Artificial Intelligence-Based Autosegmentation: Advantages in Delineation, Absorbed Dose-Distribution, and Logistics
ADVANCES IN RADIATION ONCOLOGY(2024)
摘要
Purpose: The study's purpose was to compare the performance of artificial intelligence (AI) in auto-contouring compared with a human practitioner in terms of precision, differences in dose distribution, and time consumption. Methods and Materials: Datasets of previously irradiated patients in 3 different segments (head and neck, breast, and prostate cancer) were retrospectively collected. An experienced radiation oncologist (MD) performed organs-at-risk (OARs) and standard clinical target volume delineations as baseline structures for comparison. AI-based autocontours were generated in 2 additional CT copies; therefore, 3 groups were assessed: MD alone, AI alone, and AI plus MD corrections (AI+C). Differences in Dice similarity coefficient (DSC) and person -hour burden were assessed. Furthermore, changes in clinically relevant dose-volume parameters were evaluated and compared. Results: Seventy-five previously treated cases were collected (25 per segment) for the analysis. Compared with MD contours, the mean DSC scores were higher than 0.7 for 74% and 80% of AI and AI+C, respectively. After corrections, 17.1% structures presented DSC score deviations higher than 0.1 and 10.4% dose-volume parameters significantly changed in AI-contoured structures. The time consumption assessment yielded mean person -hour reductions of 68%, 51%, and 71% for breast, prostate, and head and neck cancer, respectively. Conclusions: In great extent, AI yielded clinically acceptable OARs and certain clinical target volumes in the explored anatomic segments. Sparse correction and assessment requirements place AI+C as a standard workflow. Minimal clinically relevant differences in OAR exposure were identified. A substantial amount of person-hours could be repurposed with this technology. (c) 2023 The Author(s). Published by Elsevier Inc. on behalf of American Society for Radiation Oncology. This is an open access article under the CC BY-NC-ND license (http://creativecommons.org/licenses/by-nc-nd/4.0/).
更多查看译文
AI 理解论文
溯源树
样例
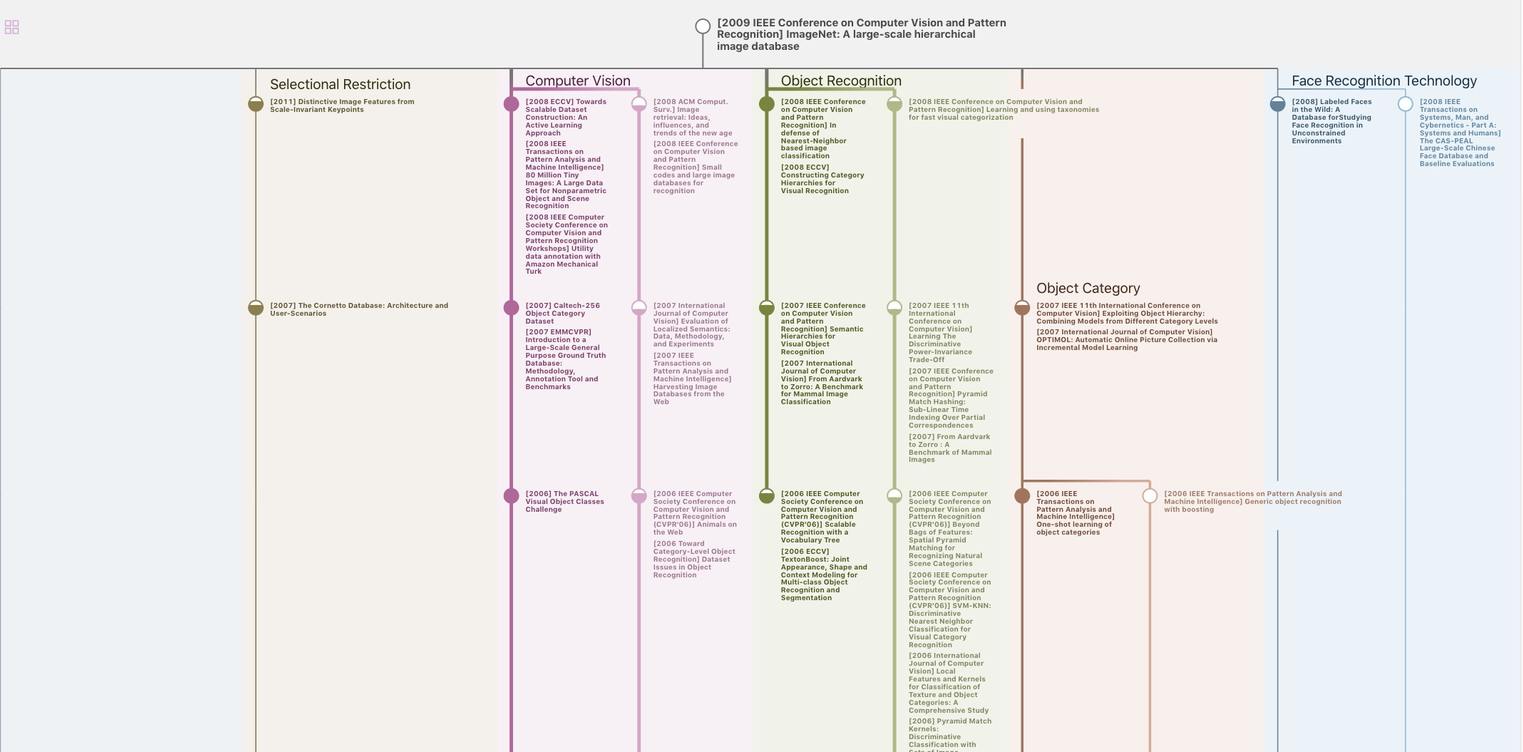
生成溯源树,研究论文发展脉络
Chat Paper
正在生成论文摘要