LCB-net: Long-Context Biasing for Audio-Visual Speech Recognition
CoRR(2024)
摘要
The growing prevalence of online conferences and courses presents a new challenge in improving automatic speech recognition (ASR) with enriched textual information from video slides. In contrast to rare phrase lists, the slides within videos are synchronized in real-time with the speech, enabling the extraction of long contextual bias. Therefore, we propose a novel long-context biasing network (LCB-net) for audio-visual speech recognition (AVSR) to leverage the long-context information available in videos effectively. Specifically, we adopt a bi-encoder architecture to simultaneously model audio and long-context biasing. Besides, we also propose a biasing prediction module that utilizes binary cross entropy (BCE) loss to explicitly determine biased phrases in the long-context biasing. Furthermore, we introduce a dynamic contextual phrases simulation to enhance the generalization and robustness of our LCB-net. Experiments on the SlideSpeech, a large-scale audio-visual corpus enriched with slides, reveal that our proposed LCB-net outperforms general ASR model by 9.4%/9.1%/10.9% relative WER/U-WER/B-WER reduction on test set, which enjoys high unbiased and biased performance. Moreover, we also evaluate our model on LibriSpeech corpus, leading to 23.8%/19.2%/35.4% relative WER/U-WER/B-WER reduction over the ASR model.
更多查看译文
关键词
Multi-Modal,Audio-Visual Speech Recognition,Biasing Prediction,Contextual Adaptation
AI 理解论文
溯源树
样例
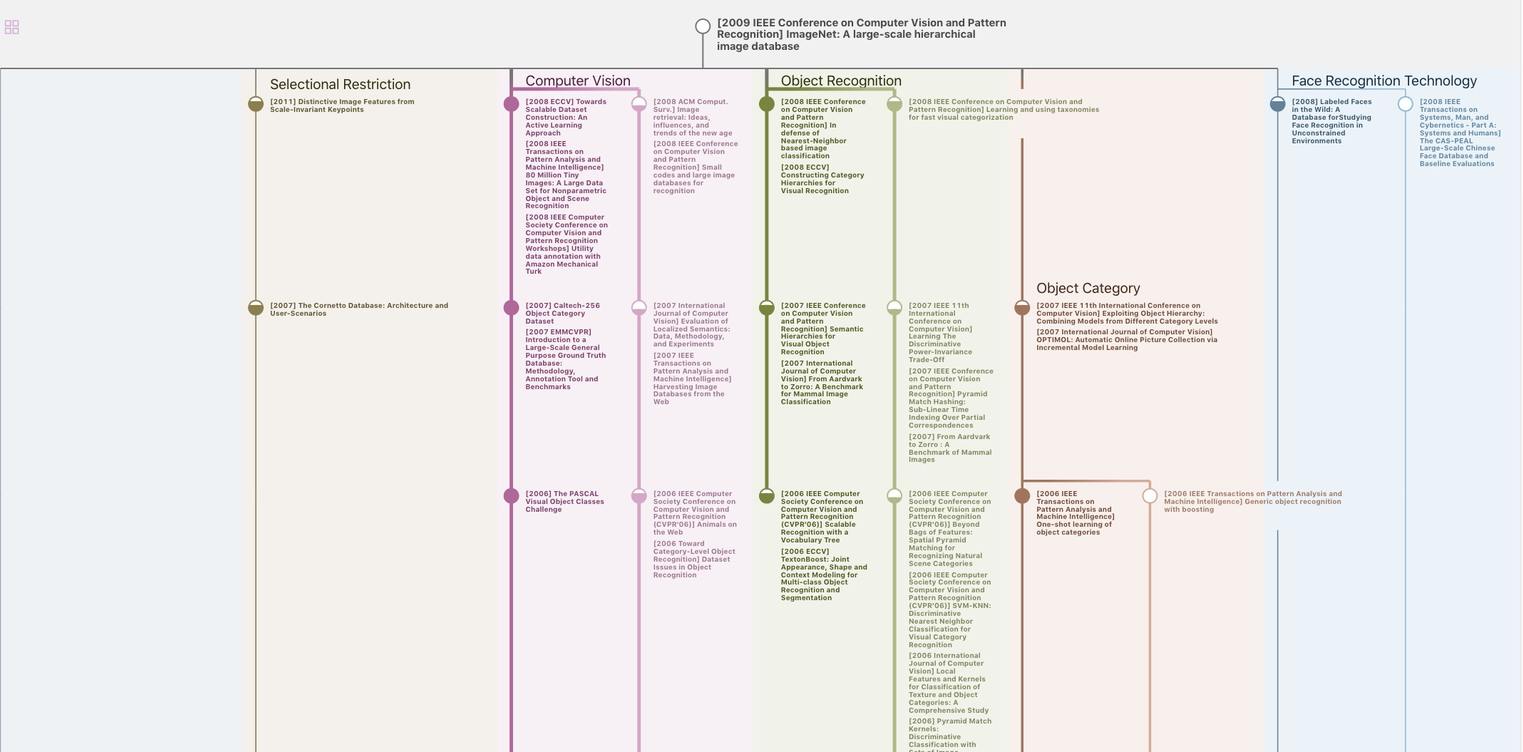
生成溯源树,研究论文发展脉络
Chat Paper
正在生成论文摘要