Accommodating Misclassification Effects on Optimizing Dynamic Treatment Regimes with Q-learning
STATISTICS IN MEDICINE(2024)
Abstract
Research on dynamic treatment regimes has enticed extensive interest. Many methods have been proposed in the literature, which, however, are vulnerable to the presence of misclassification in covariates. In particular, although Q-learning has received considerable attention, its applicability to data with misclassified covariates is unclear. In this article, we investigate how ignoring misclassification in binary covariates can impact the determination of optimal decision rules in randomized treatment settings, and demonstrate its deleterious effects on Q-learning through empirical studies. We present two correction methods to address misclassification effects on Q-learning. Numerical studies reveal that misclassification in covariates induces non-negligible estimation bias and that the correction methods successfully ameliorate bias in parameter estimation.
MoreTranslated text
Key words
dynamic treatment regimes,estimating function,misclassification,Q-learning,regression calibration,regression models
AI Read Science
Must-Reading Tree
Example
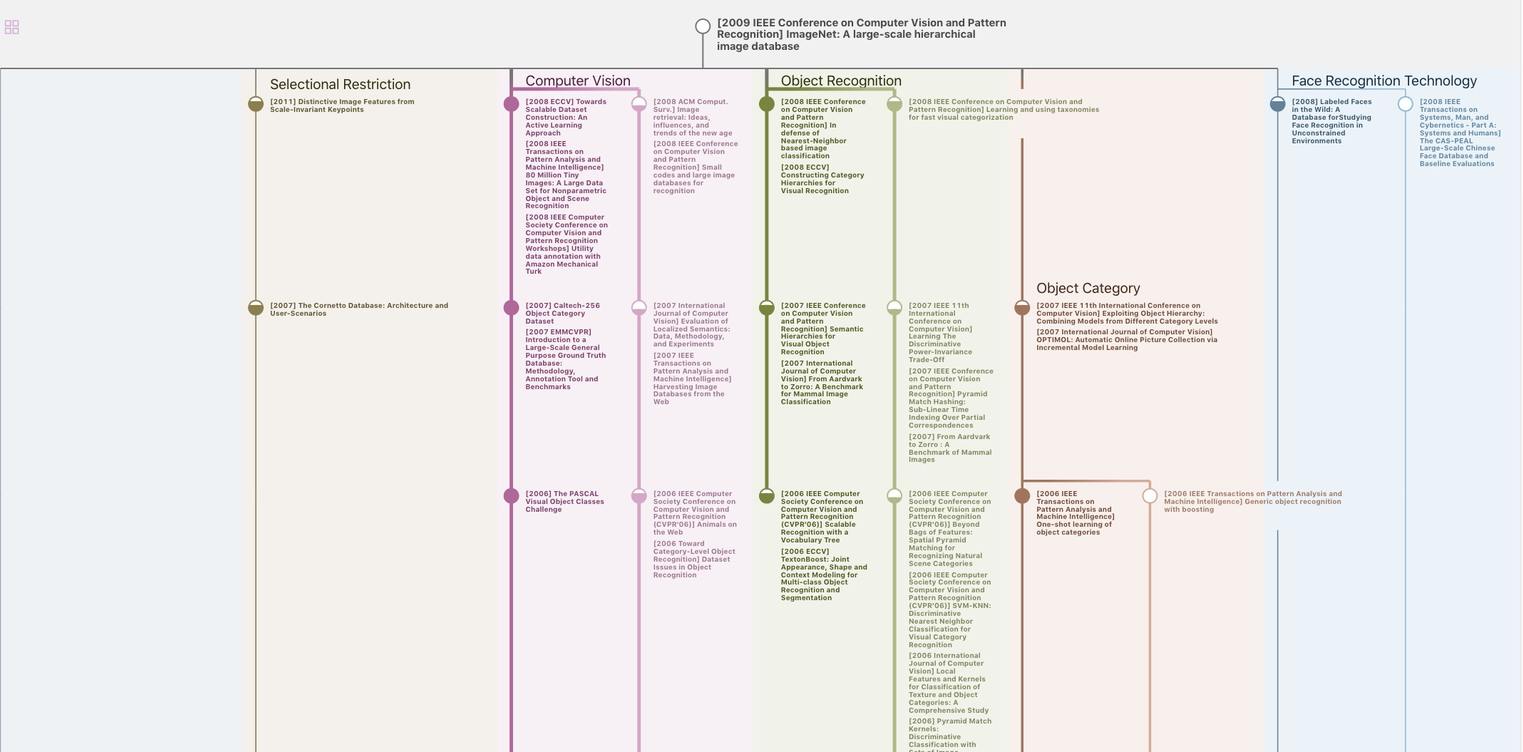
Generate MRT to find the research sequence of this paper
Chat Paper
Summary is being generated by the instructions you defined