Shapelet-based orientation and defect identification method for nanostructured surface imaging
NANOTECHNOLOGY(2024)
摘要
Structure-property relations are of fundamental importance for continued progress in materials research. Determining these relationships for nanomaterials introduces additional challenges, especially when nanostructure is present, either through self-assembly or nano-lithographic processes. Recent advances have been made for quantification of nanostructured surfaces, for which many robust experimental imaging methods exist. One promising approach is based on the use of shapelet functions for image analysis, which may be used as a reduced basis for surface pattern structure resulting from a broad range of phenomena (e.g. self-assembly). These shapelet-based methods enable automated quantification of nanostructured images, guided by the user/researcher, providing pixel-level information of local order without requiring detailed knowledge of order symmetries. In this work, enhancements to the existing shapelet-based response distance method are developed which enable further analysis of local order, including quantification of local orientation and identification of topological defects. The presented shapelet-based methods are applied to a representative set of images of self-assembled surfaces from experimental characterization techniques including scanning electron microscopy, atomic force microscopy, and transmission electron microscopy. These methods are shown to be complementary in implementation and, importantly, provide researchers with a robust and generalized computational approach to comprehensively quantify nanostructure order, including local orientation and boundaries within well-aligned grains.
更多查看译文
关键词
nanostructure,surfaces,self-assembly,shapelets,image processing,machine learning
AI 理解论文
溯源树
样例
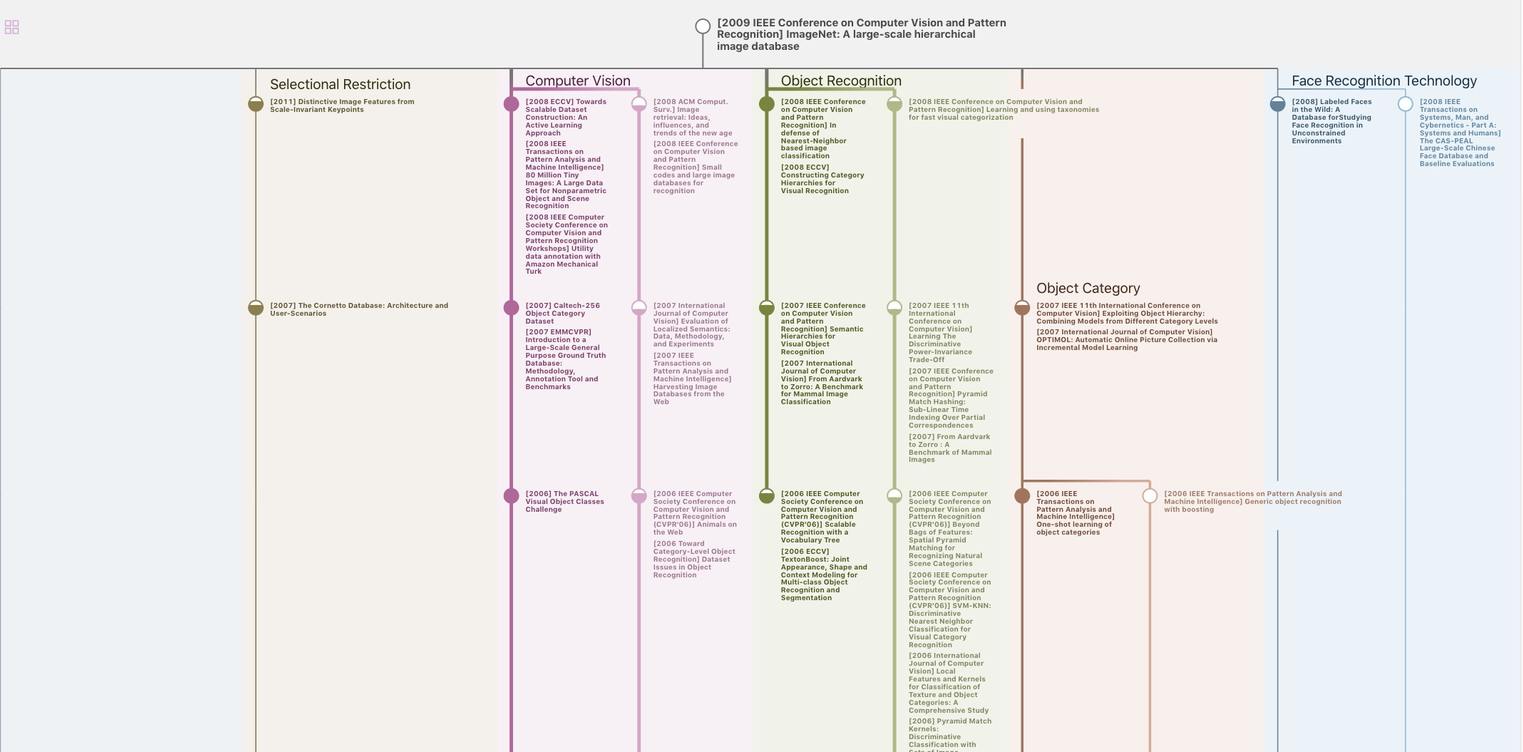
生成溯源树,研究论文发展脉络
Chat Paper
正在生成论文摘要