Biometric systems for identification and verification scenarios using spatial footsteps components
Neural Computing and Applications(2024)
摘要
Humans are distinguished by their walking patterns; many approaches, including using various types of sensors, have been used to establish walking patterns as biometrics. By studying the distinguishing features of a person's footsteps, footstep recognition may be utilized in numerous security applications, such as managing access in protected locations or giving an additional layer of biometric verification for secure admittance into restricted regions. We proposed biometric systems for verifying and identifying a person by acquiring spatial foot pressure images from the values obtained from the piezoelectric sensors using the Swansea Foot Biometric Database, which contains 19,980 footstep signals from 127 users and is the most prominent open-source gait database available for footstep recognition. The images acquired are fed into the ConvNeXt model, which was trained using the transfer learning technique, using 16 stride footstep signals in each batch with an Adam optimizer and a learning rate of 0.0001, and using sparse categorical cross-entropy as the loss function. The proposed ConvNeXt model has been adjusted to acquire 512 feature vectors per image, and these feature vectors are used to train the logistic regression models. We propose two biometric systems. The first biometric system is based on training one logistic regression model as a classifier to identify 40 different users using 1600 signals for training, 6697 signals for validation, and 200 signals for evaluation. The second biometric system is based on training 40 logistic regression models, one for each user, to validate the user's authenticity, with a total number of 2363 training signals, 7077 validation signals, and 500 evaluation signals. Each of the 40 models has a 40-training signal per client and a different number of signals per imposter, a different number of signals for the validation that ranges between 8 and 650 signals, a 5-signal for an authenticated client, and a different number of signals per imposter for evaluation. Independent validation and evaluation sets are used to evaluate our systems. In the biometric identification system, we obtained an equal error rate of 15.30% and 21.72% for the validation and evaluation sets, while in the biometric verification system, we obtained an equal error rate of 6.97% and 10.25% for the validation and evaluation sets.
更多查看译文
关键词
Biometrics,Logistic regression,ConvNeXt network,Transfer learning,Footsteps recognition
AI 理解论文
溯源树
样例
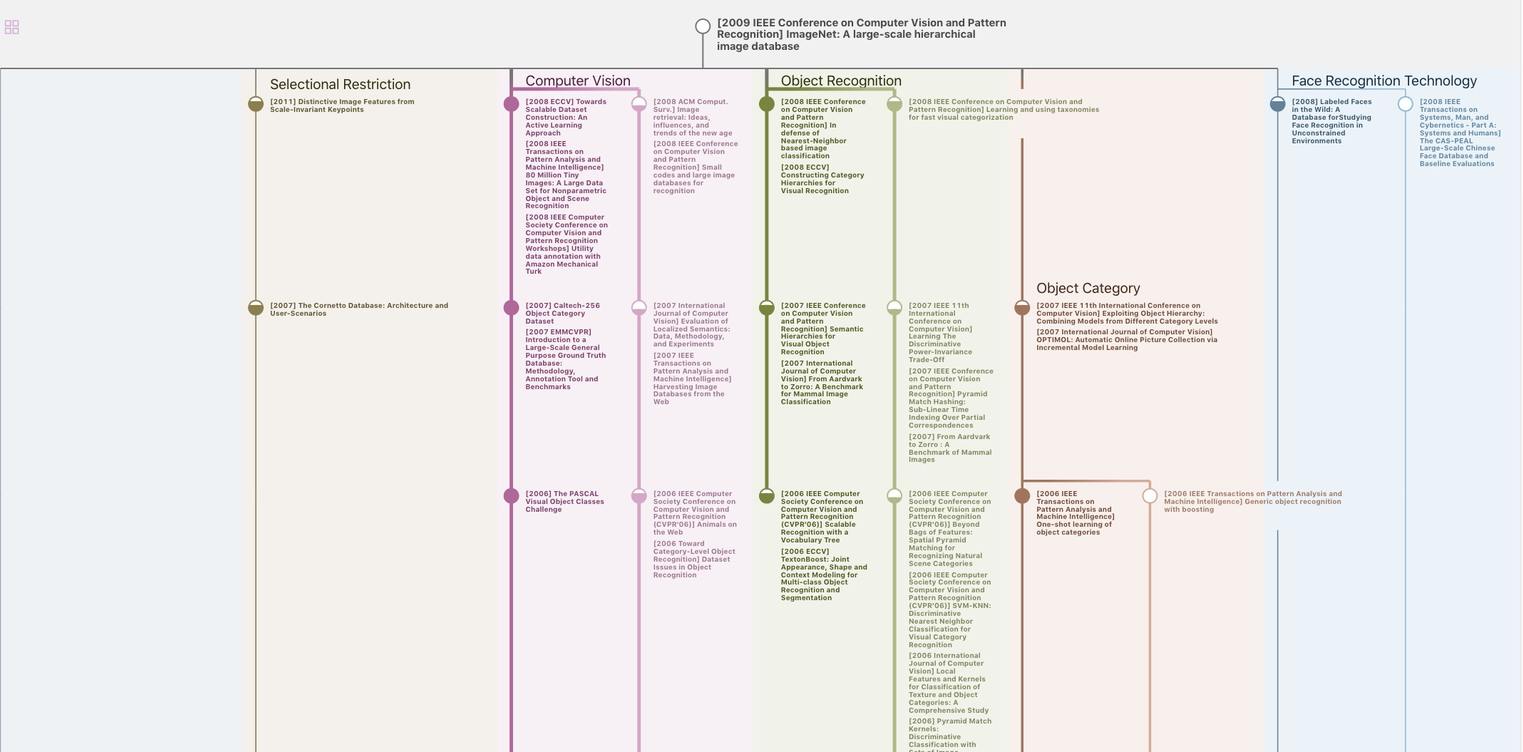
生成溯源树,研究论文发展脉络
Chat Paper
正在生成论文摘要