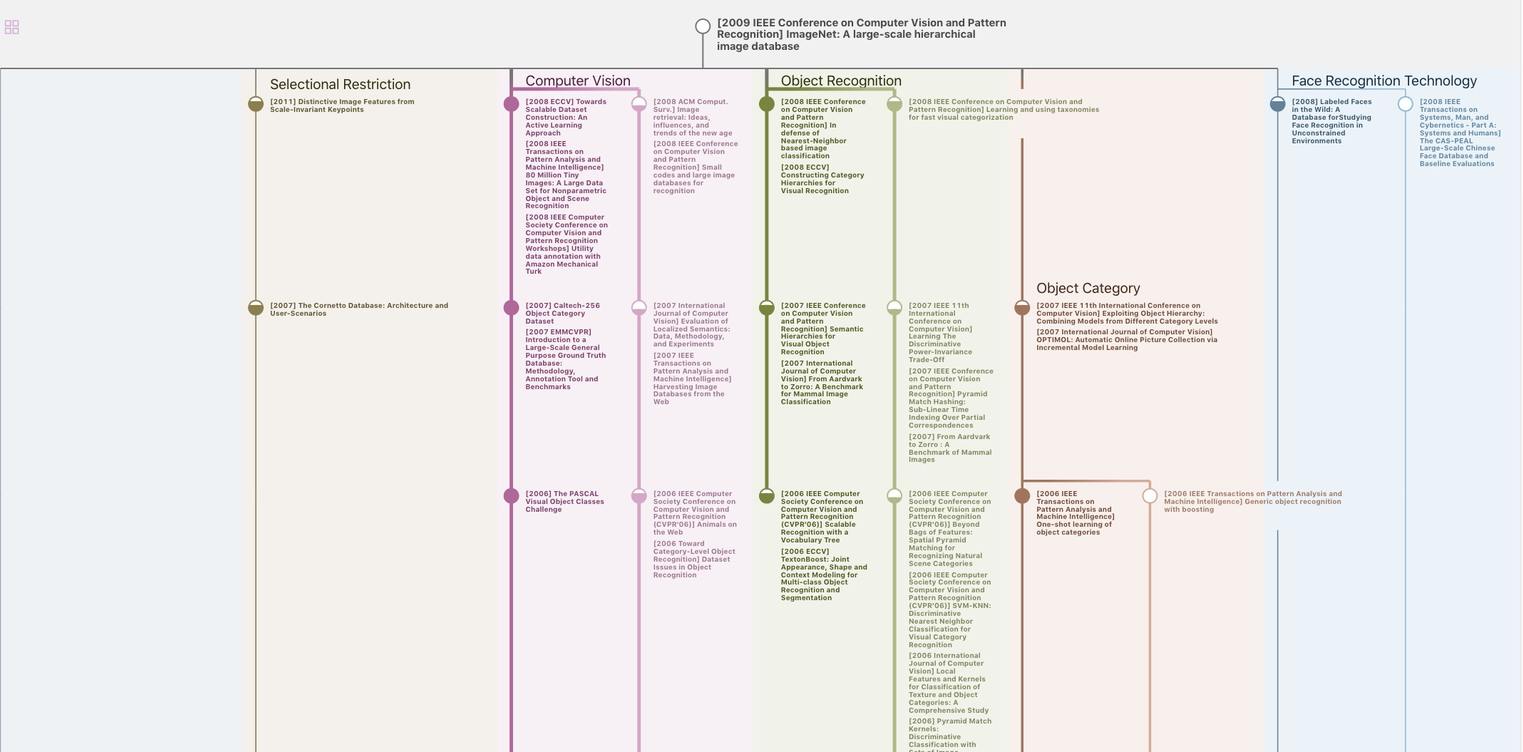
生成溯源树,研究论文发展脉络
Chat Paper
正在生成论文摘要