Dual-Supervised Contrastive Learning for Bundle Recommendation
IEEE TRANSACTIONS ON COMPUTATIONAL SOCIAL SYSTEMS(2023)
摘要
Self-supervised contrastive learning has well advanced the development of bundle recommendation. However, self-supervised contrastive learning may misclassify some positive samples as negative samples, resulting in suboptimal models. Therefore, the industry has proposed supervised contrastive learning to alleviate this drawback. Inspired by this idea, we seek a more elegant contrastive learning paradigm in the field of recommendation, so we propose a dual-supervised contrastive learning for bundle recommendation (DSCBR), which integrates supervised and self-supervised contrastive learning to exploit the full potential of contrastive learning. Specifically, we first construct self-supervised contrastive learning between the two different views (bundle view and item view), which encourages the alignment of the two separately learned views and boosts the effectiveness of learned representations. Second, we use the interaction information to construct supervised contrastive learning and leverage the bundle-bundle cooccurrence graph for further enhancement. By introducing supervised contrastive learning, our model explicitly models user and bundle proximity in different views, improving the model's robustness and generalization. Finally, we jointly perform self-supervised and supervised contrastive learning across multiple views. Extensive experiments on three public datasets demonstrate the effectiveness of our model.
更多查看译文
关键词
Bundle recommendation,self-supervised contrastive learning,supervised contrastive learning
AI 理解论文
溯源树
样例
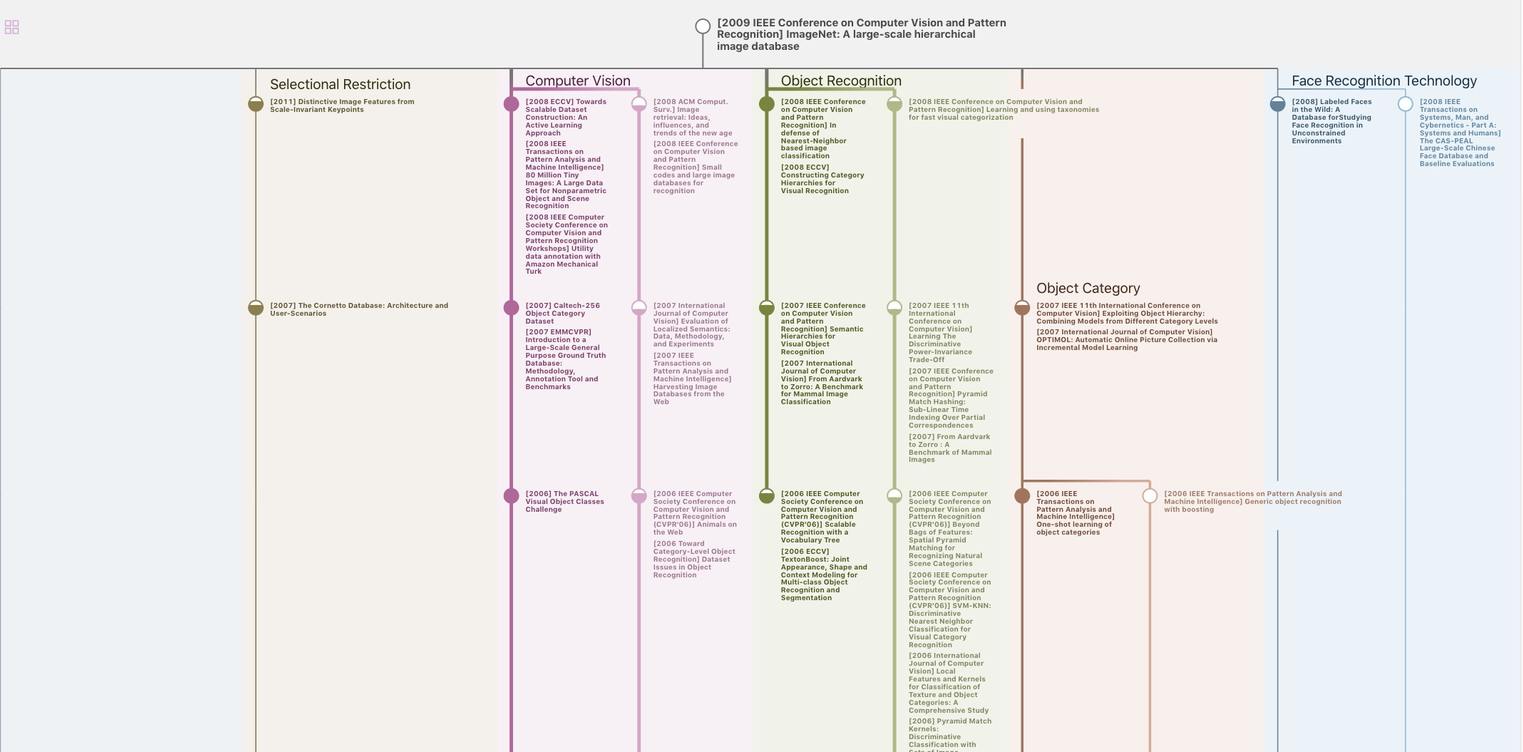
生成溯源树,研究论文发展脉络
Chat Paper
正在生成论文摘要