Towards Efficient Neural Decoder for Dexterous Finger Force Predictions.
IEEE transactions on bio-medical engineering(2024)
摘要
OBJECTIVE:Dexterous control of robot hands requires a robust neural-machine interface capable of accurately decoding multiple finger movements. Existing studies primarily focus on single-finger movement or rely heavily on multi-finger data for decoder training, which requires large datasets and high computation demand. In this study, we investigated the feasibility of using limited single-finger surface electromyogram (sEMG) data to train a neural decoder capable of predicting the forces of unseen multi-finger combinations.
METHODS:We developed a deep forest-based neural decoder to concurrently predict the extension and flexion forces of three fingers (index, middle, and ring-pinky). We trained the model using varying amounts of high-density EMG data in a limited condition (i.e., single-finger data).
RESULTS:We showed that the deep forest decoder could achieve consistently commendable performance with 7.0% of force prediction errors and R2 value of 0.874, significantly surpassing the conventional EMG amplitude method and convolutional neural network approach. However, the deep forest decoder accuracy degraded when a smaller amount of data was used for training and when the testing data became noisy.
CONCLUSION:The deep forest decoder shows accurate performance in multi-finger force prediction tasks. The efficiency aspect of the deep forest lies in the short training time and small volume of training data, which are two critical factors in current neural decoding applications.
SIGNIFICANCE:This study offers insights into efficient and accurate neural decoder training for advanced robotic hand control, which has the potential for real-life applications during human-machine interactions.
更多查看译文
关键词
Hand function,finger force prediction,deep forest,convolutional neural network
AI 理解论文
溯源树
样例
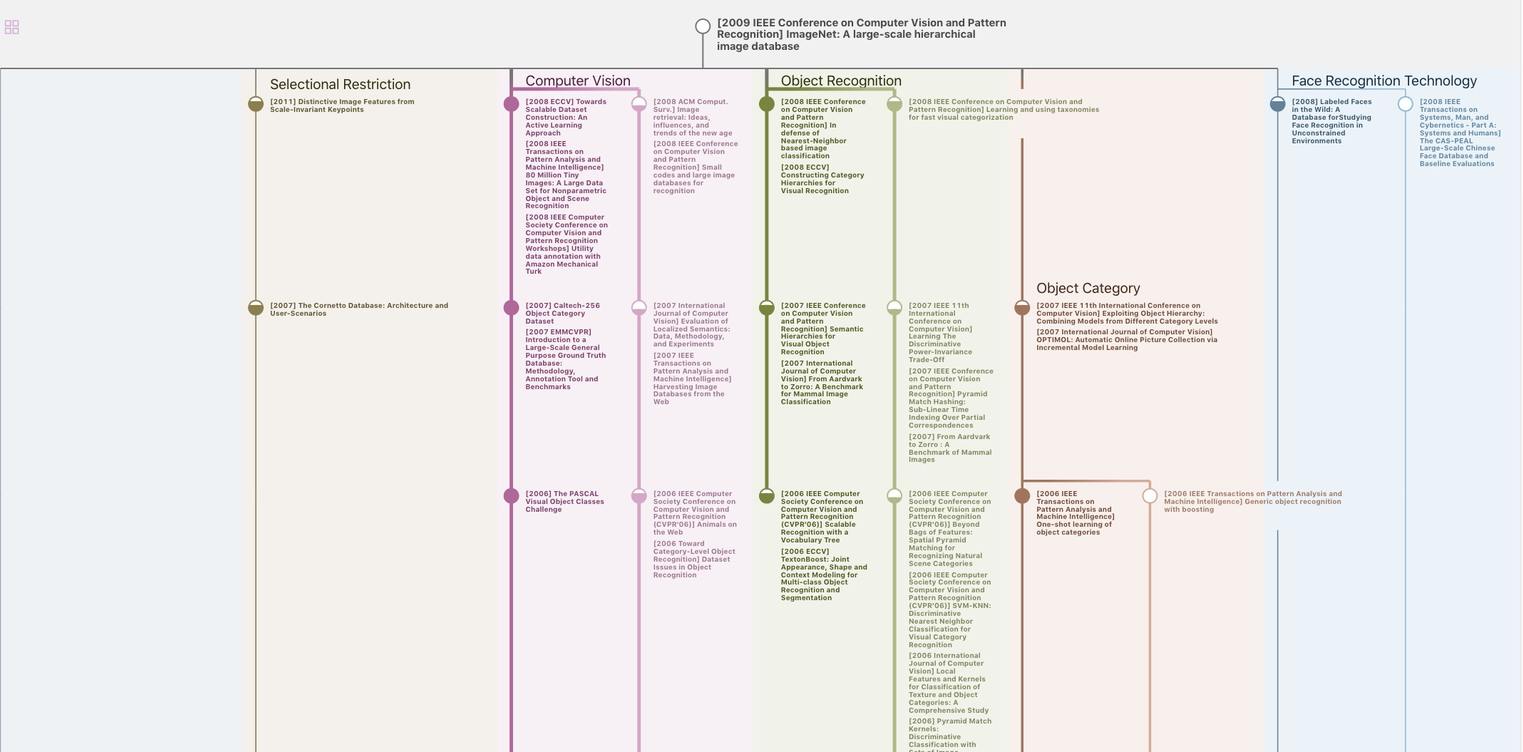
生成溯源树,研究论文发展脉络
Chat Paper
正在生成论文摘要