A Case-Study Comparison of Machine Learning Approaches for Predicting Student's Dropout from Multiple Online Educational Entities
ALGORITHMS(2023)
摘要
Predicting student dropout is a crucial task in online education. Traditionally, each educational entity (institution, university, faculty, department, etc.) creates and uses its own prediction model starting from its own data. However, that approach is not always feasible or advisable and may depend on the availability of data, local infrastructure, and resources. In those cases, there are various machine learning approaches for sharing data and/or models between educational entities, using a classical centralized machine learning approach or other more advanced approaches such as transfer learning or federated learning. In this paper, we used data from three different LMS Moodle servers representing homogeneous different-sized educational entities. We tested the performance of the different machine learning approaches for the problem of predicting student dropout with multiple educational entities involved. We used a deep learning algorithm as a predictive classifier method. Our preliminary findings provide useful information on the benefits and drawbacks of each approach, as well as suggestions for enhancing performance when there are multiple institutions. In our case, repurposed transfer learning, stacked transfer learning, and centralized approaches produced similar or better results than the locally trained models for most of the entities.
更多查看译文
关键词
dropout prediction,predictive analytics,transfer learning,federated learning
AI 理解论文
溯源树
样例
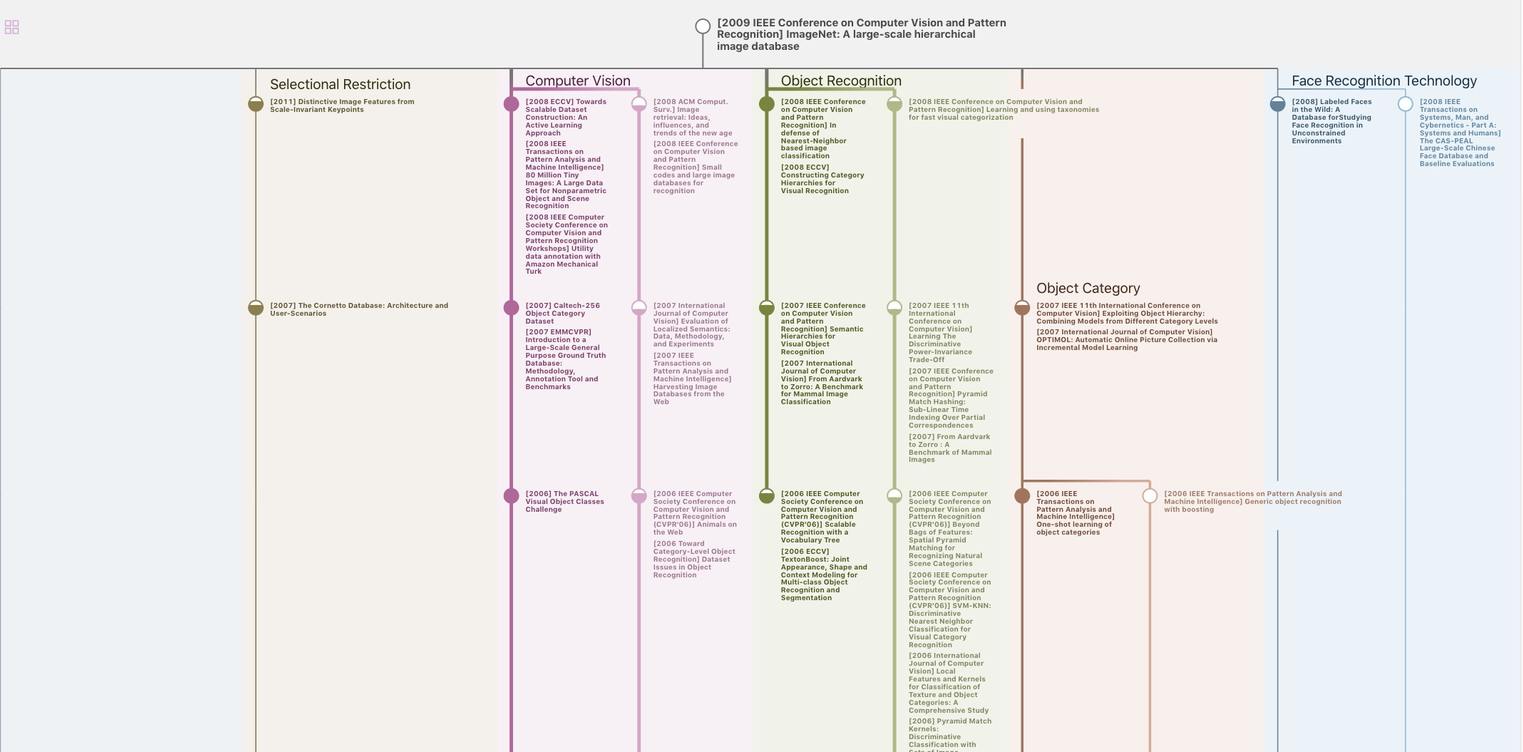
生成溯源树,研究论文发展脉络
Chat Paper
正在生成论文摘要