Semantic Knowledge Integrated Graph Convolutional Network for Zero-Sample Tracing of Control Performance Degradation
INDUSTRIAL & ENGINEERING CHEMISTRY RESEARCH(2023)
摘要
Tracing the cause of control performance degradation (CPD) as early as possible is important to reducing the loss and risks for industrial processes. The existing tracing algorithms for CPD generally require massive amounts of data for model training. However, in practical industries, many CPD types may not be supported by historical data, which are denoted as unseen CPD types here but require online identification. This common but challenging scenario is termed the zero-sample tracing of CPD. In this work, a semantic knowledge integrated graph convolutional network is proposed to solve this problem. While data corresponding to some CPD types are not available during the training phase, the CPD types themselves are known to the users. We describe all of the CPD types in both training and testing sets by a series of attributes, including degradation locations, characteristics, causal reasons, and so forth, which contain semantic knowledge. Unlike existing methods that directly predict class labels of CPD types, we predict the semantic attributes of the unseen CPD types to further infer the class labels. In this way, semantic attributes act as a bridge connecting degraded data and labels, which can transfer the knowledge of seen CPD types to unseen ones to realize the zero-sample tracing of CPD. Besides, the sparse graph convolutional network is designed to remove redundant information while concurrently analyzing the correlated and temporal relationships hidden in the loop data. The practical utility and feasibility of the proposed method are verified with the Tennessee-Eastman process.
更多查看译文
AI 理解论文
溯源树
样例
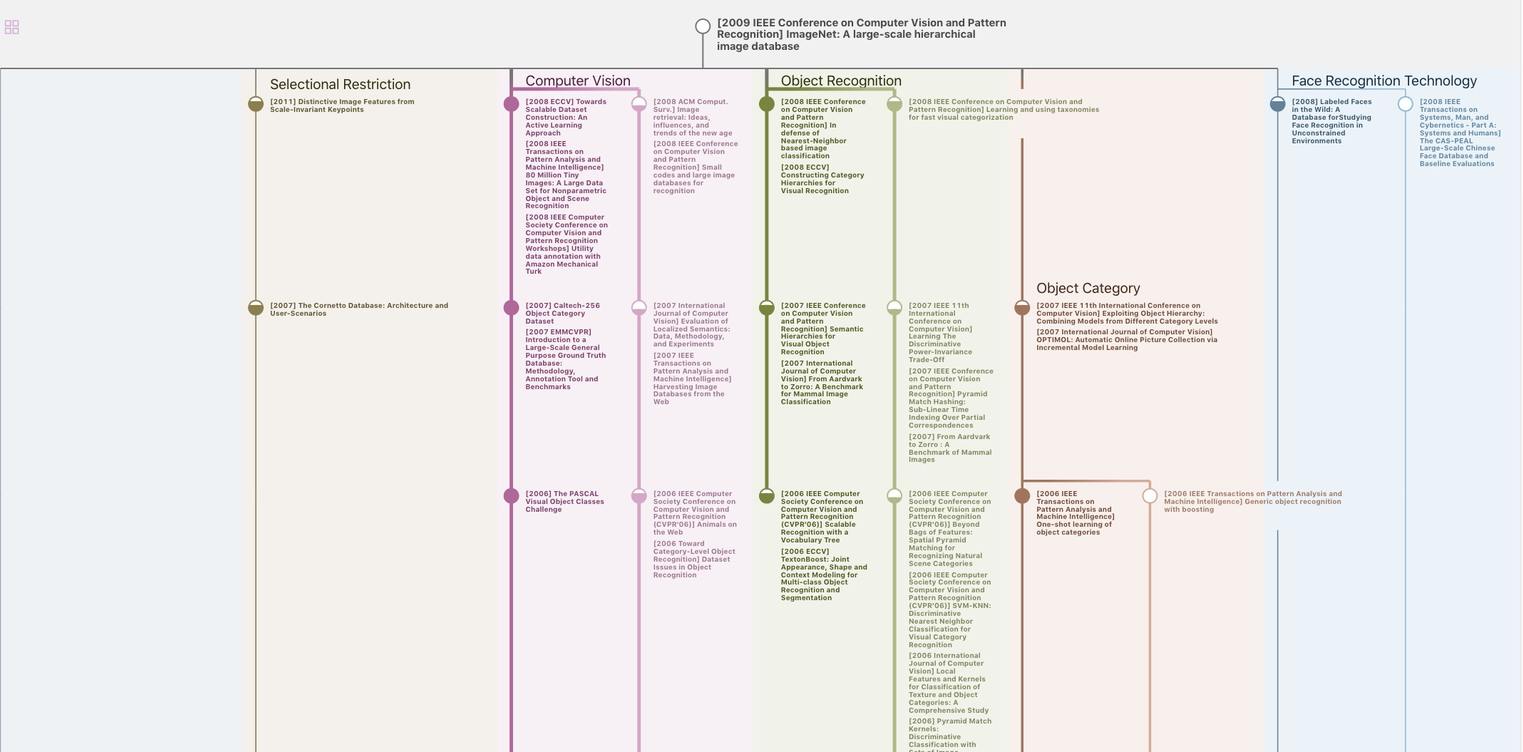
生成溯源树,研究论文发展脉络
Chat Paper
正在生成论文摘要